Sparse Deep Neural Network Optimization For Embedded Intelligence
INTERNATIONAL JOURNAL ON ARTIFICIAL INTELLIGENCE TOOLS(2020)
摘要
Deep neural networks become more popular as its ability to solve very complex pattern recognition problems. However, deep neural networks often need massive computational and memory resources, which is main reason resulting them to be difficult efficiently and entirely running on embedded platforms. This work addresses this problem by saving the computational and memory requirements of deep neural networks by proposing a variance reduced (VR)-based optimization with regularization techniques to compress the requirements of memory of models within fast training process. It is shown theoretically and experimentally that sparsity-inducing regularization can be effectively worked with the VR-based optimization whereby in the optimizer the behaviors of the stochastic element is controlled by a hyper-parameter to solve non-convex problems.
更多查看译文
关键词
First order optimization, l(1) regularization, model compression, deep neural network, embedded systems
AI 理解论文
溯源树
样例
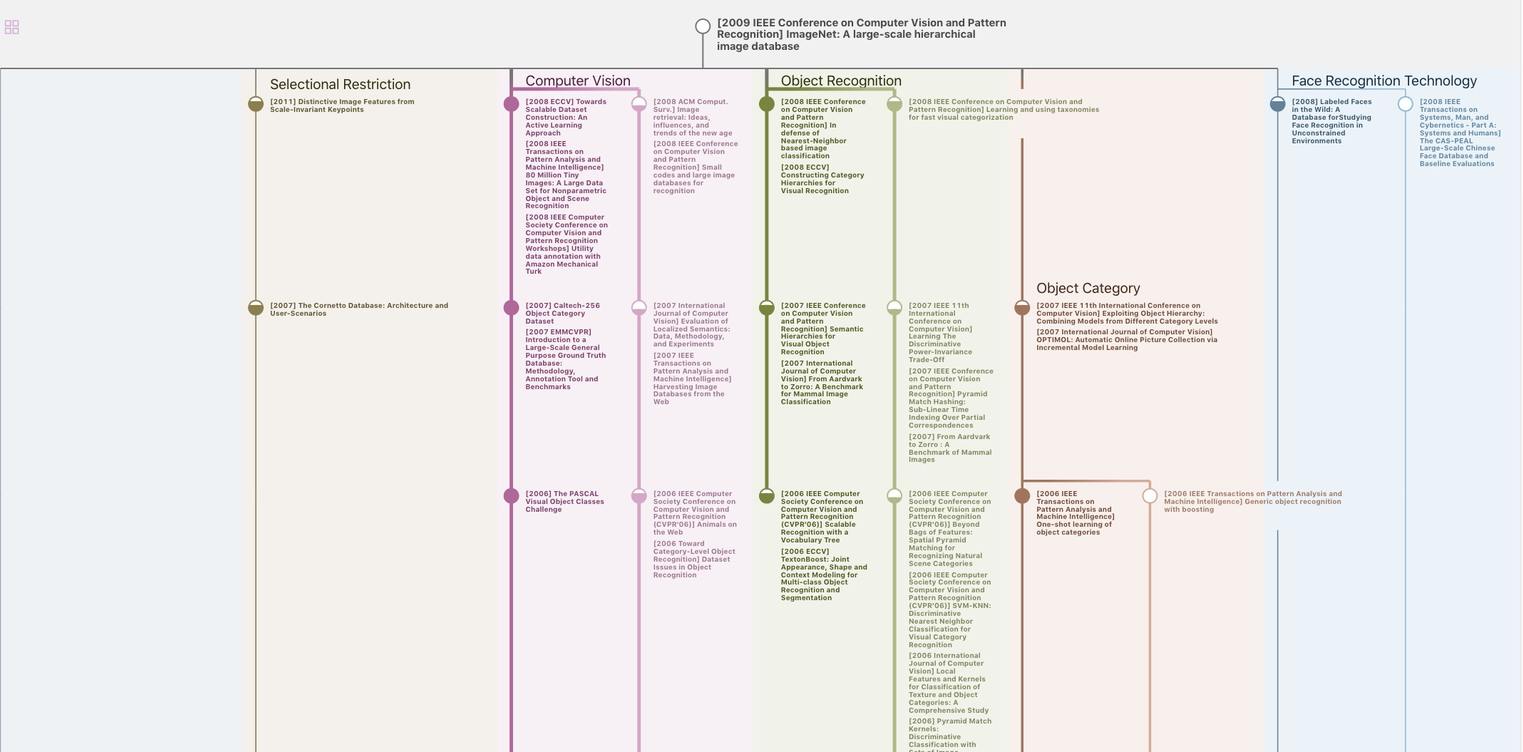
生成溯源树,研究论文发展脉络
Chat Paper
正在生成论文摘要