Improving Multiperson Pose Estimation by Mask-aware Deep Reinforcement Learning
ACM Transactions on Multimedia Computing, Communications, and Applications(2020)
摘要
Research on single-person pose estimation based on deep neural networks has recently witnessed progress in both accuracy and execution efficiency. However, multiperson pose estimation is still a challenging topic, partially because the object regions are selected greedily from proposals via class-agnostic nonmaximum suppression (NMS), and the misalignment in the redundant detection yields inaccurate human poses. Therefore, we consider how to obtain the optimal input in human pose estimation under conditions in which intermediate label information is not available. As supervised learning–based alignment does not generalize well to unseen samples in the human pose space, in this article, we present a mask-aware deep reinforcement learning approach to modify the detection result. We use mask information to remove the adverse effects from the cluttered background and to select the optimal action according to the revised reward function. We also propose a new regularization term to punish joints that are outside of the silhouette region in the human pose estimation stage. We evaluate our approach on the MPII Multiperson dataset and the MS-COCO Keypoints Challenge. The results show that our approach yields competing inference results when it is compared to the other state-of-the-art approaches.
更多查看译文
关键词
Computer vision,deep learning,regularization,reinforcement learning
AI 理解论文
溯源树
样例
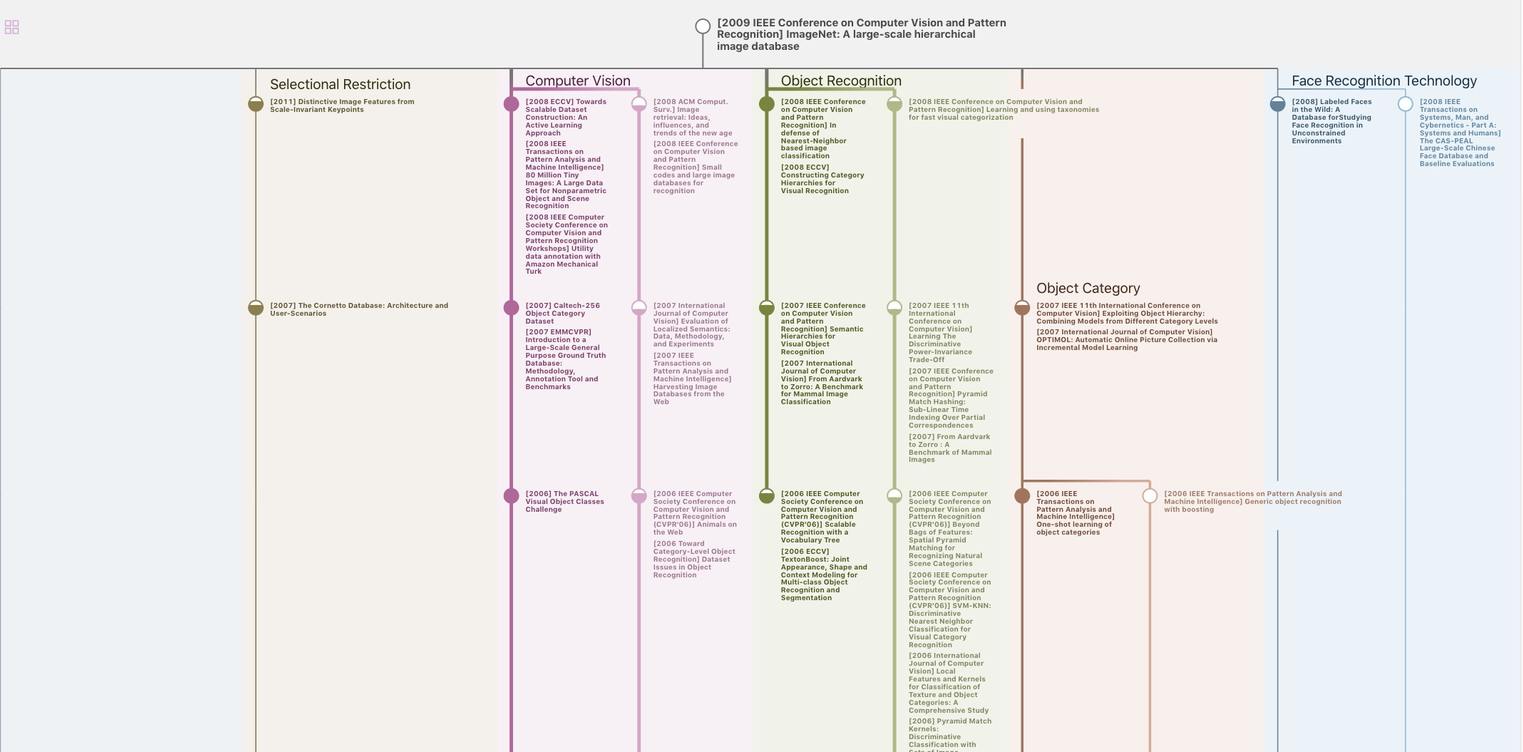
生成溯源树,研究论文发展脉络
Chat Paper
正在生成论文摘要