R-fMRI reconstruction from k-t undersampled data using a subject-invariant dictionary model and VB-EM with nested minorization.
Medical Image Analysis(2020)
摘要
•Faster R-fMRI imaging allows higher spatial resolution and more reliable functional connectivity analysis.•We propose a novel undersampling scheme in k-space and time (k-t) both to provide the necessary speedup.•We propose a novel dictionary-based model on the signal, which is robust, spatially regularized, and subject invariant by leveraging an equivalence-class structure on the dictionary.•We propose a novel Bayesian inference framework based on variational Bayesian expectation maximization with nested minorization (VB-EM-NM), which allows us to estimate per-voxel uncertainty in the reconstruction.•Empirical evaluation of (i) R-fMRI reconstructions from simulated data and (ii) functional-network estimates from reconstructions of brain R-fMRI demonstrate that our framework improves over the state of the art, and, additionally, enables significantly higher spatial resolution.
更多查看译文
关键词
R-fMRI,Undersample,Reconstruction,Variational Bayesian factorization,Expectation maximization,Nested minorization,Dictionary prior,Robust,Subject-invariant,Sparse,Spatial regularization
AI 理解论文
溯源树
样例
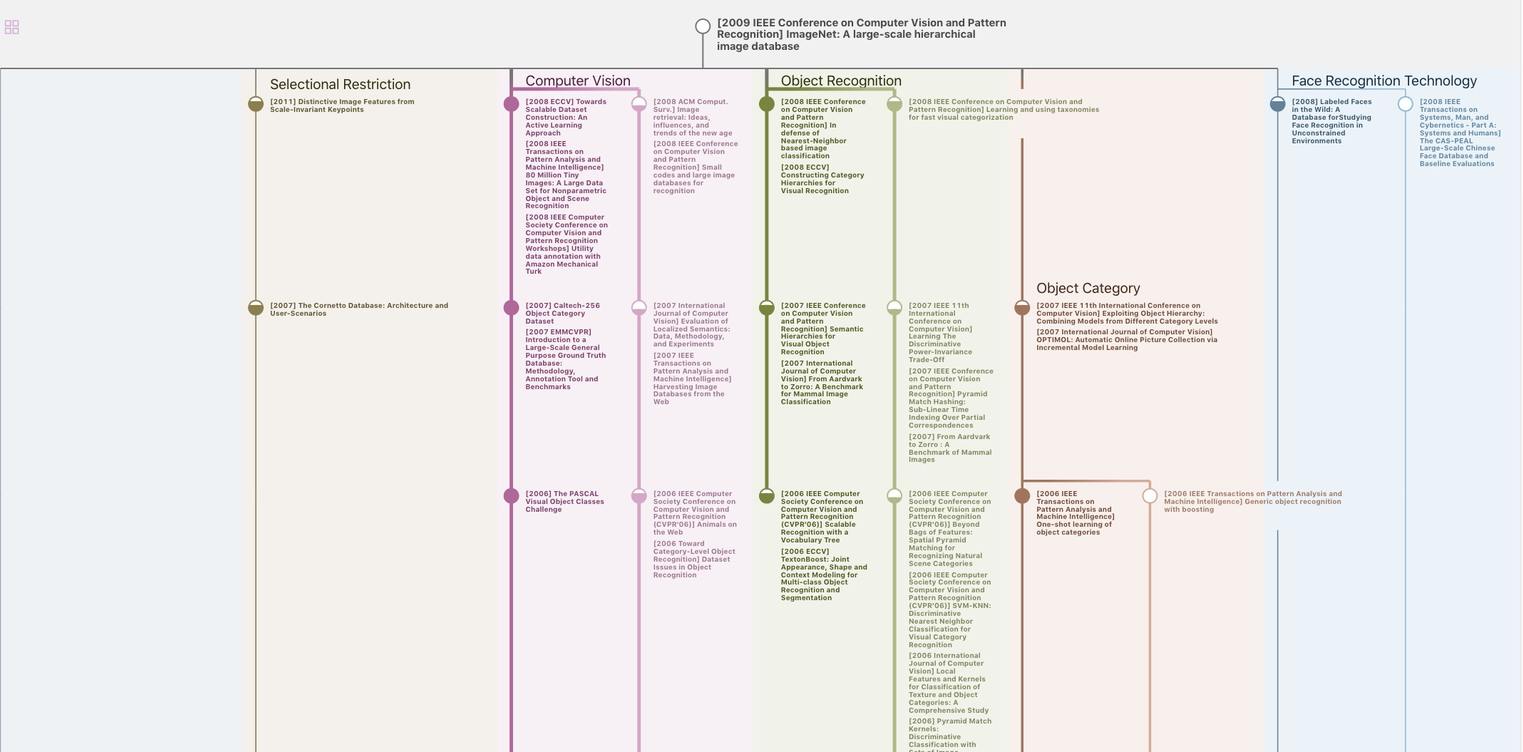
生成溯源树,研究论文发展脉络
Chat Paper
正在生成论文摘要