Robust Attribute and Structure Preserving Graph Embedding.
PAKDD (2)(2020)
摘要
Graph embedding methods are useful for a wide range of graph analysis tasks including link prediction and node classification. Most graph embedding methods learn only the topological structure of graphs. Nevertheless, it has been shown that the incorporation of node attributes is beneficial in improving the expressive power of node embeddings. However, real-world graphs are often noisy in terms of structure and/or attributes (missing and/or erroneous edges/attributes). Most existing graph embedding methods are susceptible to this noise, as they do not consider uncertainty during the modelling process. In this paper, we introduce RASE, a Robust Attribute and Structure preserving graph Embedding model. RASE is a novel graph representation learning model which effectively preserves both graph structure and node attributes through a unified loss function. To be robust, RASE uses a denoising attribute auto-encoder to deal with node attribute noise, and models uncertainty in the embedding space as Gaussians to cope with graph structure noise. We evaluate the performance of RASE through an extensive experimental study on various real-world datasets. Results demonstrate that RASE outperforms state-of-the-art embedding methods on multiple graph analysis tasks and is robust to both structure and attribute noise.
更多查看译文
关键词
Robust graph embedding, Node classification, Link prediction
AI 理解论文
溯源树
样例
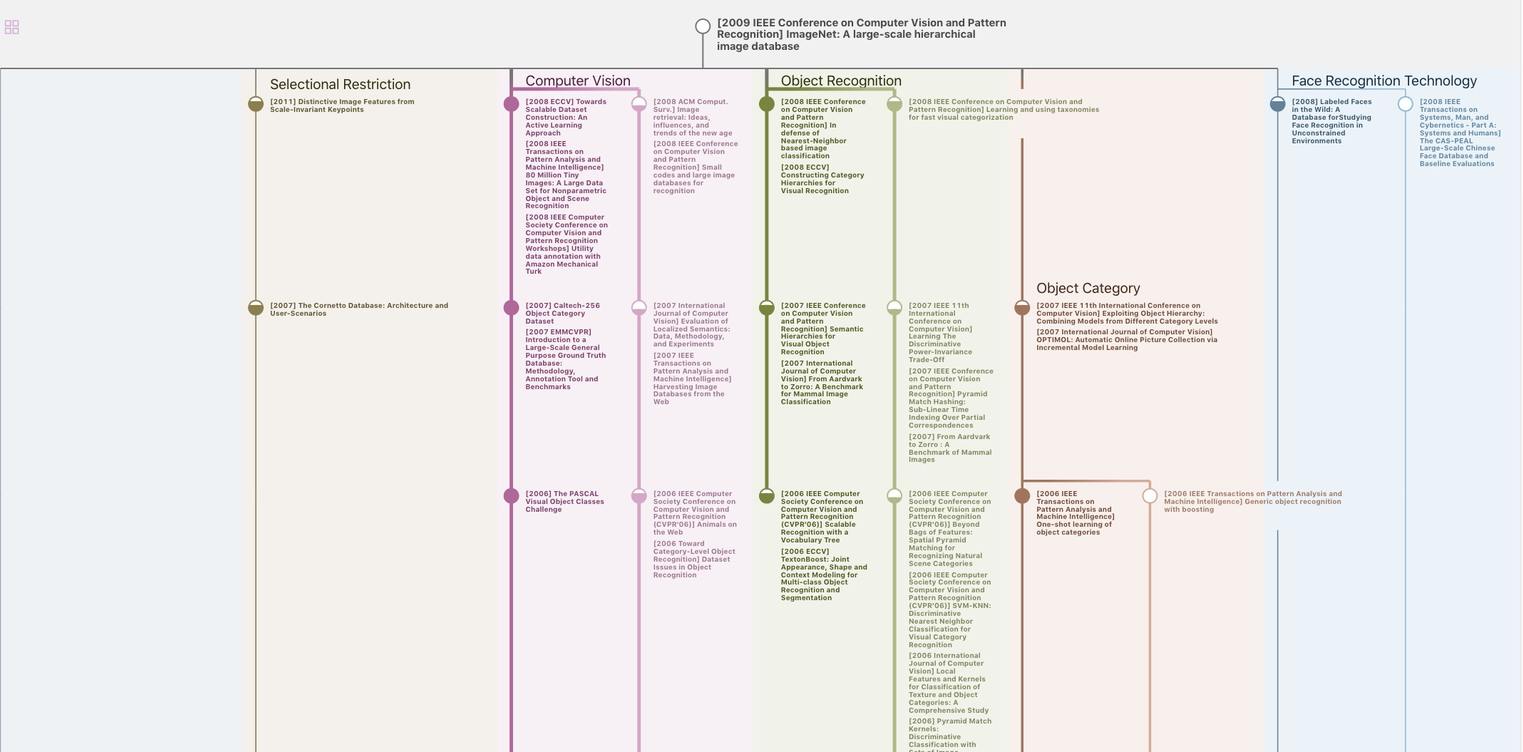
生成溯源树,研究论文发展脉络
Chat Paper
正在生成论文摘要