Graphical Convergence of Subgradien s in Nonconvex Optimization and Learning
MATHEMATICS OF OPERATIONS RESEARCH(2022)
摘要
We investigate the stochastic optimization problem of minimizing population risk, where the loss defining the risk is assumed to be weakly convex. Compositions of Lipschitz convex functions with smooth maps are the primary examples of such losses. We analyze the estimation quality of such nonsmooth and nonconvex problems by their sample average approximations. Our main results establish dimension-dependent rates on subgradient estimation in full generality and dimension-independent rates when the loss is a generalized linear model. As an application of the developed techniques, we analyze the nonsmooth landscape of a robust nonlinear regression problem.
更多查看译文
关键词
subdifferential, stability, population risk, sample average approximation, weak convexity, Moreau envelope, graphical convergence
AI 理解论文
溯源树
样例
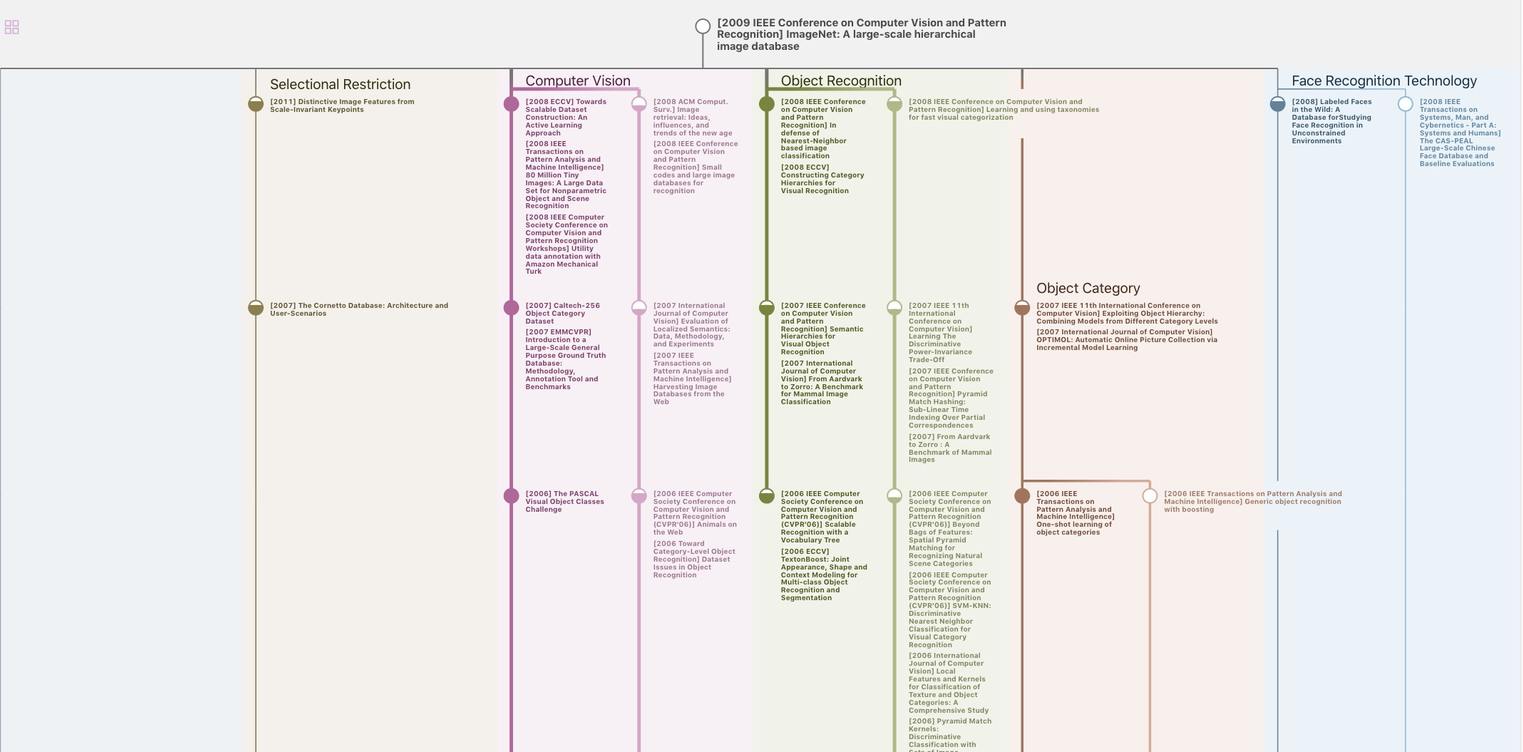
生成溯源树,研究论文发展脉络
Chat Paper
正在生成论文摘要