A Request-level Guaranteed Delivery Advertising Planning: Forecasting and Allocation
KDD '20: The 26th ACM SIGKDD Conference on Knowledge Discovery and Data Mining Virtual Event CA USA July, 2020(2020)
摘要
The guaranteed delivery model is widely used in online advertising. The publisher sells impressions in advance by promising to serve each advertiser an agreed-upon number of target impressions that satisfy specific attribute requirements over a fixed time period. Previous efforts usually model the service as a crowd-level or user-level supply allocation problem and focus on searching optimal allocation for online serving, assuming that forecasts of supply are available and contracts are already signed. Existing techniques are not sufficient to meet the needs of today's industry trends: 1) advertisers pursue more precise targeting, which requires not only user-level attributes but also request-level attributes; 2) users prefer more friendly ad serving, which imposes more diverse serving constraints; 3) the bottleneck of the publisher's revenue growth lies in not only the ad serving, but also the forecast accuracy and sales strategy. These issues are non-trivial to address, since the scale of the request-level model is orders of magnitude larger than that of the crowd-level or user-level models. Facing the challenges, we present a holistic design of a request-level guaranteed delivery advertising planning system with careful optimization for all three critical components including impression forecasting, selling and serving. Our system has been deployed in the Tencent online guaranteed delivery advertising system serving billion level users for nearly one year. Evaluations on large-scale real data and the performance of the deployed system both demonstrate that our design can significantly increase the request-level impression forecast accuracy and delivery rate.
更多查看译文
关键词
Guaranteed Delivery Advertising, Request-level Impression Forecasting, Advertisement Allocation
AI 理解论文
溯源树
样例
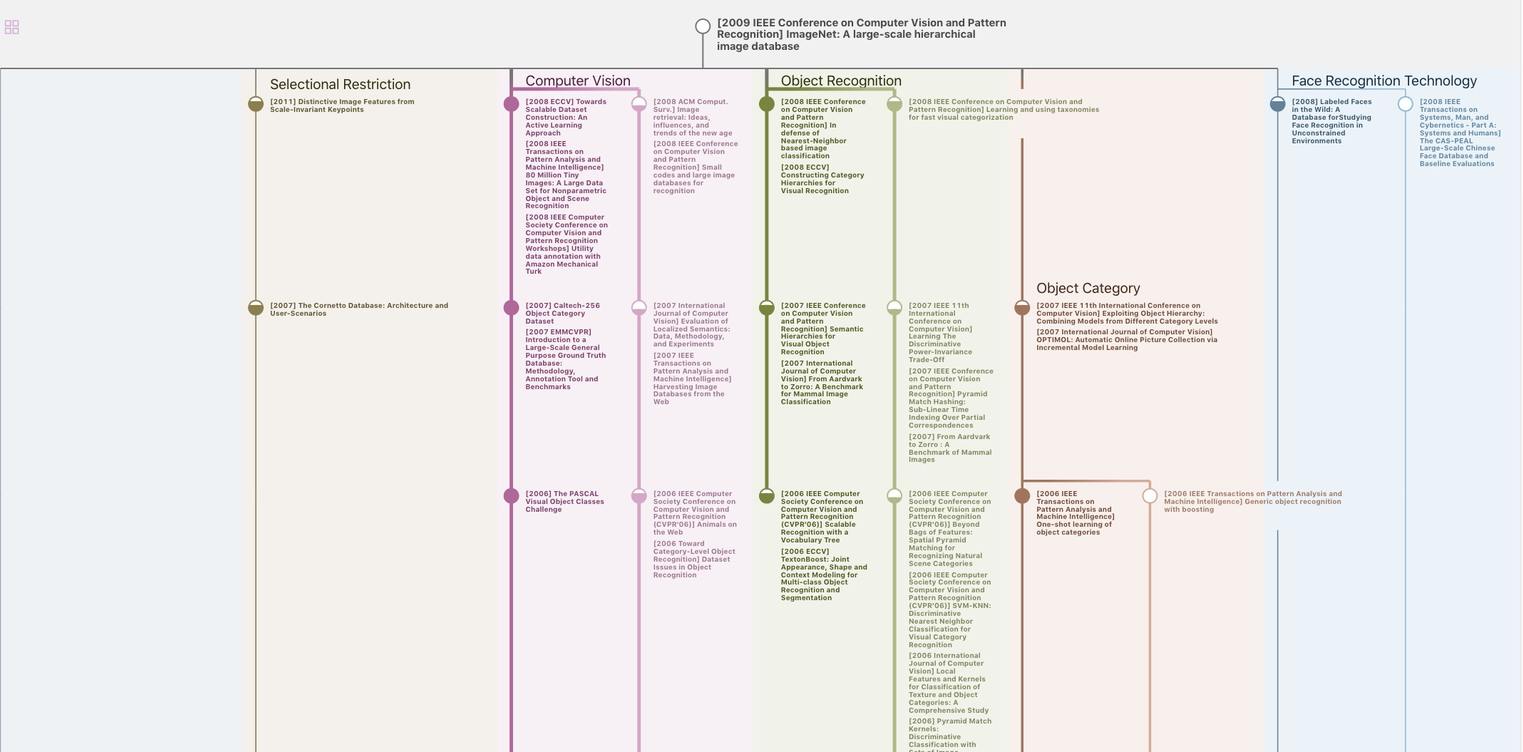
生成溯源树,研究论文发展脉络
Chat Paper
正在生成论文摘要