Predicting Healthcare-Associated Infections at Admission: Implications for Nursing Care.
NURSING RESEARCH(2020)
摘要
Background Accurate, real-time models to predict hospital adverse events could facilitate timely and targeted interventions to improve patient outcomes. Advances in computing enable the use of supervised machine learning (SML) techniques to predict hospital-onset infections. Objectives The purpose of this study was to trial SML methods to predict urinary tract infections (UTIs) during inpatient hospitalization at the time of admission. Methods In a large cohort of adult hospitalizations in three New York City acute care facilities (N= 897,344), we used two SML methods-neural networks and decision trees-to predict having a hospital-onset UTI using data available and accessible on the first day of admission at healthcare facilities in the United States. Results Performance for both neural network and decision tree models were superior compared to logistic regression methods. The decision tree model had a higher sensitivity compared to neural network, but a lower specificity. Discussion SML methods show potential for automated accurate UTI risk stratification using electronic data routinely available at admission; this could relieve nurses from the burden of having to complete and document additional risk assessment forms in the electronic medical record. Future studies should pilot and test interventions linked to the risk stratification results, such as short nursing educational modules or alerts triggered for high-risk patients.
更多查看译文
关键词
healthcare-associated infections,machine learning,urinary tract infections
AI 理解论文
溯源树
样例
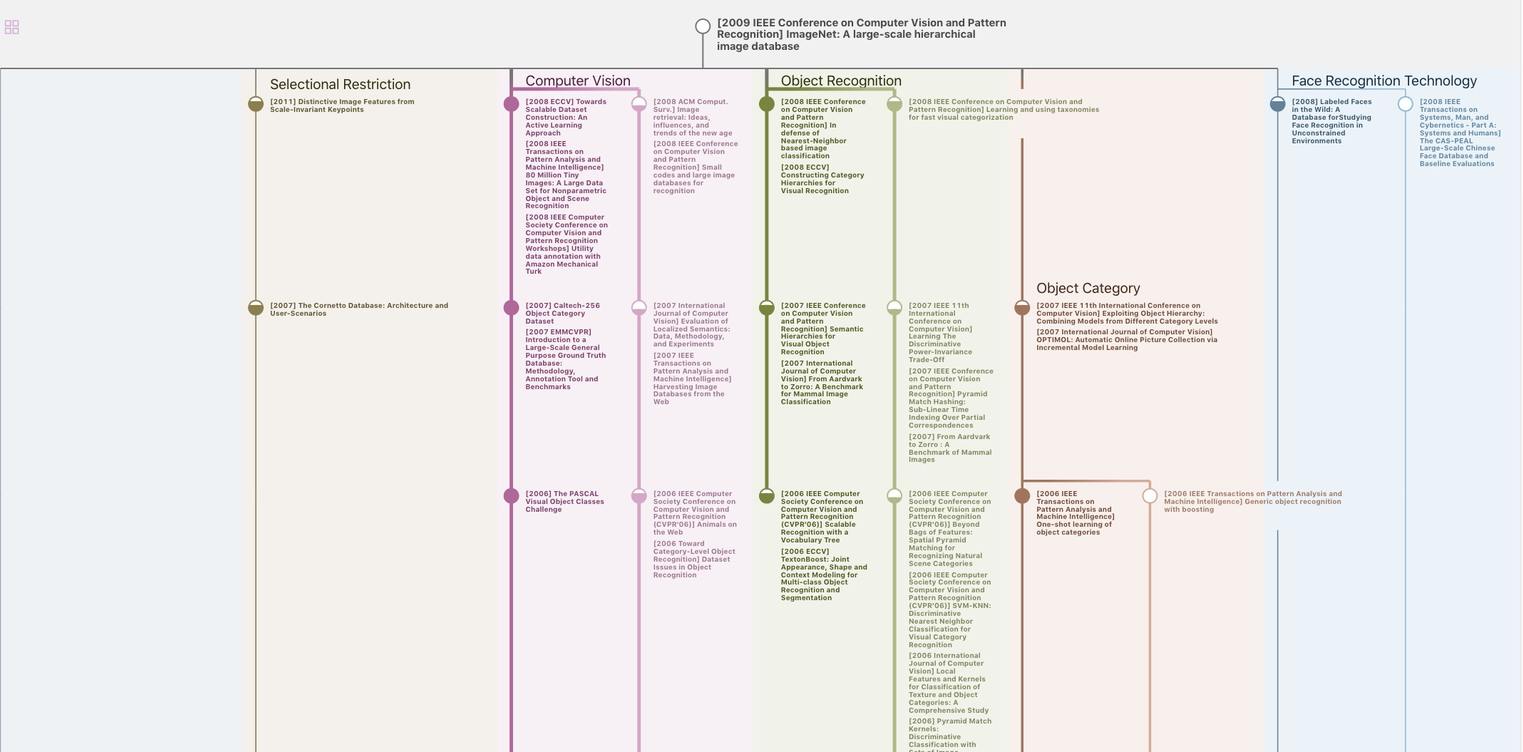
生成溯源树,研究论文发展脉络
Chat Paper
正在生成论文摘要