TADS: Learning Time-Aware Scheduling Policy with Dyna-Style Planning for Spaced Repetition
SIGIR '20: The 43rd International ACM SIGIR conference on research and development in Information Retrieval Virtual Event China July, 2020(2020)
摘要
Spaced repetition technique aims at improving long-term memory retention for human students by exploiting repeated, spaced reviews of learning contents. The study of spaced repetition focuses on designing an optimal policy to schedule the learning contents. To the best of our knowledge, none of the existing methods based on reinforcement learning take into account the varying time intervals between two adjacent learning events of the student, which, however, are essential to determine real-world schedule. In this paper, we aim to learn a scheduling policy that fully exploits the varying time interval information with high sample efficiency. We propose the Time-Aware scheduler with Dyna-Style planning (TADS) approach: a sample-efficient reinforcement learning framework for realistic spaced repetition. TADS learns a Time-LSTM policy to select an optimal content according to the student's whole learning history and the time interval since the last learning event. Besides, Dyna-style planning is integrated into TADS to further improve the sample efficiency. We evaluate our approach on three environments built from synthetic data and real-world data based on well-recognized cognitive models. Empirical results demonstrate that TADS achieves superior performance against state-of-the-art algorithms.
更多查看译文
关键词
spaced repetition, human memory, reinforcement learning
AI 理解论文
溯源树
样例
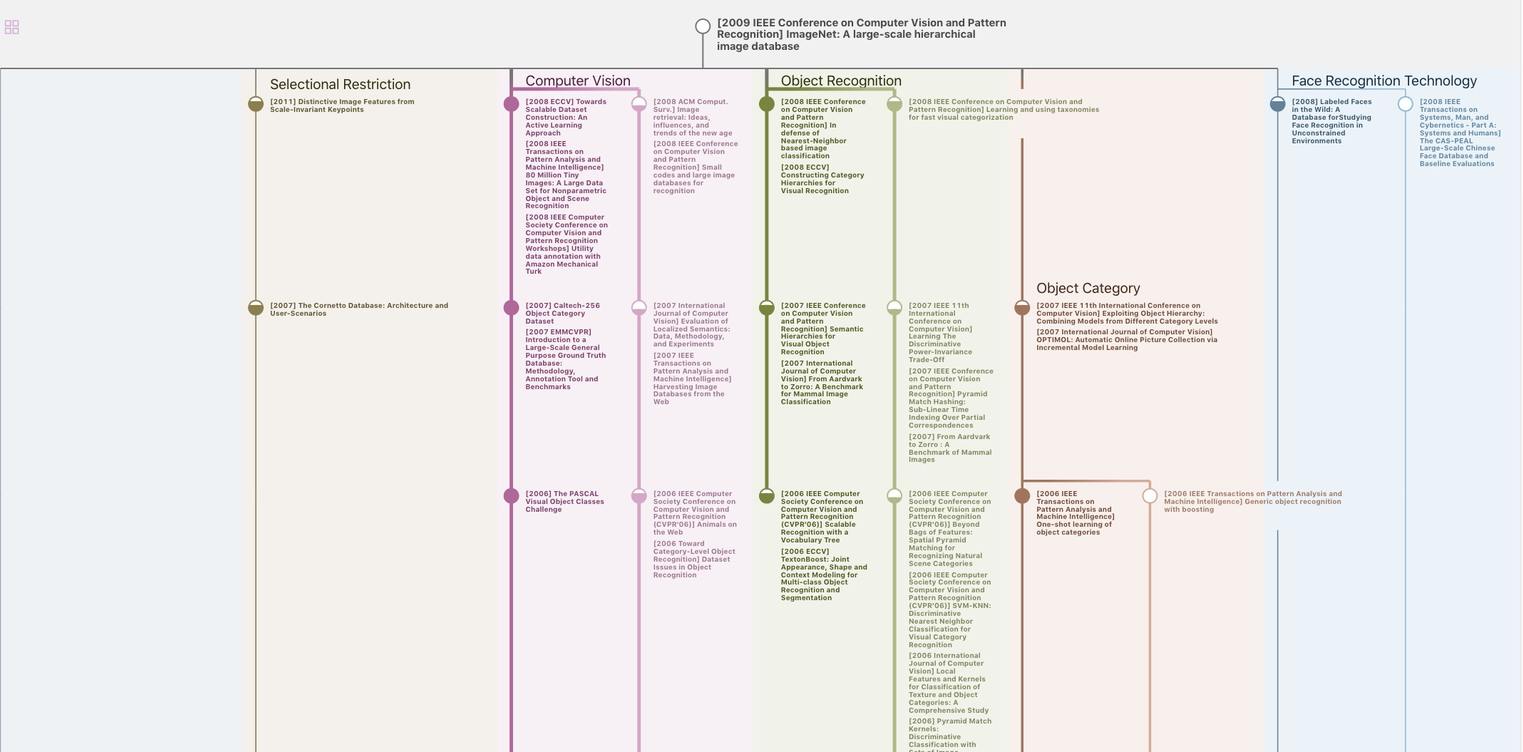
生成溯源树,研究论文发展脉络
Chat Paper
正在生成论文摘要