Robust Inverse Reinforcement Learning under Transition Dynamics Mismatch
Annual Conference on Neural Information Processing Systems(2021)
摘要
We study the inverse reinforcement learning (IRL) problem under a transition dynamics mismatch between the expert and the learner. Specifically, we consider the Maximum Causal Entropy (MCE) IRL learner model and provide a tight upper bound on the learner's performance degradation based on the l(1)-distance between the transition dynamics of the expert and the learner. Leveraging insights from the Robust RL literature, we propose a robust MCE IRL algorithm, which is a principled approach to help with this mismatch. Finally, we empirically demonstrate the stable performance of our algorithm compared to the standard MCE IRL algorithm under transition dynamics mismatches in both finite and continuous MDP problems.
更多查看译文
关键词
robust inverse reinforcement learning,reinforcement learning,transition dynamics mismatch
AI 理解论文
溯源树
样例
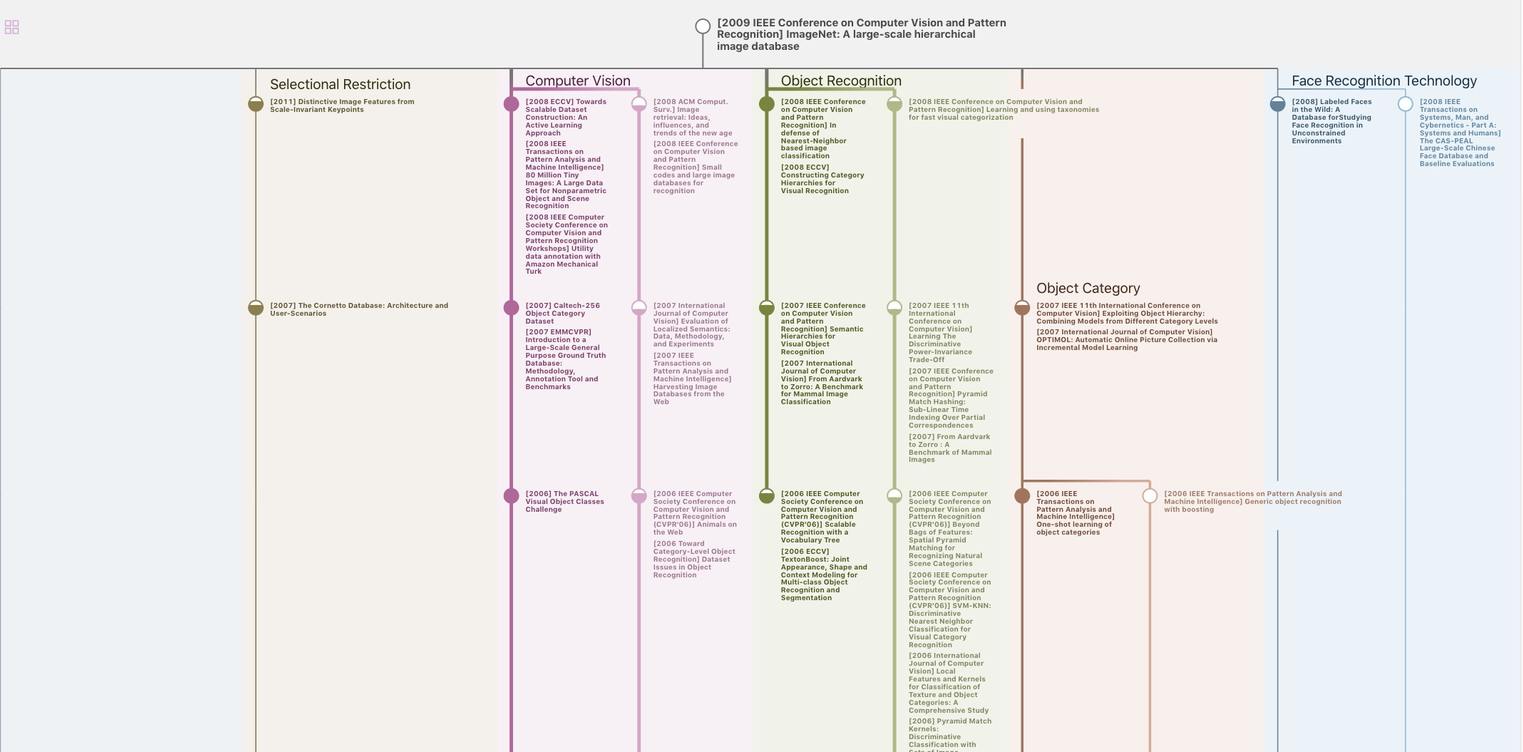
生成溯源树,研究论文发展脉络
Chat Paper
正在生成论文摘要