Interval Neural Networks as Instability Detectors for Image Reconstructions
Bildverarbeitung für die Medizin(2021)
摘要
This work investigates the detection of instabilities that may occur when utilizing deep learning models for image reconstruction tasks. Although neural networks often empirically outperform traditional reconstruction methods, their usage for sensitive medical applications remains controversial. Indeed, in a recent series of works, it has been demonstrated that deep learning approaches are susceptible to various types of instabilities, caused for instance by adversarial noise or out-of-distribution features. It is argued that this phenomenon can be observed regardless of the underlying architecture and that there is no easy remedy. Based on this insight, the present work demonstrates on two use cases how uncertainty quantification methods can be employed as instability detectors. In particular, it is shown that the recently proposed Interval Neural Networks are highly effective in revealing instabilities of reconstructions. Such an ability is crucial to ensure a safe use of deep learning-based methods for medical image reconstruction.
更多查看译文
关键词
instability detectors,neural networks,interval
AI 理解论文
溯源树
样例
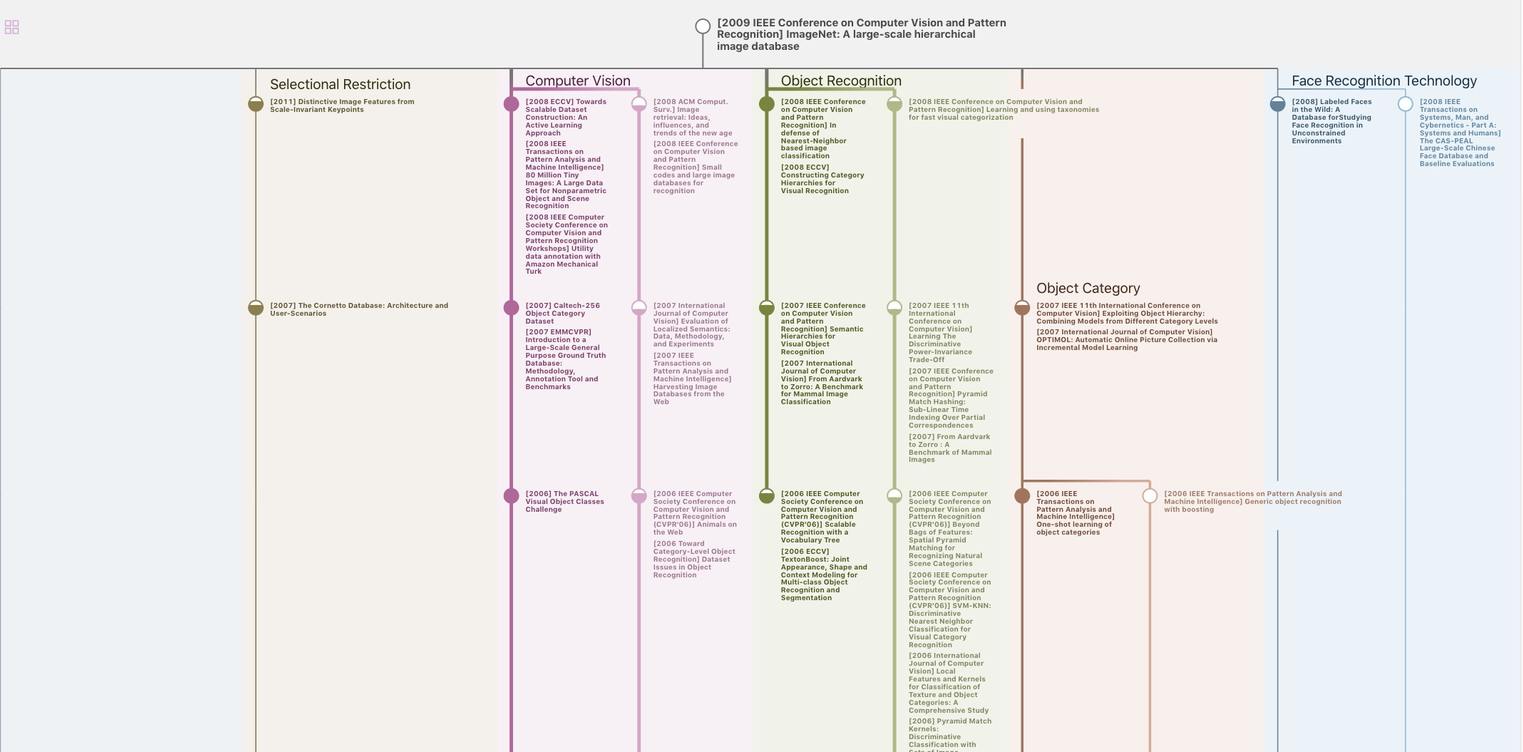
生成溯源树,研究论文发展脉络
Chat Paper
正在生成论文摘要