Convolutional Neural Network and Motor Current Signature Analysis during the Transient State for Detection of Broken Rotor Bars in Induction Motors.
SENSORS(2020)
摘要
Although induction motors (IMs) are robust and reliable electrical machines, they can suffer different faults due to usual operating conditions such as abrupt changes in the mechanical load, voltage, and current power quality problems, as well as due to extended operating conditions. In the literature, different faults have been investigated; however, the broken rotor bar has become one of the most studied faults since the IM can operate with apparent normality but the consequences can be catastrophic if the fault is not detected in low-severity stages. In this work, a methodology based on convolutional neural networks (CNNs) for automatic detection of broken rotor bars by considering different severity levels is proposed. To exploit the capabilities of CNNs to carry out automatic image classification, the short-time Fourier transform-based time-frequency plane and the motor current signature analysis (MCSA) approach for current signals in the transient state are first used. In the experimentation, four IM conditions were considered: half-broken rotor bar, one broken rotor bar, two broken rotor bars, and a healthy rotor. The results demonstrate the effectiveness of the proposal, achieving 100% of accuracy in the diagnosis task for all the study cases.
更多查看译文
关键词
broken rotor bars,convolutional neural network,current signals,induction motor,motor current signature analysis (MCSA),short-time Fourier transform,transient state
AI 理解论文
溯源树
样例
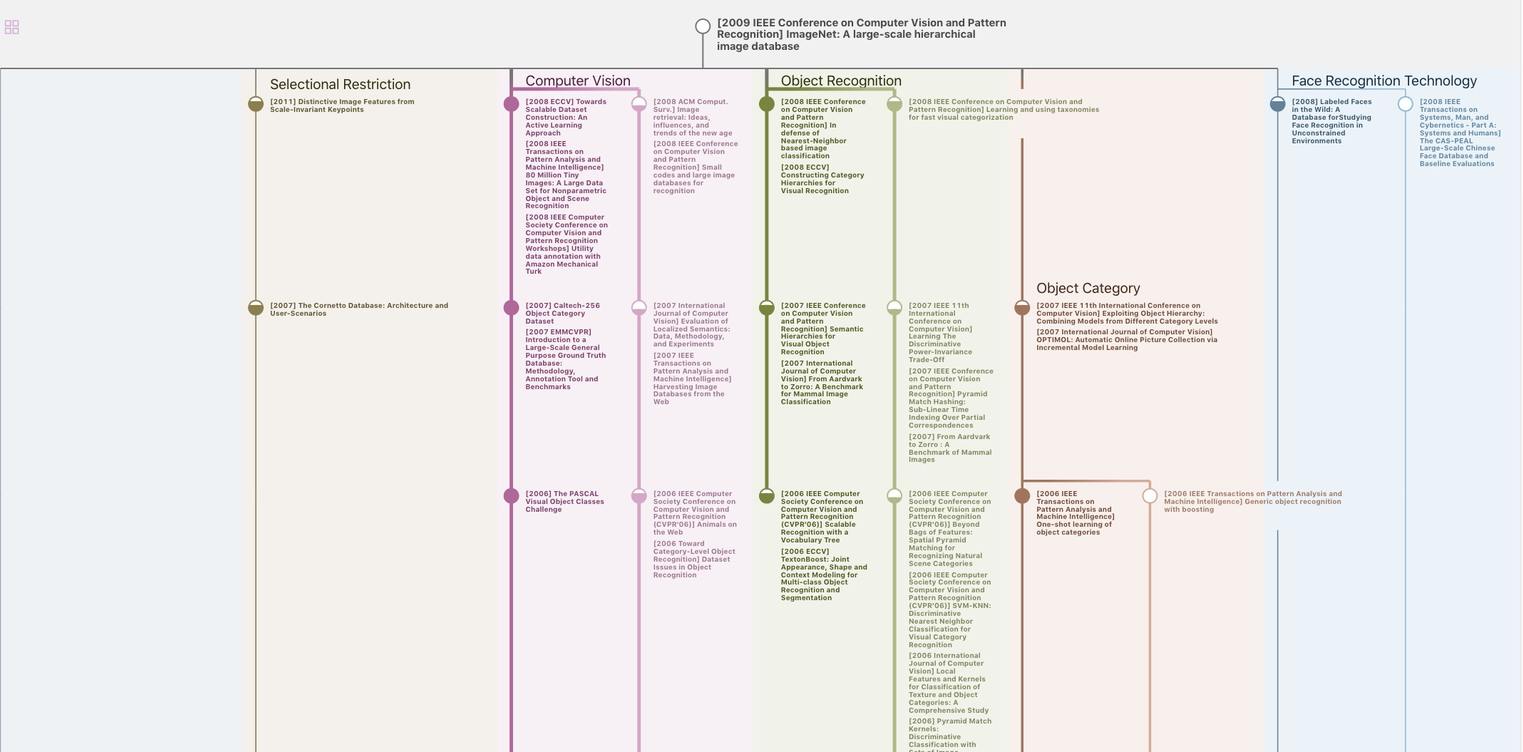
生成溯源树,研究论文发展脉络
Chat Paper
正在生成论文摘要