Graph Feature Networks
ICLR'19 RLGM workshop(2019)
摘要
Graph Neural Nets (GNNs) have received increasing attentions, partially due to their superior performance in many node and graph classification tasks. However, there is a lack of understanding on what they are learning and how sophisticated the learned graph functions are. In this work, we propose Graph Feature Network (GFN), a simple lightweight neural net defined on a set of graph augmented features. GFN can be used to approximate GNNs with improved efficiency, and serve as a tool to access and understand the graph functions learned. To our surprise, we find that, despite its simplicity, GFN could match or exceed the best accuracies produced by recently proposed GNNs on common graph classification benchmarks. Our results seemingly suggest that (1) GFN may serve as an efficient and effective alternative to GNNs, and (2) existing GNNs may not have learned more sophisticated graph functions on these benchmarks. Furthermore, we observe that when treating images as pixels defined in graphs/grids, the same type of GNNs can outperform GFN, indicating GNNs may have learned more complex graph functions in that case.1
更多查看译文
AI 理解论文
溯源树
样例
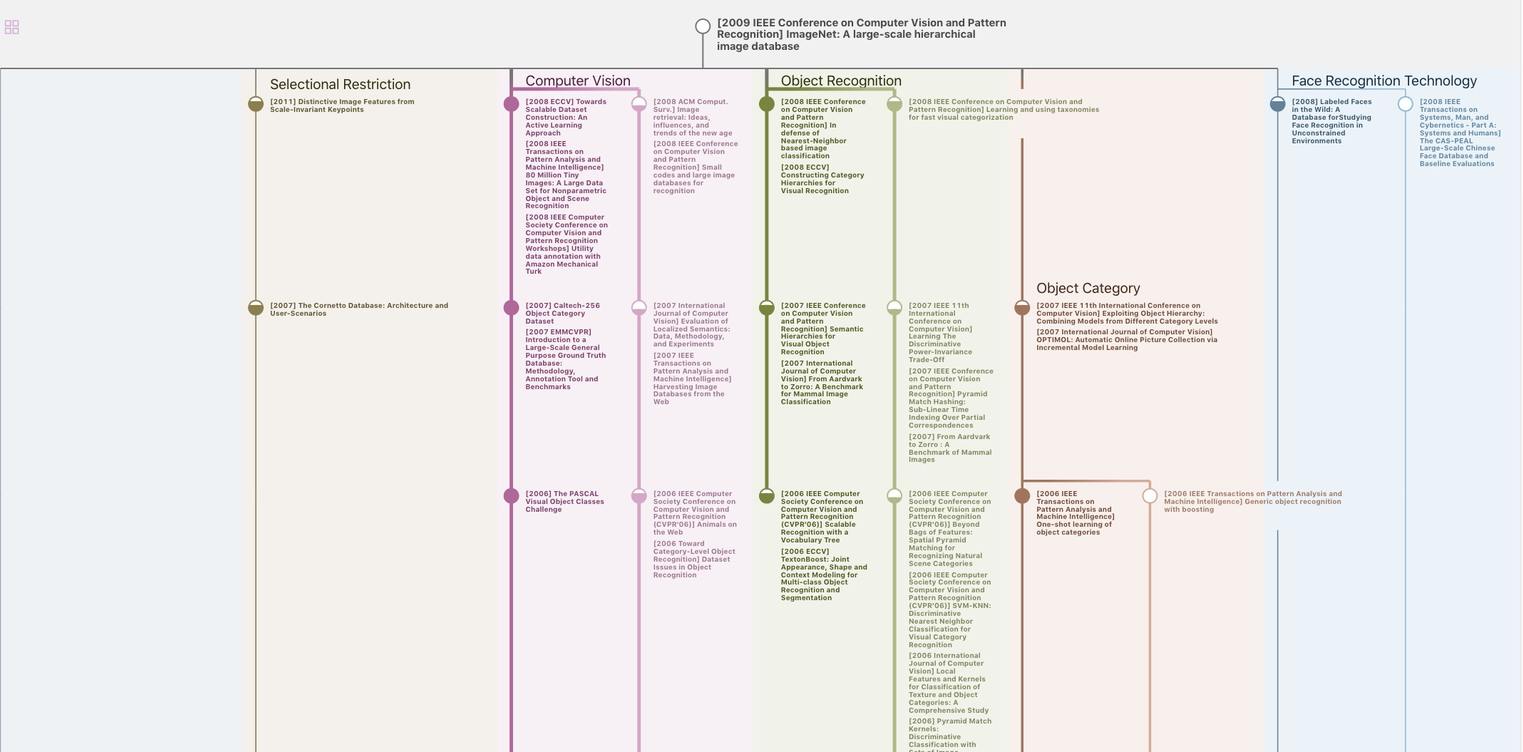
生成溯源树,研究论文发展脉络
Chat Paper
正在生成论文摘要