The LoCA Regret: A Consistent Metric to Evaluate Model-Based Behavior in Reinforcement Learning
NIPS 2020(2020)
摘要
Deep model-based Reinforcement Learning (RL) has the potential to substantially improve the sample-efficiency of deep RL. While various challenges have long held it back, a number of papers have recently come out reporting success with deep model-based methods. This is a great development, but the lack of a consistent metric to evaluate such methods makes it difficult to compare various approaches. For example, the common single-task sample-efficiency metric conflates improvements due to model-based learning with various other aspects, such as representation learning, making it difficult to assess true progress on model-based RL. To address this, we introduce an experimental setup to evaluate model-based behavior of RL methods, inspired by work from neuroscience on detecting model-based behavior in humans and animals. Our metric based on this setup, the Local Change Adaptation (LoCA) regret, measures how quickly an RL method adapts to a local change in the environment. Our metric can identify model-based behavior, even if the method uses a poor representation and provides insight in how close a method's behavior is from optimal model-based behavior. We use our setup to evaluate the model-based behavior of MuZero on a variation of the classic Mountain Car task.
更多查看译文
关键词
loca regret,learning,consistent metric,model-based
AI 理解论文
溯源树
样例
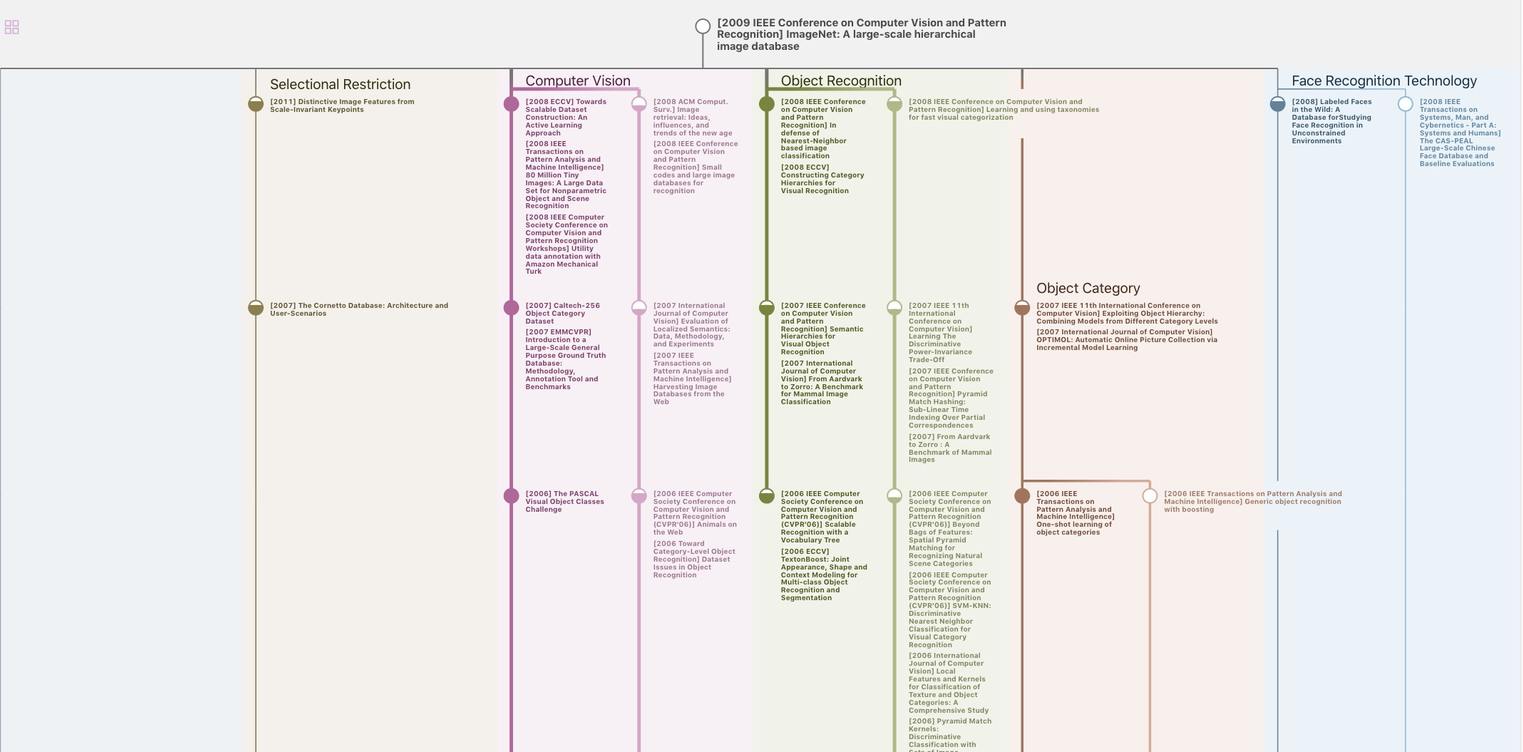
生成溯源树,研究论文发展脉络
Chat Paper
正在生成论文摘要