Discriminate-and-Rectify Encoders: Learning from Image Transformation Sets
arXiv (Cornell University)(2017)
摘要
The complexity of a learning task is increased by transformations in the input space that preserve class identity. Visual object recognition for example is affected by changes in viewpoint, scale, illumination or planar transformations. While drastically altering the visual appearance, these changes are orthogonal to recognition and should not be reflected in the representation or feature encoding used for learning. We introduce a framework for weakly supervised learning of image embeddings that are robust to transformations and selective to the class distribution, using sets of transforming examples (orbit sets), deep parametrizations and a novel orbit-based loss. The proposed loss combines a discriminative, contrastive part for orbits with a reconstruction error that learns to rectify orbit transformations. The learned embeddings are evaluated in distance metric-based tasks, such as one-shot classification under geometric transformations, as well as face verification and retrieval under more realistic visual variability. Our results suggest that orbit sets, suitably computed or observed, can be used for efficient, weakly-supervised learning of semantically relevant image embeddings.
更多查看译文
关键词
encoders,transformation,learning,sets,image,discriminate-and-rectify
AI 理解论文
溯源树
样例
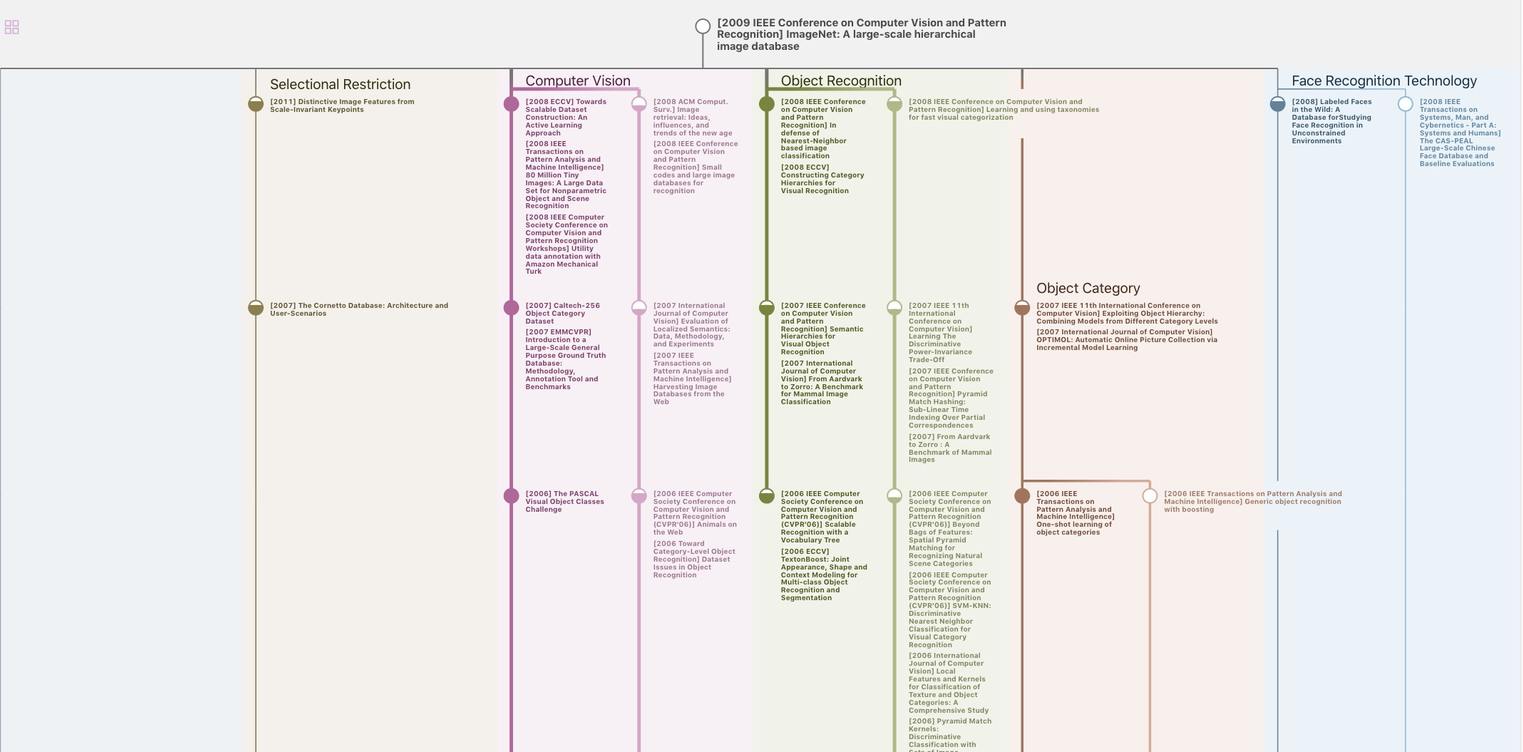
生成溯源树,研究论文发展脉络
Chat Paper
正在生成论文摘要