A New Basis for Sparse Principal Component Analysis
JOURNAL OF COMPUTATIONAL AND GRAPHICAL STATISTICS(2023)
摘要
Previous versions of sparse principal component analysis (PCA) have presumed that the eigen-basis (a p x k matrix) is approximately sparse. We propose a method that presumes the p x k matrix becomes approximately sparse after a k x k rotation. The simplest version of the algorithm initializes with the leading k principal components. Then, the principal components are rotated with an k x k orthogonal rotation to make them approximately sparse. Finally, soft-thresholding is applied to the rotated principal components. This approach differs from prior approaches because it uses an orthogonal rotation to approximate a sparse basis. One consequence is that a sparse component need not to be a leading eigenvector, but rather a mixture of them. In this way, we propose a new (rotated) basis for sparse PCA. In addition, our approach avoids "deflation" and multiple tuning parameters required for that. Our sparse PCA framework is versatile; for example, it extends naturally to a two-way analysis of a data matrix for simultaneous dimensionality reduction of rows and columns. We provide evidence showing that for the same level of sparsity, the proposed sparse PCA method is more stable and can explain more variance compared to alternative methods. Through three applications-sparse coding of images, analysis of transcriptome sequencing data, and large-scale clustering of social networks, we demonstrate the modern usefulness of sparse PCA in exploring multivariate data. An R package, epca, and the supplementary materials for this article are available online.
更多查看译文
关键词
Column sparsity,Dimensionality reduction,Independent component analysis,Orthogonal rotation,Sparse matrix decomposition
AI 理解论文
溯源树
样例
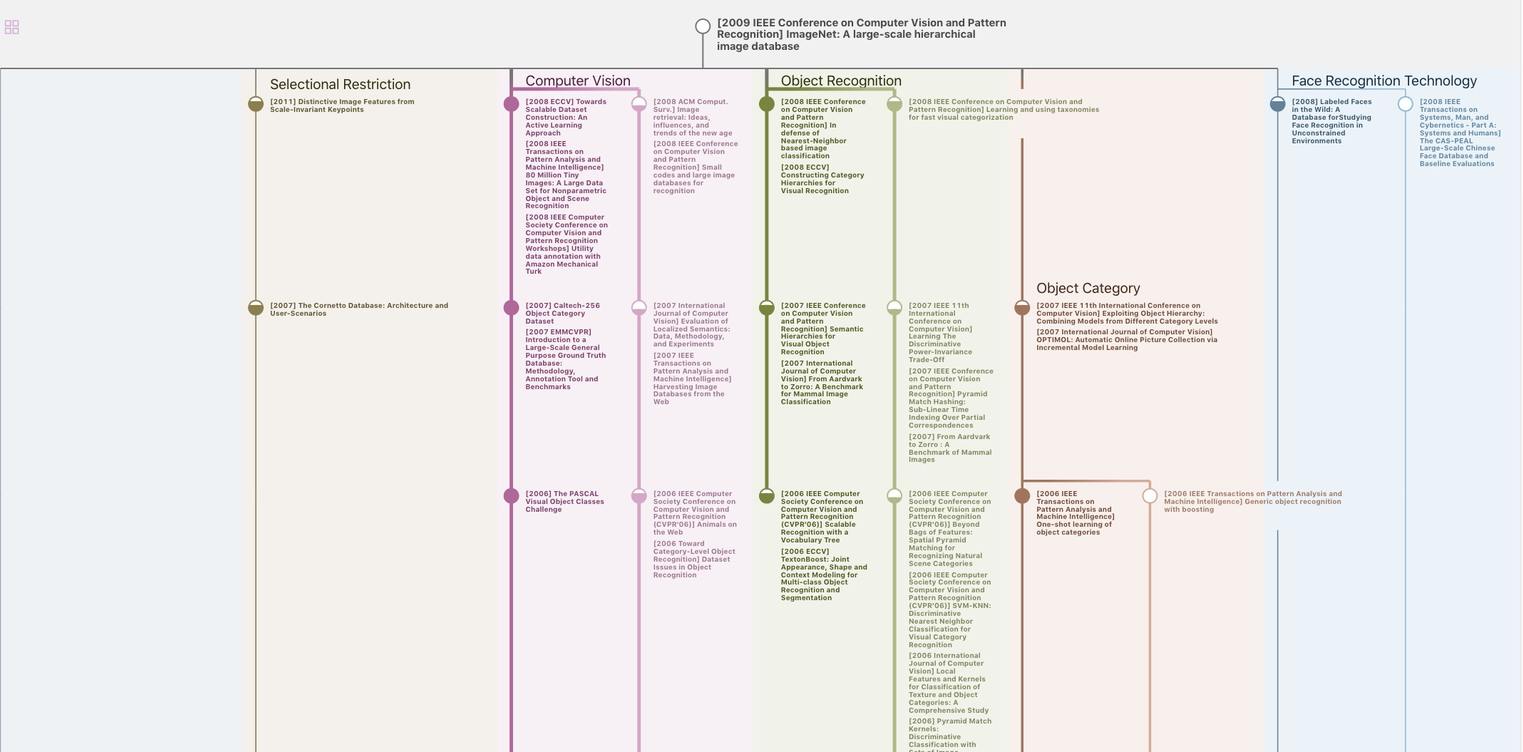
生成溯源树,研究论文发展脉络
Chat Paper
正在生成论文摘要