Isolation Distributional Kernel: A new tool for kernel based anomaly detection
KDD 2020(2020)
摘要
We introduce Isolation Distributional Kernel as a new way to measure the similarity between two distributions. Existing approaches based on kernel mean embedding, which converts a point kernel to a distributional kernel, have two key issues: the point kernel employed has a feature map with intractable dimensionality; and it is data independent. This paper shows that Isolation Distributional Kernel (IDK), which is based on a data dependent point kernel, addresses both key issues. We demonstrate IDK's efficacy and efficiency as a new tool for kernel based anomaly detection. Without explicit learning, using IDK alone outperforms existing kernel based anomaly detector OCSVM and other kernel mean embedding methods that rely on Gaussian kernel. We reveal for the first time that an effective kernel based anomaly detector based on kernel mean embedding must employ a characteristic kernel which is data dependent.
更多查看译文
关键词
Distributional Kernel, Kernel Mean Embedding, Anomaly Detection
AI 理解论文
溯源树
样例
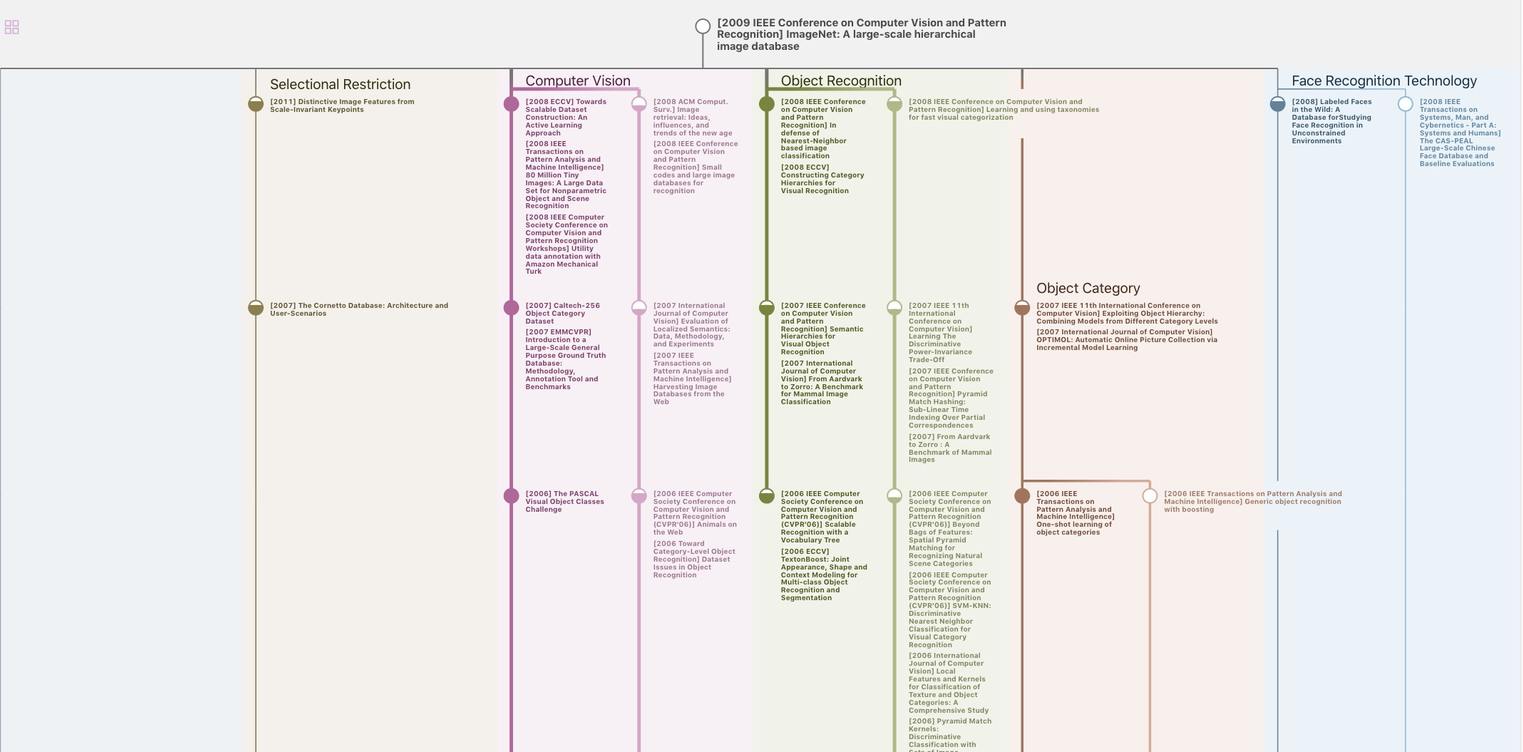
生成溯源树,研究论文发展脉络
Chat Paper
正在生成论文摘要