CRSAL: Conversational Recommender Systems with Adversarial Learning
ACM Transactions on Information Systems(2020)
摘要
AbstractRecommender systems have been attracting much attention from both academia and industry because of their ability to capture user interests and generate personalized item recommendations. As the life pace in contemporary society speeds up, traditional recommender systems are inevitably limited by their disconnected interaction styles and low adaptivity to users’ evolving demands. Consequently, conversational recommender systems emerge as a prospective research area, where an intelligent dialogue agent is integrated with a recommender system. Conversational recommender systems possess the ability to accurately understand end-users’ intent or request and generate human-like dialogue responses when performing recommendations. However, existing conversational recommender systems only allow the systems to ask users for more preference information, while users’ further questions and concerns about the recommended items (e.g., enquiring the location of a recommended restaurant) can hardly be addressed. Though the recent task-oriented dialogue systems allow for two-way communications, they are not easy to train because of their high dependence on human guidance in terms of user intent recognition and system response generation. Hence, to enable two-way human-machine communications and tackle the challenges brought by manually crafted rules, we propose Conversational Recommender System with Adversarial Learning (CRSAL), a novel end-to-end system to tackle the task of conversational recommendation. In CRSAL, we innovatively design a fully statistical dialogue state tracker coupled with a neural policy agent to precisely capture each user’s intent from limited dialogue data and generate conversational recommendation actions. We further develop an adversarial Actor-Critic reinforcement learning approach to adaptively refine the quality of generated system actions, thus ensuring coherent human-like dialogue responses. Extensive experiments on two benchmark datasets fully demonstrate the superiority of CRSAL on conversational recommendation tasks.
更多查看译文
关键词
Conversational recommender systems, dialogue systems, adversarial learning, deep neural networks
AI 理解论文
溯源树
样例
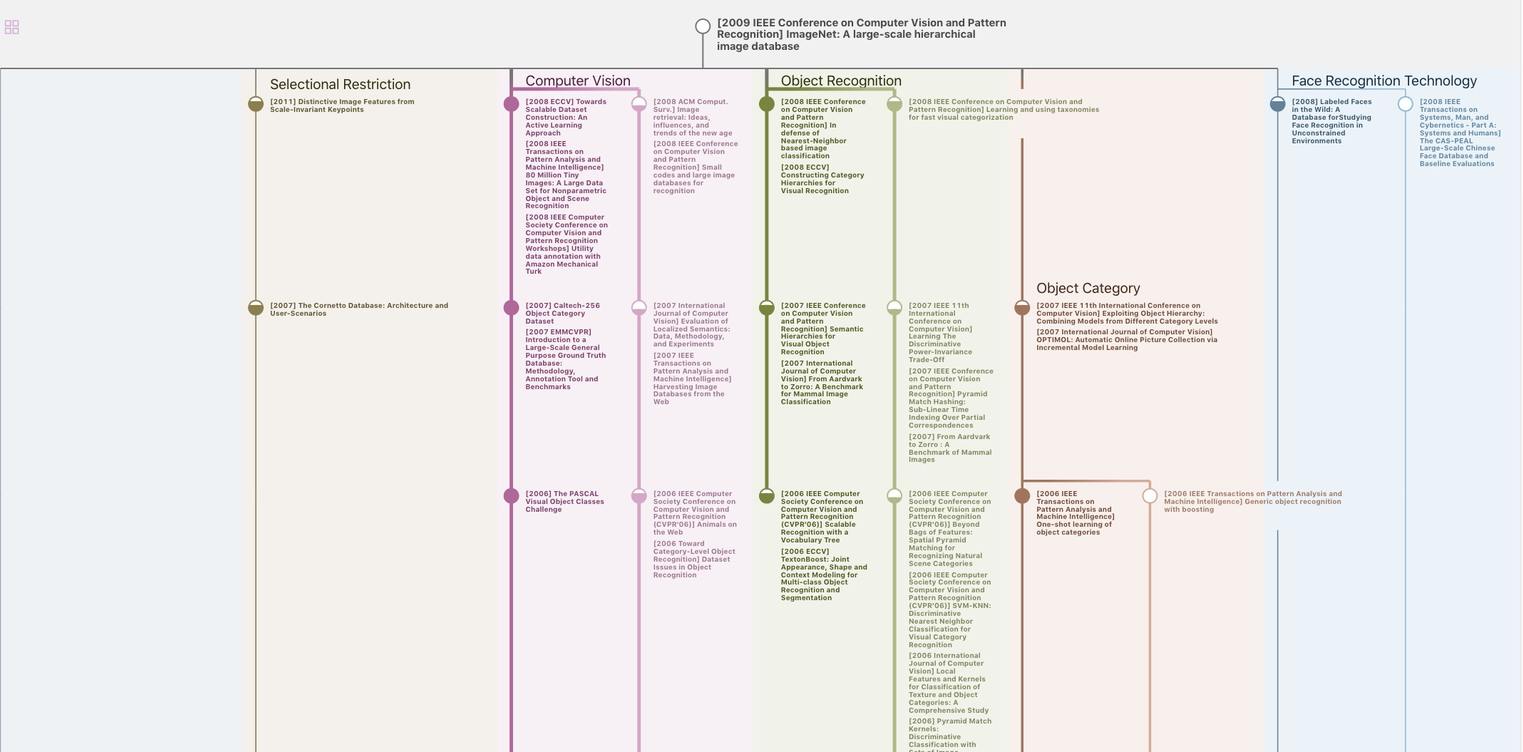
生成溯源树,研究论文发展脉络
Chat Paper
正在生成论文摘要