Neural Hierarchical Factorization Machines for User's Event Sequence Analysis
SIGIR '20: The 43rd International ACM SIGIR conference on research and development in Information Retrieval Virtual Event China July, 2020(2020)
摘要
Many prediction tasks of real-world applications need to model multi-order feature interactions in user's event sequence for better detection performance. However, existing popular solutions usually suffer two key issues: 1) only focusing on feature interactions and failing to capture the sequence influence; 2) only focusing on sequence information, but ignoring internal feature relations of each event, thus failing to extract a better event representation. In this paper, we consider a two-level structure for capturing the hierarchical information over user's event sequence: 1) learning effective feature interactions based event representation; 2) modeling the sequence representation of user's historical events. Experimental results on both industrial and public datasets clearly demonstrate that our model achieves significantly better performance compared with state-of-the-art baselines.
更多查看译文
关键词
Neural Hierarchical Factorization Machines, Event Sequence Analysis, Event Representation, Sequence Representation
AI 理解论文
溯源树
样例
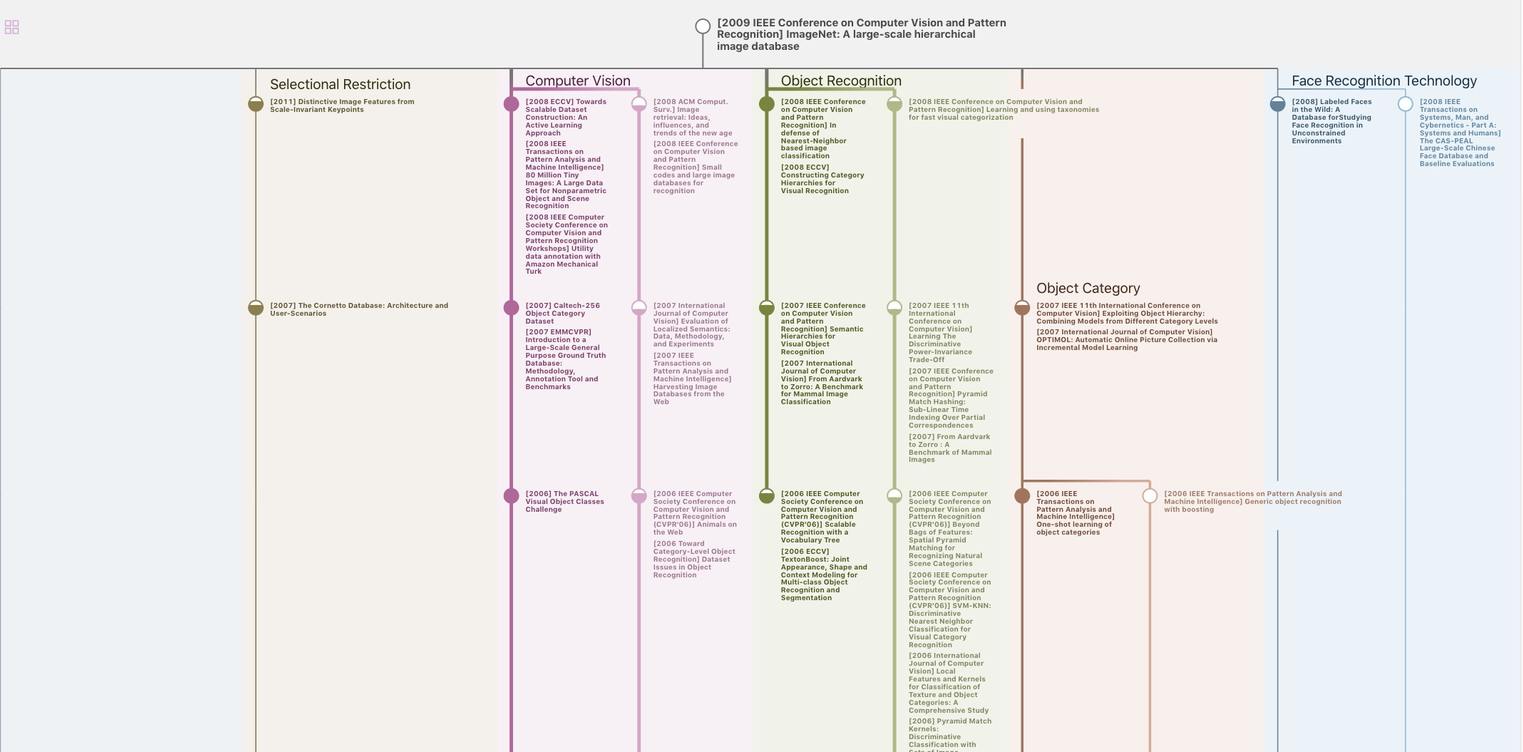
生成溯源树,研究论文发展脉络
Chat Paper
正在生成论文摘要