Knowledge-Aware Language Model Pretraining
arxiv(2020)
摘要
How much knowledge do pretrained language models hold? Recent research observed that pretrained transformers are adept at modeling semantics but it is unclear to what degree they grasp human knowledge, or how to ensure they do so. In this paper we incorporate knowledge-awareness in language model pretraining without changing the transformer architecture, inserting explicit knowledge layers, or adding external storage of semantic information. Rather, we simply signal the existence of entities to the input of the transformer in pretraining, with an entity-extended tokenizer; and at the output, with an additional entity prediction task. Our experiments show that solely by adding these entity signals in pretraining, significantly more knowledge is packed into the transformer parameters: we observe improved language modeling accuracy, factual correctness in LAMA knowledge probing tasks, and semantics in the hidden representations through edge probing.We also show that our knowledge-aware language model (KALM) can serve as a drop-in replacement for GPT-2 models, significantly improving downstream tasks like zero-shot question-answering with no task-related training.
更多查看译文
关键词
language,model,knowledge-aware
AI 理解论文
溯源树
样例
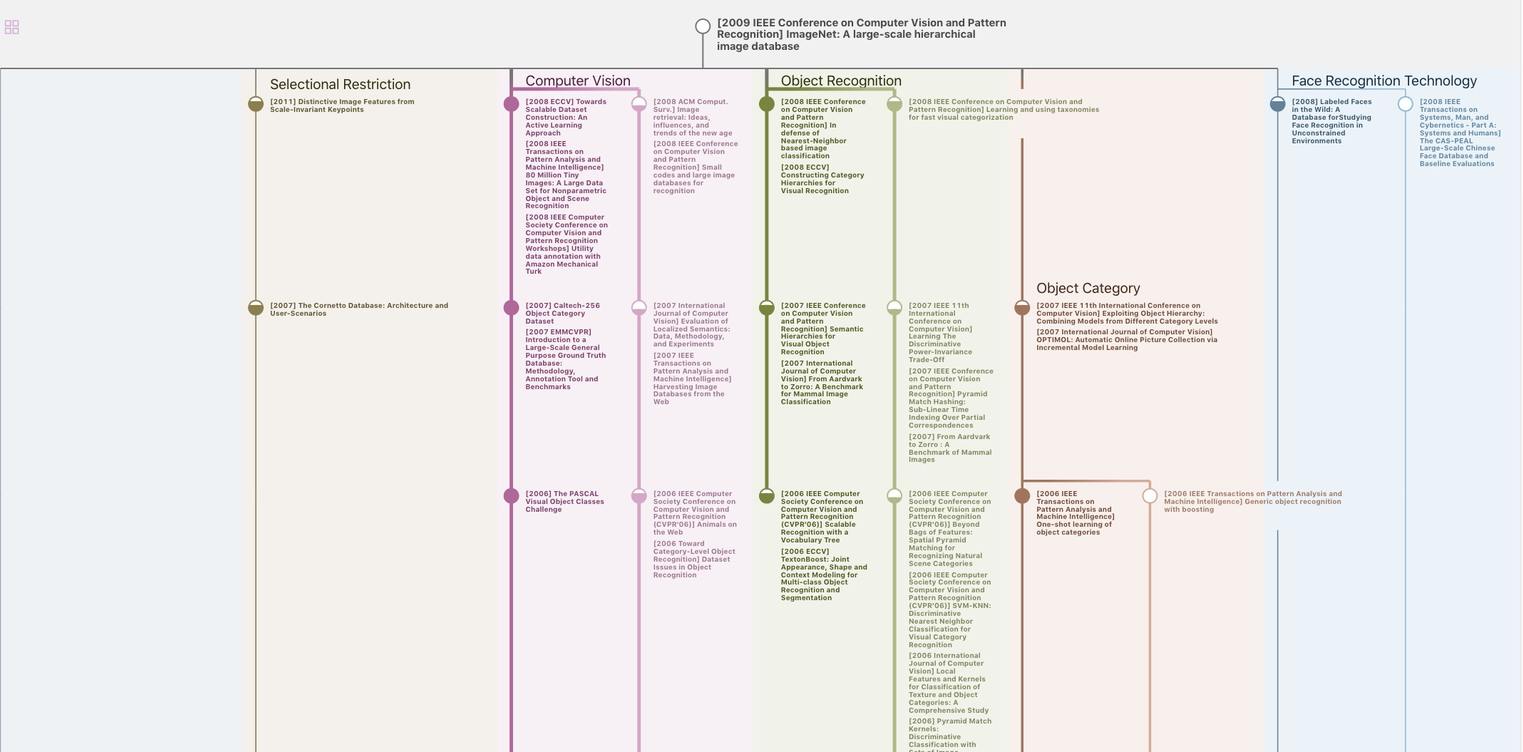
生成溯源树,研究论文发展脉络
Chat Paper
正在生成论文摘要