Diverse Ensembles Improve Calibration
arxiv(2020)
摘要
Modern deep neural networks can produce badly calibrated predictions, especially when train and test distributions are mismatched. Training an ensemble of models and averaging their predictions can help alleviate these issues. We propose a simple technique to improve calibration, using a different data augmentation for each ensemble member. We additionally use the idea of `mixing' un-augmented and augmented inputs to improve calibration when test and training distributions are the same. These simple techniques improve calibration and accuracy over strong baselines on the CIFAR10 and CIFAR100 benchmarks, and out-of-domain data from their corrupted versions.
更多查看译文
关键词
calibration
AI 理解论文
溯源树
样例
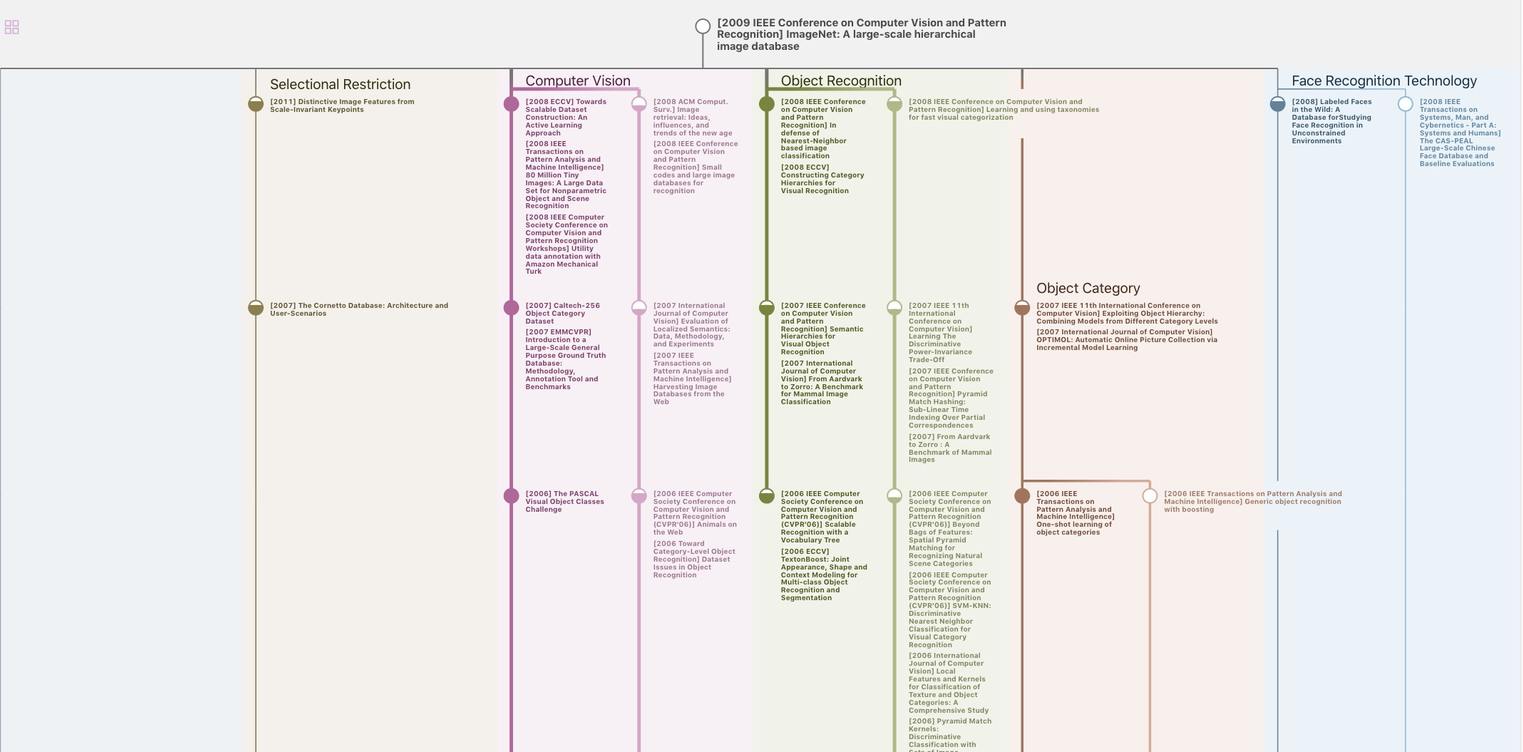
生成溯源树,研究论文发展脉络
Chat Paper
正在生成论文摘要