Automatic Fiducial Marker Detection And Localization In Ct Images: A Combined Approach
MEDICAL IMAGING 2020: IMAGE-GUIDED PROCEDURES, ROBOTIC INTERVENTIONS, AND MODELING(2021)
摘要
Patient-to-image registration is a key step for guidance in computer-assisted surgery during interventions like cochlear implant or deep brain stimulation surgeries. Automatizing fiducial detection and localization in pre-operative images of the patient can lead to better registration accuracy, reduced human errors and shorter intervention time. We present an algorithm that builds on earlier approaches with morphological functions and pose estimation algorithms. A Convolutional Neural Network is proposed for the fiducial classification task. A digital experiment, with cone-beam CT imaging software, is performed to determine the accuracy of the algorithm. The inputs to this software are virtual phantoms represented as 3D surface models (meshes) of skull and (screw and spherical) fiducial markers combined with specific imaging parameters like material properties, detector resolution, etc. The software generates realistic CT images for establishing a ground-truth measure to validate the algorithm. The localized fiducial positions in the image by the presented algorithm were compared to the actual known positions in the phantom models. The difference represents the fiducial localization error (FLE). Validation data sets with different slice thicknesses contain screws and spherical markers of different dimensions. The achieved FLE mean and its standard deviation for a screw and spherical marker are 58 (14) mu m and 14 (6) mu m, respectively. Large marker volume and smaller voxel size yield smaller FLEs. Furthermore, we found that attenuating noise by mesh smoothing has a minor effect on localization accuracy.
更多查看译文
关键词
automatization, fiducial marker, segmentation, deep learning classification, localization, phantom models, virtual CT
AI 理解论文
溯源树
样例
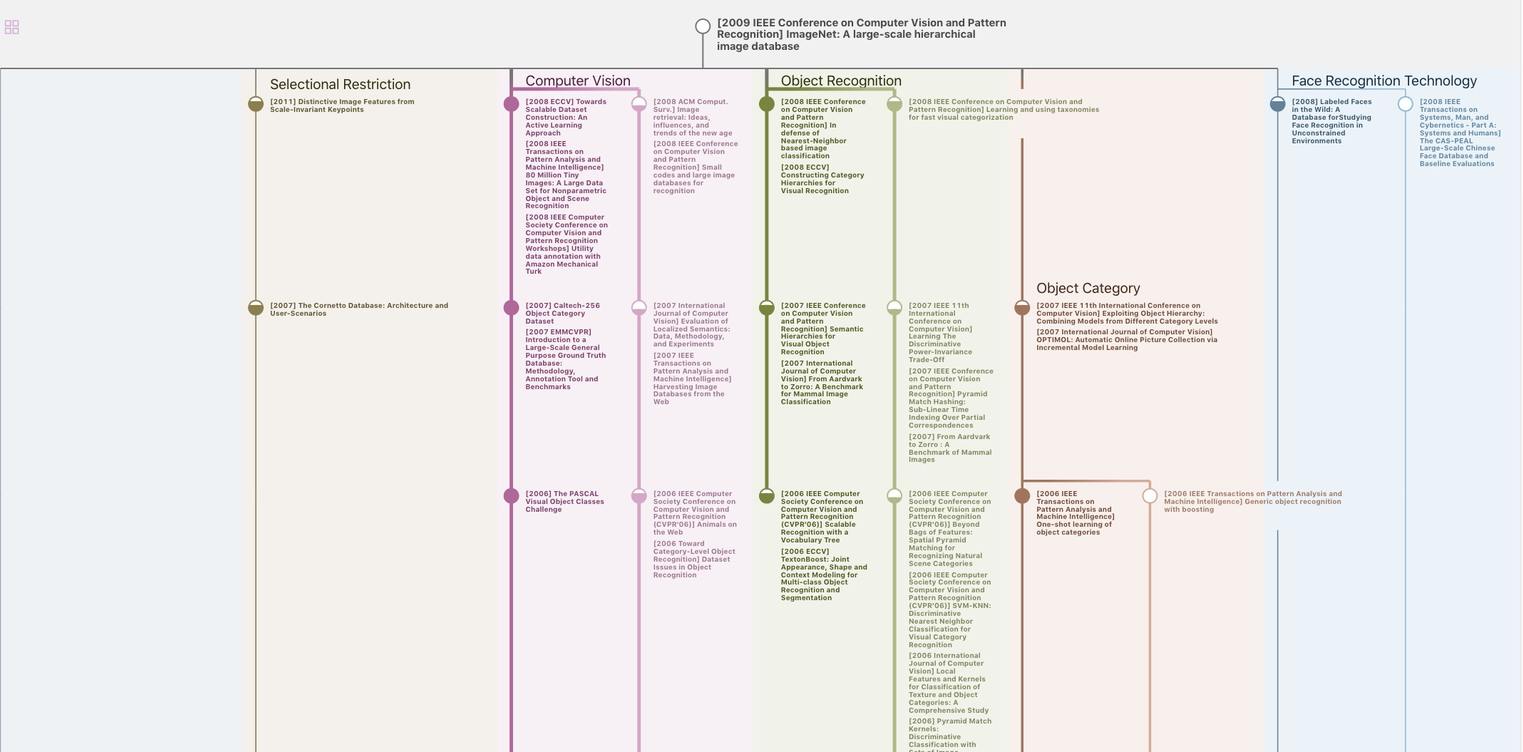
生成溯源树,研究论文发展脉络
Chat Paper
正在生成论文摘要