Auto-Sklearn 2.0: Hands-free AutoML via Meta-Learning
arxiv(2022)
摘要
Automated Machine Learning (AutoML) supports practitioners and researchers with the tedious task of designing machine learning pipelines and has recently achieved substantial success. In this paper, we introduce new AutoML approaches motivated by our winning submission to the second ChaLearn AutoML challenge. We develop PoSH Auto-sklearn, which enables AutoML systems to work well on large datasets under rigid time limits by using a new, simple and meta-feature-free meta-learning technique and by employing a suc-cessful bandit strategy for budget allocation. However, PoSH Auto-sklearn introduces even more ways of running AutoML and might make it harder for users to set it up correctly. Therefore, we also go one step further and study the design space of AutoML itself, propos-ing a solution towards truly hands-free AutoML. Together, these changes give rise to the next generation of our AutoML system, Auto-sklearn 2.0. We verify the improvements by these additions in an extensive experimental study on 39 AutoML benchmark datasets. We conclude the paper by comparing to other popular AutoML frameworks and Auto-sklearn 1.0, reducing the relative error by up to a factor of 4.5, and yielding a performance in 10 minutes that is substantially better than what Auto-sklearn 1.0 achieves within an hour.
更多查看译文
关键词
Automated machine learning,hyperparameter optimization,meta-learning,automated AutoML,benchmark
AI 理解论文
溯源树
样例
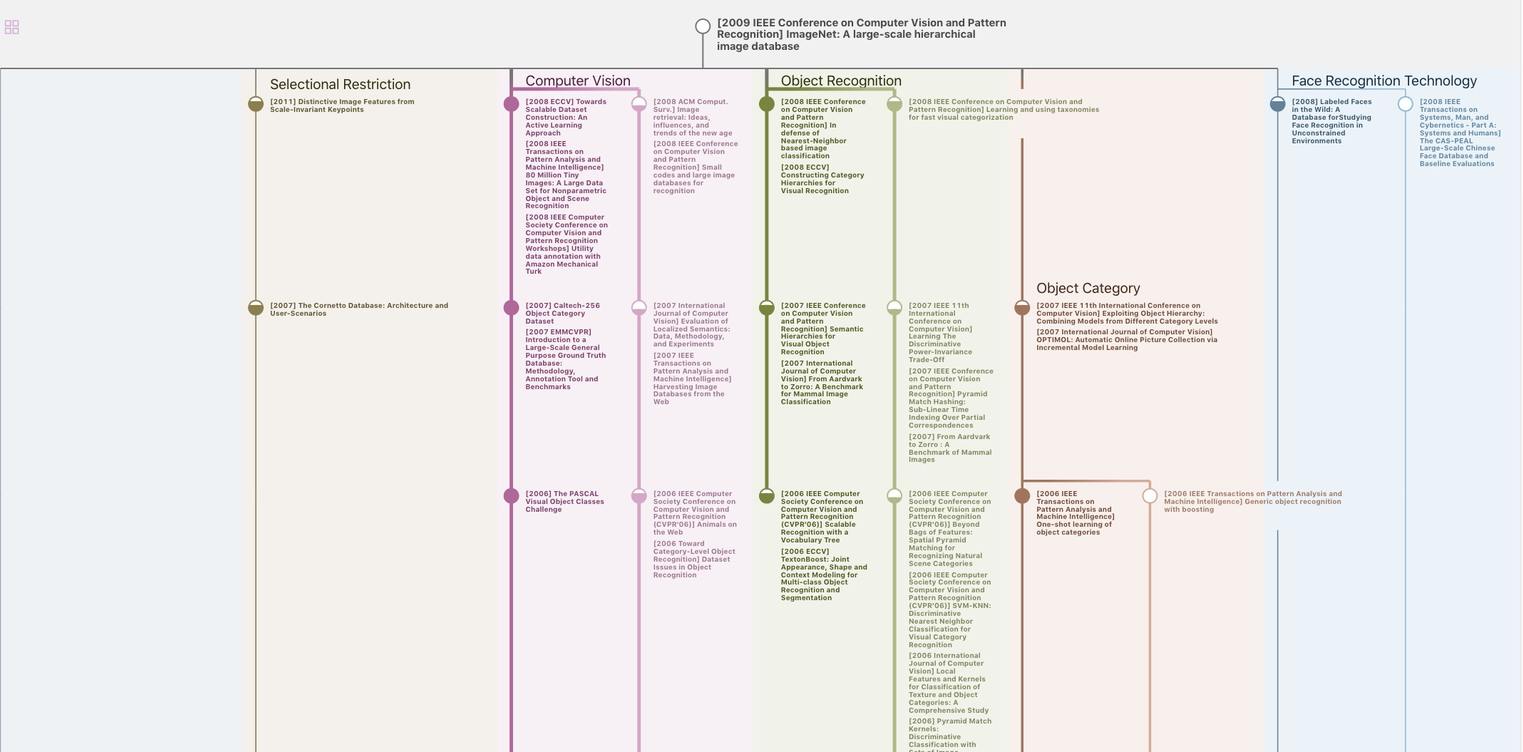
生成溯源树,研究论文发展脉络
Chat Paper
正在生成论文摘要