Multi-Level Multimodal Transformer Network for Multimodal Recipe Comprehension
SIGIR '20: The 43rd International ACM SIGIR conference on research and development in Information Retrieval Virtual Event China July, 2020(2020)
摘要
Multimodal Machine Comprehension ($\rm M^3C$) has been a challenging task that requires understanding both language and vision, as well as their integration and interaction. For example, the RecipeQA challenge, which provides several $\rm M^3C$ tasks, requires deep neural models to understand textual instructions, images of different steps, as well as the logic orders of food cooking. To address this challenge, we propose a Multi-Level Multi-Modal Transformer (MLMM-Trans) framework to integrate and understand multiple textual instructions and multiple images. Our model can conduct intensive attention mechanism at multiple levels of objects (e.g., step level and passage-image level) for sequences of different modalities. Experiments have shown that our model can achieve the state-of-the-art results on the three multimodal tasks of RecipeQA.
更多查看译文
关键词
multimodal machine reading comprehension, multimodal recipe comprehension, question answering
AI 理解论文
溯源树
样例
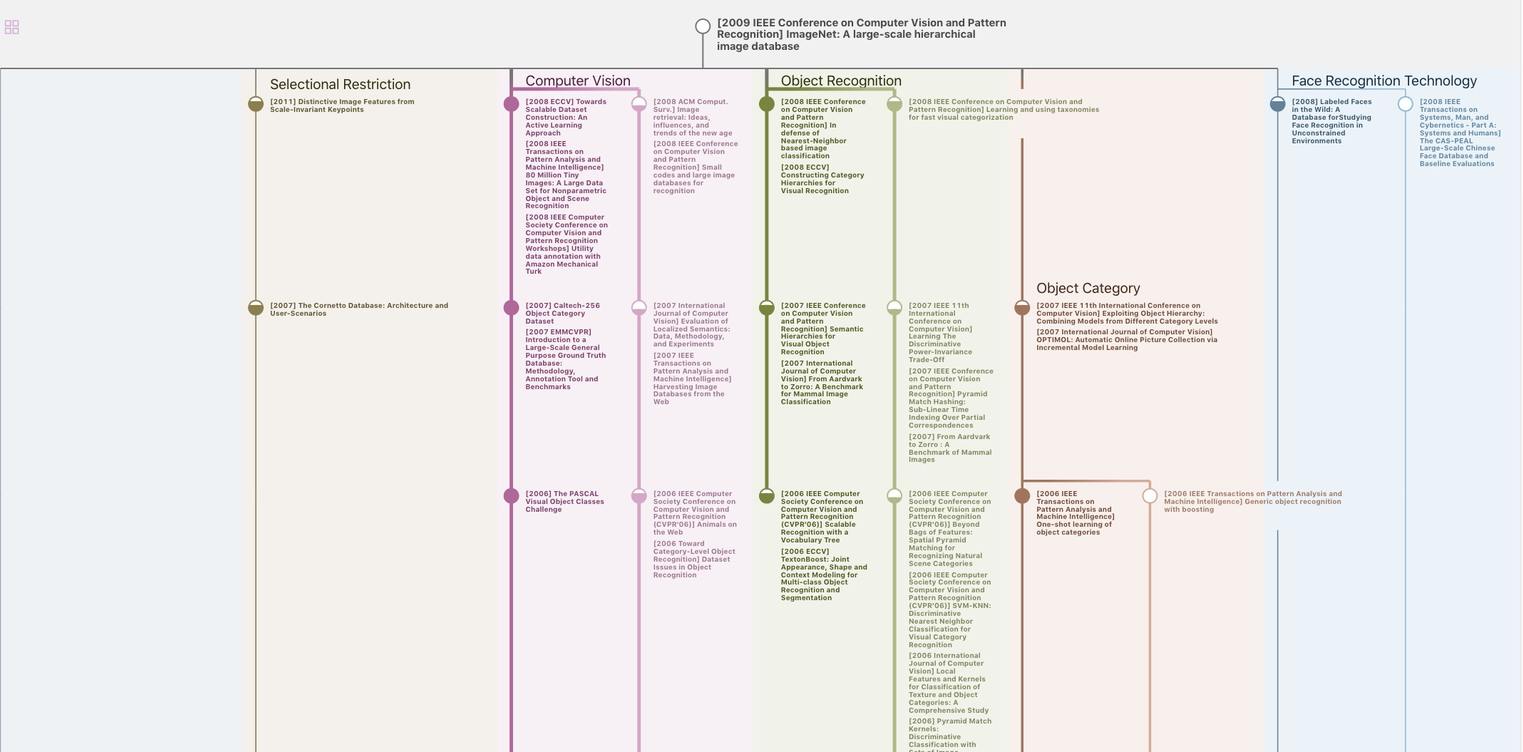
生成溯源树,研究论文发展脉络
Chat Paper
正在生成论文摘要