Auction Based Incentive Design For Efficient Federated Learning In Cellular Wireless Networks
2020 IEEE WIRELESS COMMUNICATIONS AND NETWORKING CONFERENCE (WCNC)(2020)
摘要
Federated learning is an prominent machine learning technique that model is trained distributively by using local data of mobile users, which can preserve the privacy of users and still guarantee high learning performance. In this paper, we deal with the problem of incentive mechanism design for motivating users to participate in training. In this paper, we employ the randomized auction framework for incentive mechanism design in which the base station is a seller and mobile users are buyers. Concerning the energy cost incurred due to join the training, the users need to decide how many uplink subchannels, transmission power and CPU cycle frequency and then claim them in submitted bids to the base station. After receiving the submitted bids, the base station needs algorithms to select winners and determine the corresponding rewards so that the social cost is minimized. The proposed mechanism can guarantee three economic properties, i.e., truthfulness, individual rationality and efficiency. Finally, numerical results are provided to demonstrate the effectiveness, and efficiency of our scheme.
更多查看译文
关键词
incentive design,efficient federated learning,cellular wireless networks,prominent machine learning technique,mobile users,high learning performance,incentive mechanism design,motivating users,randomized auction framework,base station,submitted bids
AI 理解论文
溯源树
样例
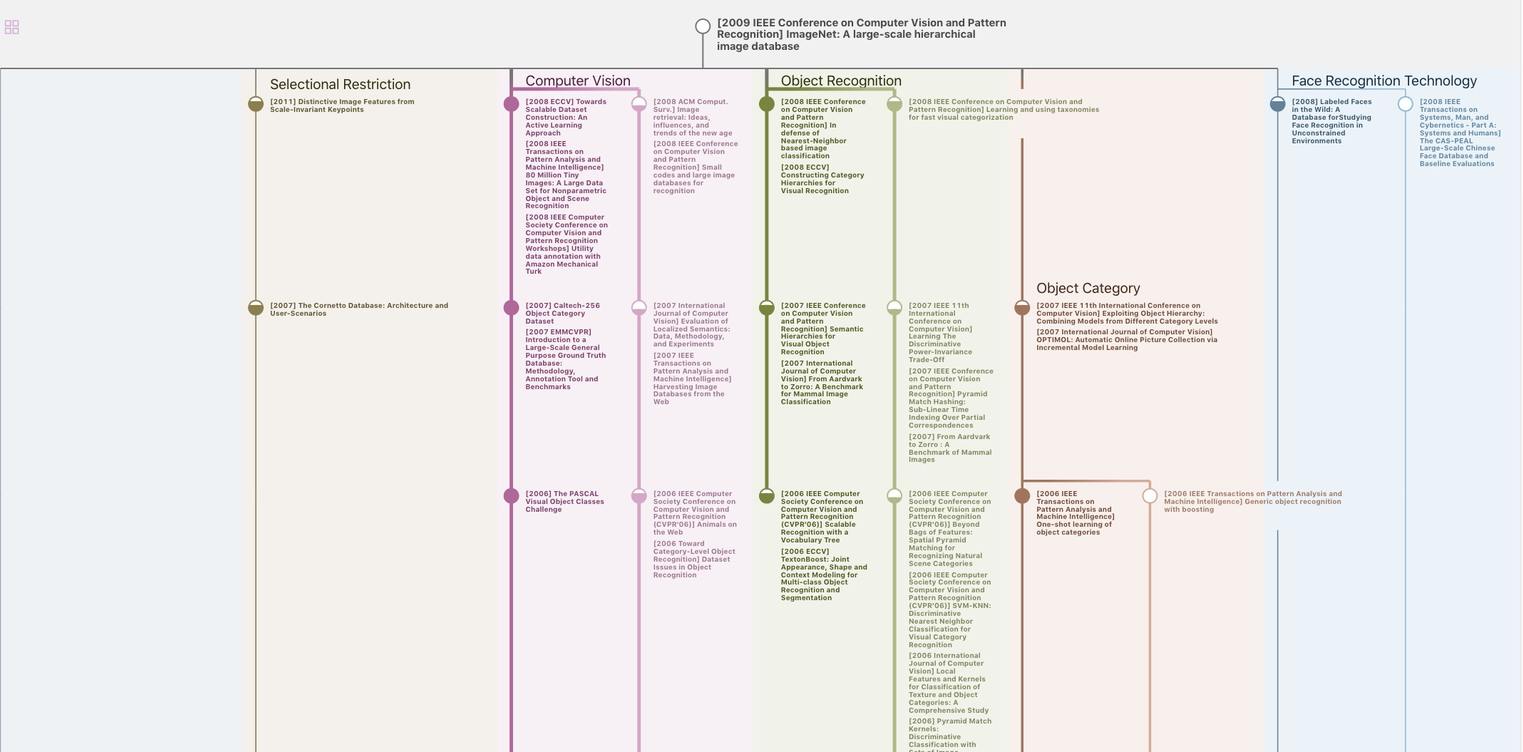
生成溯源树,研究论文发展脉络
Chat Paper
正在生成论文摘要