Generative Attribute Manipulation Scheme for Flexible Fashion Search
SIGIR '20: The 43rd International ACM SIGIR conference on research and development in Information Retrieval Virtual Event China July, 2020(2020)
摘要
In this work, we aim to investigate the practical task of flexible fashion search with attribute manipulation, where users can retrieve the target fashion items by replacing the unwanted attributes of an available query image with the desired ones (e.g., changing the collar attribute from v-neck to round). Although several pioneer efforts have been dedicated to fulfilling the task, they mainly ignore the potential of generative models in enhancing the visual understanding of target fashion items. To this end, we propose an end-to-end generative attribute manipulation scheme, which consists of a generator and a discriminator. The generator works on producing the prototype image that meets the user's requirement of attribute manipulation over the query image with the regularization of visual-semantic consistency and pixel-wise consistency. Besides, the discriminator aims to jointly fulfill the semantic learning towards correct attribute manipulation and adversarial metric learning for fashion search. Pertaining to the adversarial metric learning, we provide two general paradigms: the pair-based scheme and the triplet-based scheme, where the fake generated prototype images that closely resemble the ground truth images of target items are incorporated as hard negative samples to boost the model performance. Extensive experiments on two real-world datasets verify the effectiveness of our scheme.
更多查看译文
关键词
Fashion Search, Generative Adversarial Networks, Attribute Manipulation, Deep Metric Learning
AI 理解论文
溯源树
样例
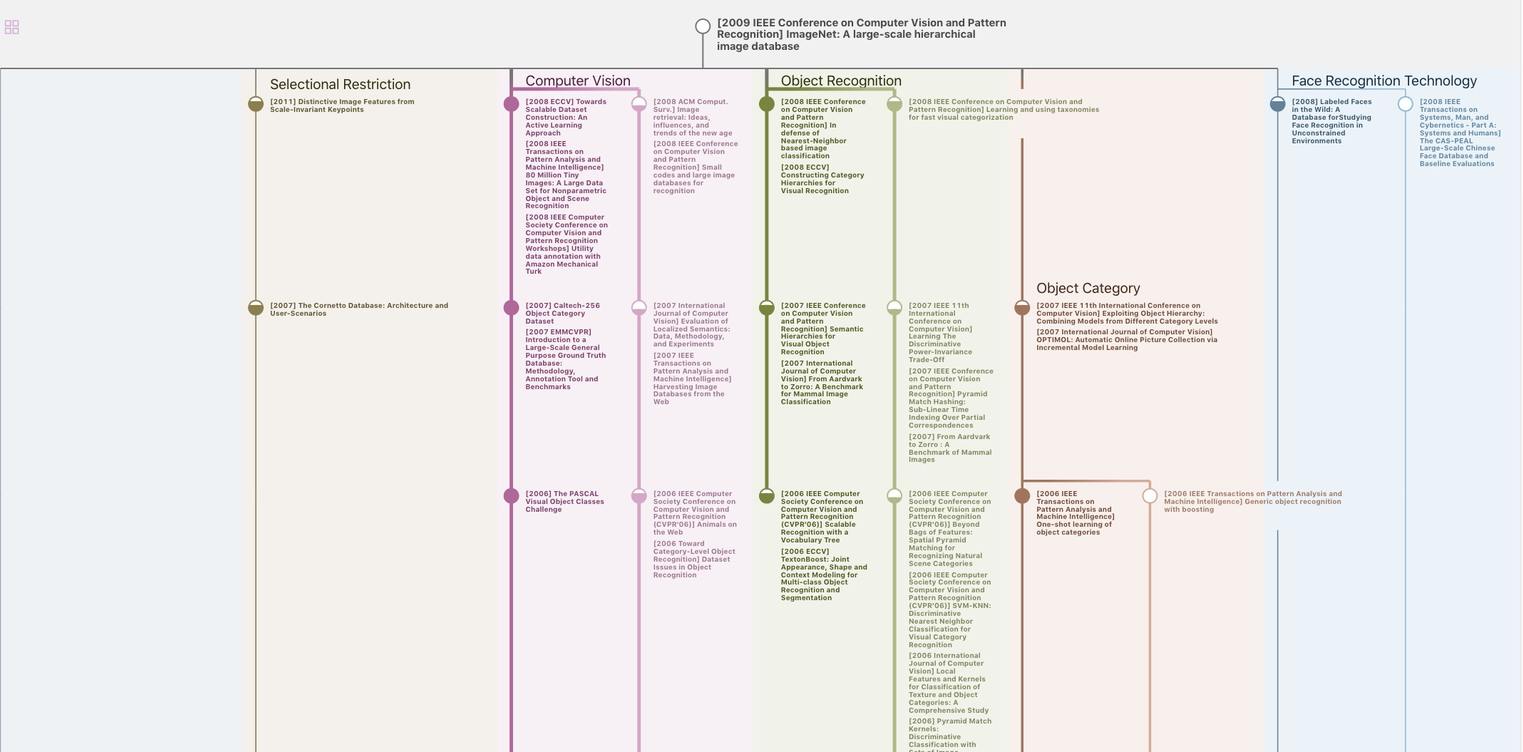
生成溯源树,研究论文发展脉络
Chat Paper
正在生成论文摘要