Chemical Class Prediction of Unknown Biomolecules Using Ion Mobility-Mass Spectrometry and Machine Learning: SIFTER.
ANALYTICAL CHEMISTRY(2020)
摘要
This work presents a machine learning algorithm referred to as the supervised inference of feature taxonomy from ensemble randomization (SIFTER), which supports the identification of features derived from untargeted ion mobility-mass spectrometry (IM-MS) experiments. SIFTER utilizes random forest machine learning on three analytical measurements derived from IM-MS (collision cross section, CCS), mass-to-charge (m/z), and mass defect (Delta m) to classify unknown features into a taxonomy of chemical kingdom, super class, class, and subclass. Each of these classifications is assigned a calculated probability as well as alternate classifications with associated probabilities. After optimization, SIFTER was tested against a set of molecules not used in the training set. The average success rate in classifying all four taxonomy categories correctly was found to be >99%. Analysis of molecular features detected from a complex biological matrix and not used in the training set yielded a lower success rate where all four categories were correctly predicted for similar to 80% of the compounds. This decline in performance is in part due to incompleteness of the training set across all potential taxonomic categories, but also resulting from a nearest-neighbor bias in the random forest algorithm. Ongoing efforts are focused on improving the class prediction accuracy of SIFTER through expansion of empirical data sets used for training as well as improvements to the core algorithm.
更多查看译文
AI 理解论文
溯源树
样例
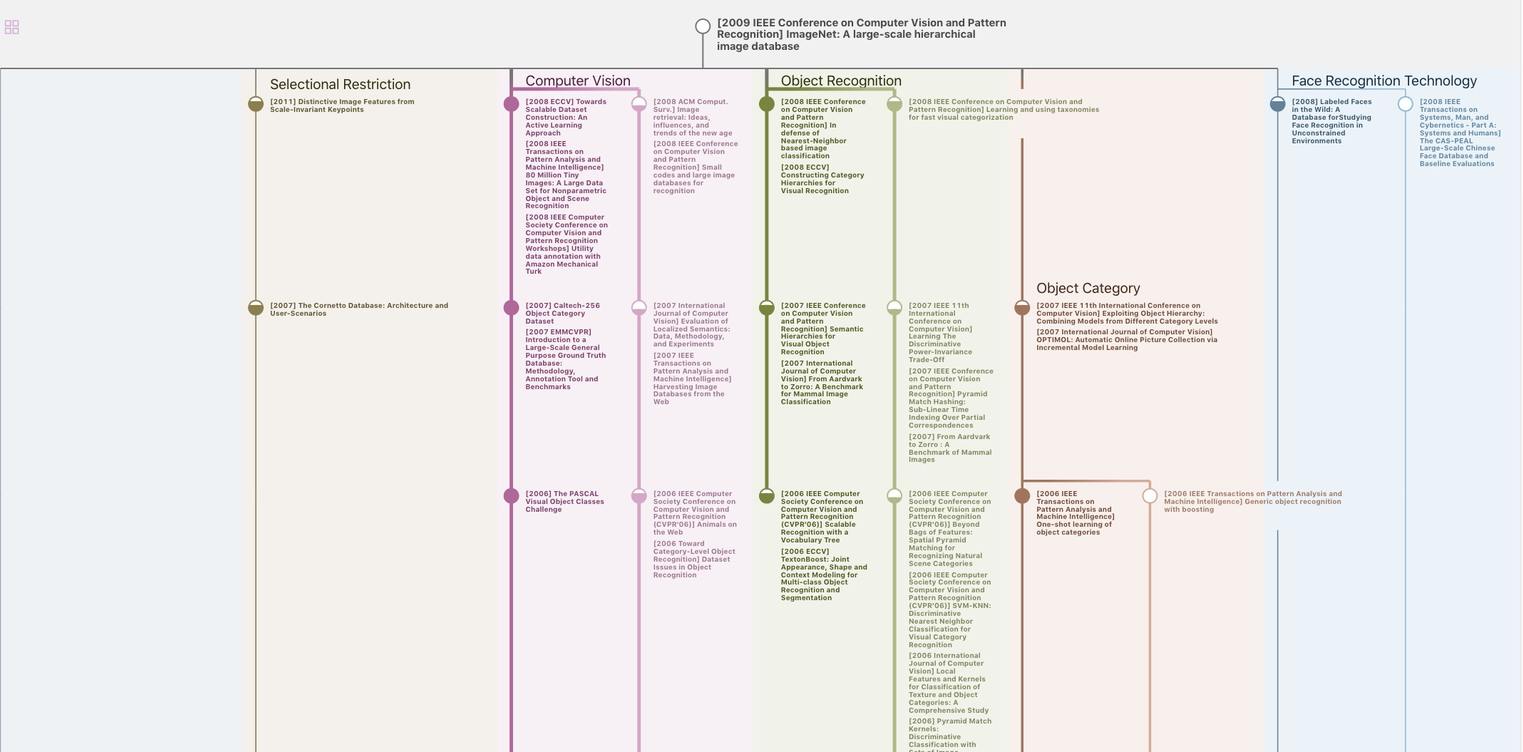
生成溯源树,研究论文发展脉络
Chat Paper
正在生成论文摘要