Toward Subgraph Guided Knowledge Graph Question Generation with Graph Neural Networks
arxiv(2020)
摘要
Knowledge graph question generation (QG) aims to generate natural language questions from KG and target answers. Most previous works mainly focusing on the simple setting are to generate questions from a single KG triple. In this work, we focus on a more realistic setting, where we aim to generate questions from a KG subgraph and target answers. In addition, most of previous works built on either RNN-based or Transformer-based models to encode a KG sugraph, which totally discard the explicit structure information contained in a KG subgraph. To address this issue, we propose to apply a bidirectional Graph2Seq model to encode the KG subgraph. In addition, we enhance our RNN decoder with node-level copying mechanism to allow directly copying node attributes from the input graph to the output question. We also explore different ways of initializing node/edge embeddings and handling multi-relational graphs. Our model is end-to-end trainable and achieves new state-of-the-art scores, outperforming existing methods by a significant margin on the two benchmarks.
更多查看译文
关键词
Deep learning,graph neural networks (GNNs),knowledge graphs (KGs),natural language (NL) processing,question generation (QG)
AI 理解论文
溯源树
样例
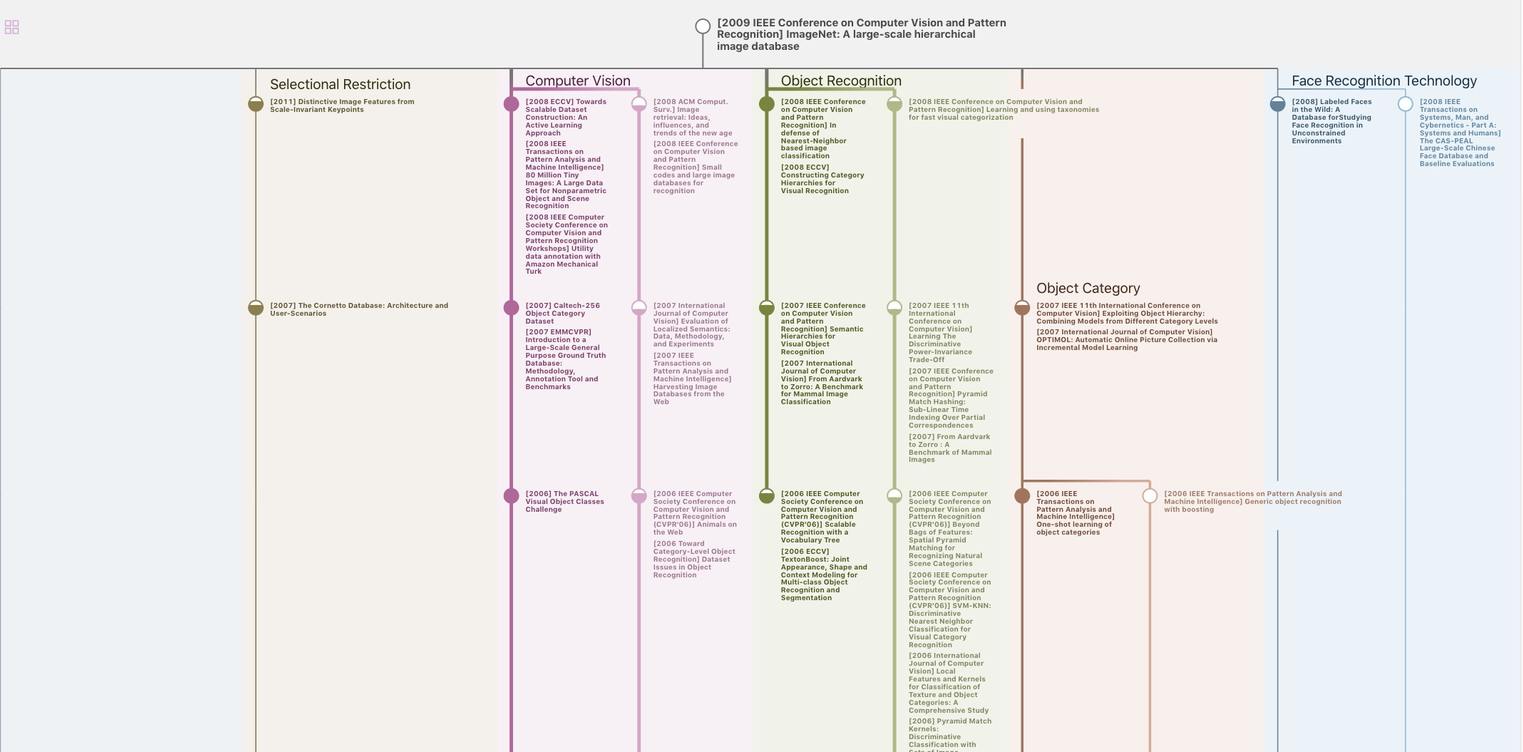
生成溯源树,研究论文发展脉络
Chat Paper
正在生成论文摘要