Feature Selection Methods for Household Food Insecurity Classification
2020 International Conference on Computer Science, Engineering and Applications (ICCSEA)(2020)
摘要
Predicting food insecurity plays a vital role in addressing the household’s problem to the respective bodies whose responsibility is to take action on prevention and intervention actions. In filter-based feature selection, features are selected based on their score values in various statistical tests for their correlation with the target variable. In this paper, filter-based feature selection techniques are explored, such as correlation matrix, feature importance, univariate, and the proposed feature selection method called intersection. Algorithms like K-Nearest Neighbour (KNN), Random Forest (RF), Logistic Regression (LR), and Support Vector Machine (SVM) are used to evaluate the accuracy of selected features. The experimental result shows that Random forest is the best classification algorithm, and feature importance is the best feature selection method with seven features which scores 99.98% accuracy and 100% ROC.
更多查看译文
关键词
Calorie,Food insecurity,Machine learning,Classification,Feature selection,HICE
AI 理解论文
溯源树
样例
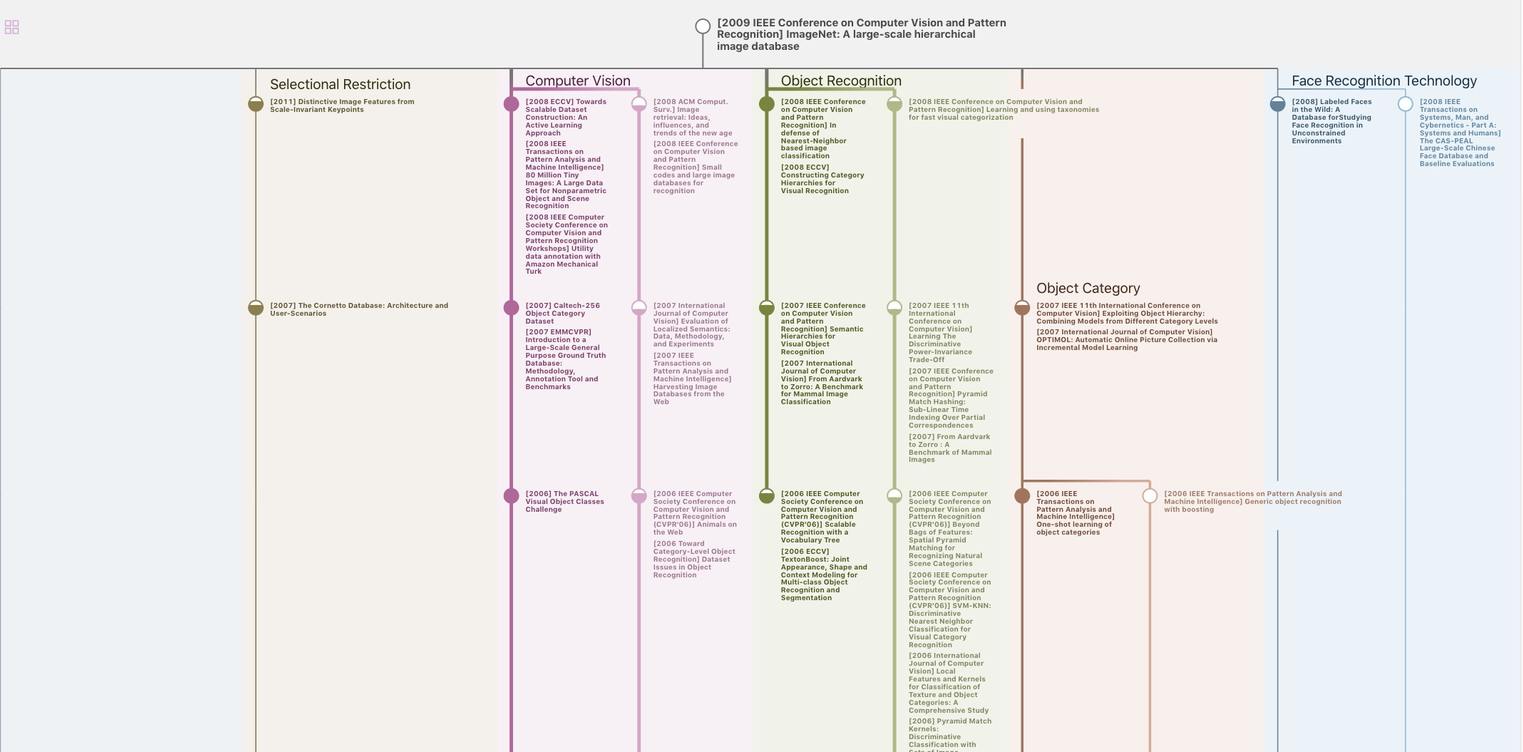
生成溯源树,研究论文发展脉络
Chat Paper
正在生成论文摘要