ResNeSt: Split-Attention Networks
IEEE Conference on Computer Vision and Pattern Recognition(2022)
摘要
The ability to learn richer network representations generally boosts the performance of deep learning models. To improve representation-learning in convolutional neural networks, we present a multi-branch architecture, which applies channel-wise attention across different network branches to leverage the complementary strengths of both feature-map attention and multi-path representation. Our proposed Split-Attention module provides a simple and modular computation block that can serve as a drop-in replacement for the popular residual block, while producing more diverse representations via cross-feature interactions. Adding a Split-Attention module into the architecture design space of RegNet-Y and FBNetV2 directly improves the performance of the resulting network. Replacing residual blocks with our Split-Attention module, we further design a new variant of the ResNet model, named ResNeSt, which outperforms EfficientNet in terms of the accuracy/latency trade-off.
更多查看译文
关键词
channel-wise attention,different network branches,feature-map attention,split-attention module,simple computation block,modular computation block,popular residual block,diverse representations,richer network representations,deep learning models,representation-learning,convolutional neural networks,multibranch architecture,ResNeSt,split-attention networks,RegNet-Y,FBNetV2
AI 理解论文
溯源树
样例
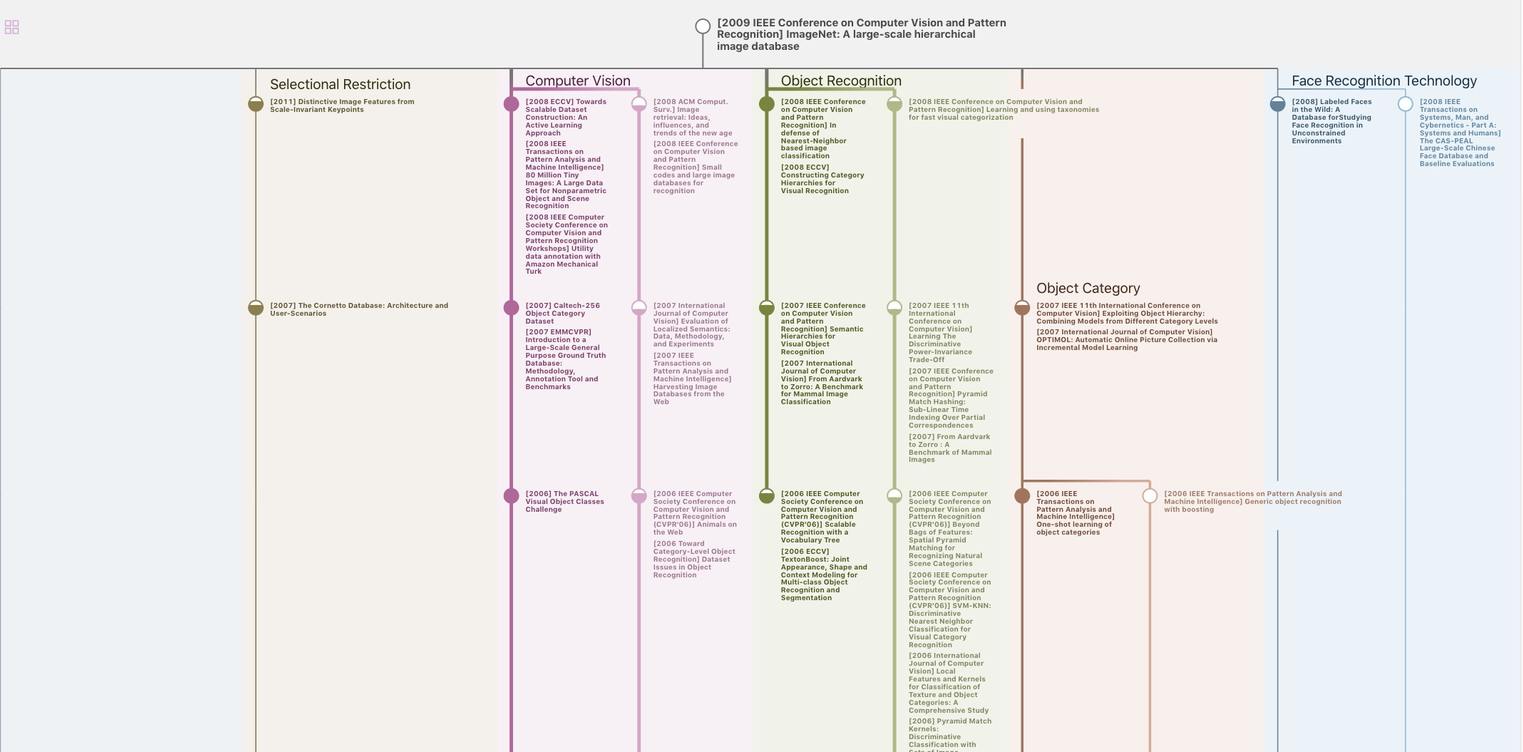
生成溯源树,研究论文发展脉络
Chat Paper
正在生成论文摘要