Self-challenging Improves Cross-Domain Generalization
European Conference on Computer Vision(2020)
摘要
Convolutional Neural Networks (CNN) conduct image classification by activating dominant features that correlated with labels. When the training and testing data are under similar distributions, their dominant features are similar, leading to decent test performance. The performance is nonetheless unmet when tested with different distributions, leading to the challenges in cross-domain image classification. We introduce a simple training heuristic, Representation Self-Challenging (RSC), that significantly improves the generalization of CNN to the out-of-domain data. RSC iteratively challenges (discards) the dominant features activated on the training data, and forces the network to activate remaining features that correlate with labels. This process appears to activate feature representations applicable to out-of-domain data without prior knowledge of the new domain and without learning extra network parameters. We present the theoretical properties and conditions of RSC for improving cross-domain generalization. The experiments endorse the simple, effective, and architecture-agnostic nature of our RSC method.
更多查看译文
关键词
Cross-domain generalization,Robustness
AI 理解论文
溯源树
样例
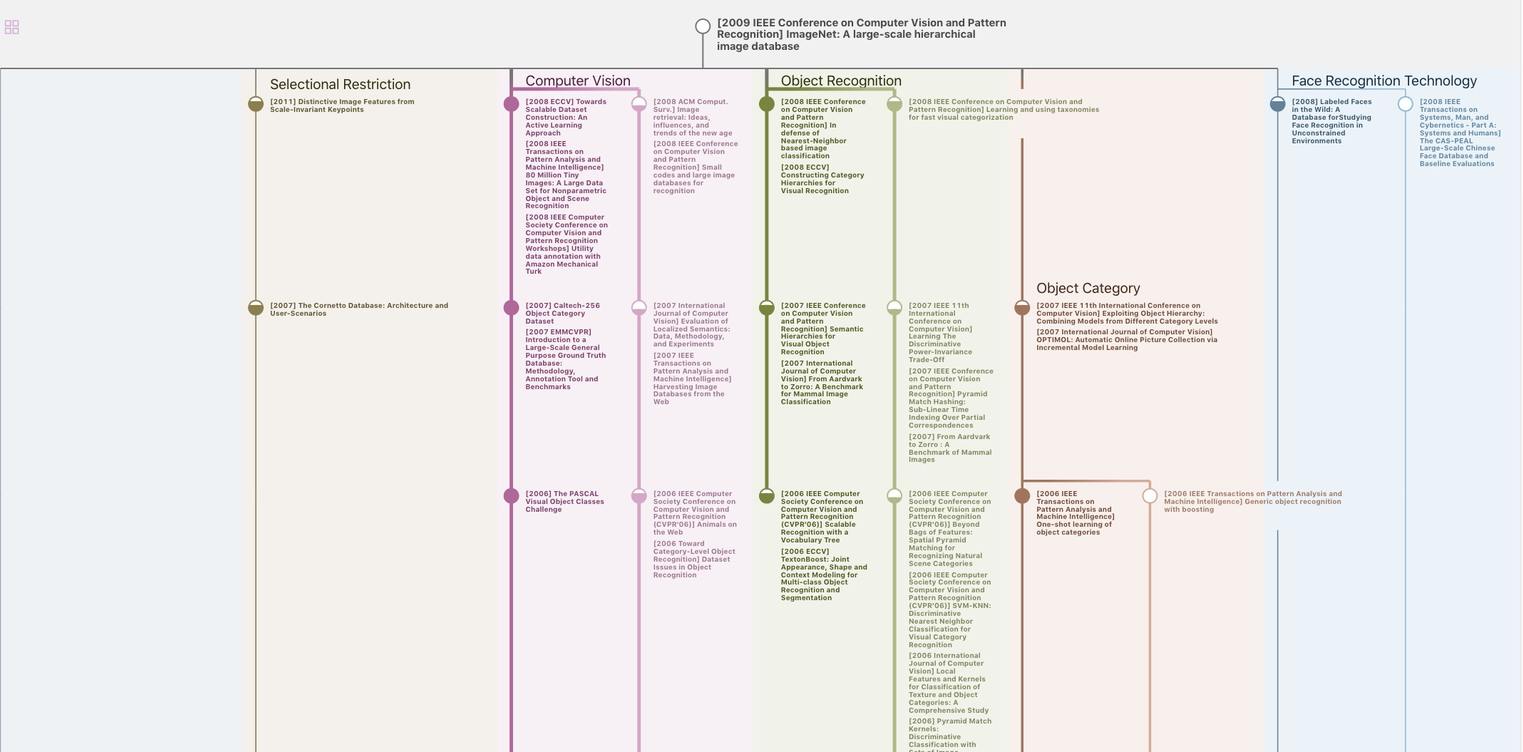
生成溯源树,研究论文发展脉络
Chat Paper
正在生成论文摘要