Mec-Enabled Wireless Vr Video Service: A Learning-Based Mixed Strategy For Energy-Latency Tradeoff
2020 IEEE WIRELESS COMMUNICATIONS AND NETWORKING CONFERENCE (WCNC)(2020)
摘要
Mobile edge computing (MEC) has received broad attention as an effective network architecture and a key enabler of the wireless virtual reality (VR) video service which is expected to take a huge share of communication traffic. In this work, we investigate the scenario of multi-tiles-based wireless VR video service with the aid of MEC network, where the primary objective is to minimize the system energy consumption and the latency as well as to arrive at a tradeoff between these two metrics. To this end, we first cast the time-varying view popularity as a modelfree Markov chain and use a long short-term memory auto-encoder network to predict its dynamics. Then, a mixed strategy, which jointly considers the dynamic caching replacement and the deterministic offloading, is designed to fully utilize the caching and computing resource in the system. The underlying multiobjective optimization problem is reformulated as a partially observable Markov decision process and solved by using a deep deterministic policy gradient algorithm. The effectiveness of the proposed scheme is confirmed by numerical simulations.
更多查看译文
关键词
MEC-enabled wireless VR video service,learning-based mixed strategy,energy-latency tradeoff,mobile edge computing,network architecture,wireless virtual reality video service,communication traffic,MEC network,system energy consumption,time-varying view popularity,long short-term memory autoencoder network,dynamic caching replacement,computing resource,model-free Markov chain,multitiles-based wireless VR video service,deterministic offloading,multiobjective optimization problem,partially observable Markov decision process,deep deterministic policy gradient algorithm
AI 理解论文
溯源树
样例
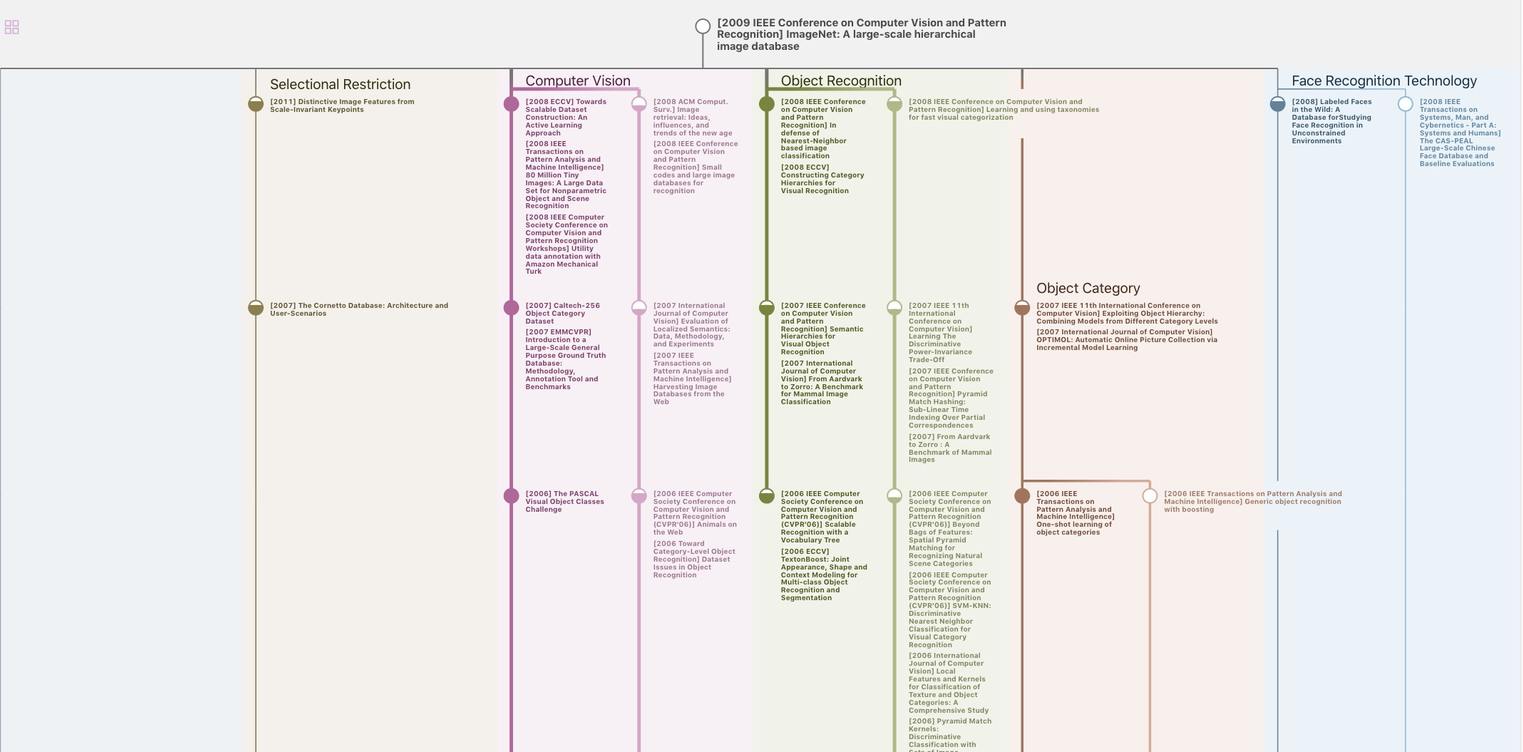
生成溯源树,研究论文发展脉络
Chat Paper
正在生成论文摘要