Variational Policy Propagation for Multi-agent Reinforcement Learning
arxiv(2020)
摘要
We propose a collaborative multi-agent reinforcement learning algorithm named variational policy propagation (VPP) to learn a joint policy through the interactions over agents. We prove that the joint policy is a Markov Random Field under some mild conditions, which in turn reduces the policy space effectively. We integrate the variational inference as special differentiable layers in policy such that the actions can be efficiently sampled from the Markov Random Field and the overall policy is differentiable. We evaluate our algorithm on several large scale challenging tasks and demonstrate that it outperforms previous state-of-the-arts.
更多查看译文
关键词
variational policy propagation,learning,multi-agent
AI 理解论文
溯源树
样例
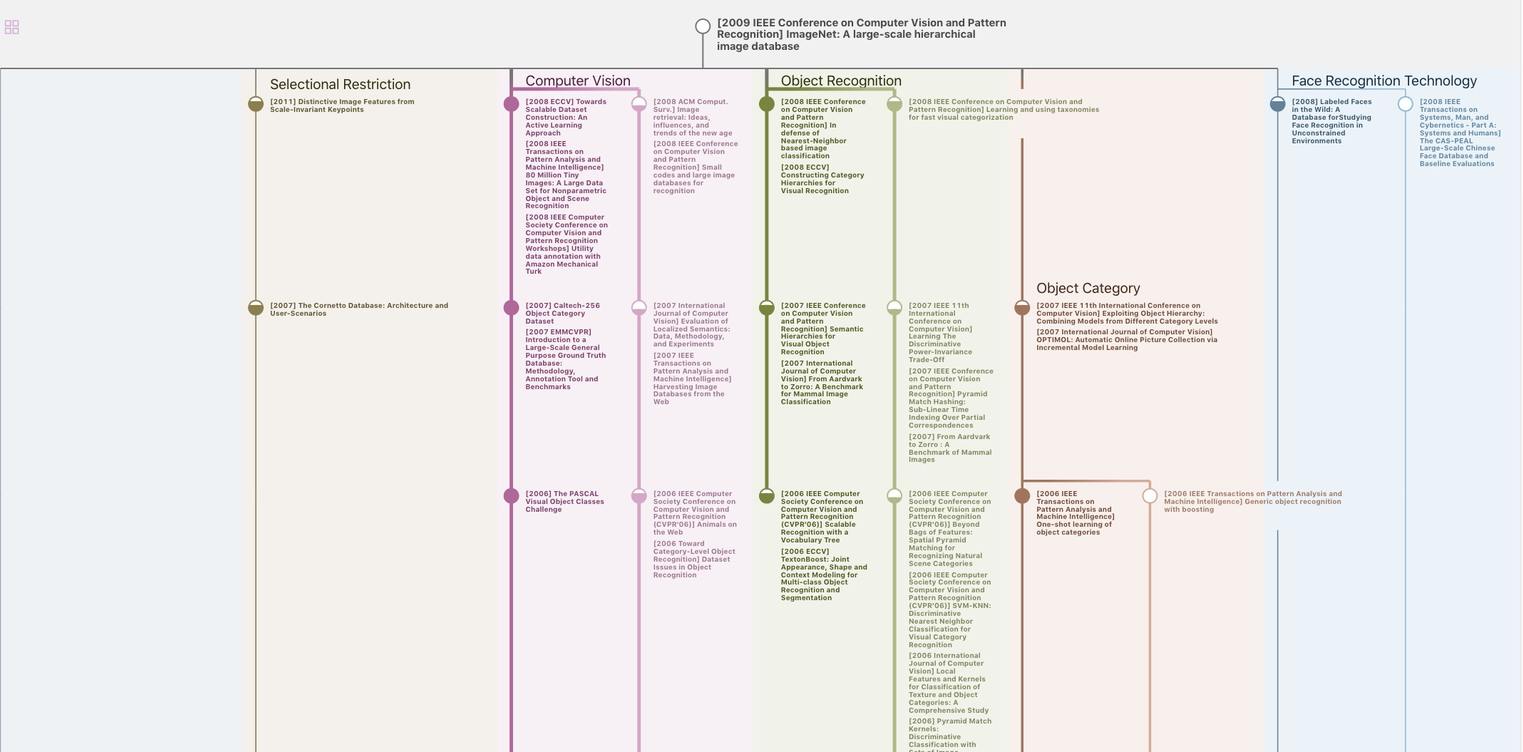
生成溯源树,研究论文发展脉络
Chat Paper
正在生成论文摘要