Tomographic Auto-Encoder: Unsupervised Bayesian Recovery of Corrupted Data
ICLR(2020)
摘要
We propose a new probabilistic method for unsupervised recovery of corrupted data. Given a large ensemble of degraded samples, our method recovers accurate posteriors of clean values, allowing the exploration of the manifold of possible reconstructed data and hence characterising the underlying uncertainty. In this setting, direct application of classical variational methods often gives rise to collapsed densities that do not adequately explore the solution space. Instead, we derive our novel reduced entropy condition approximate inference method that results in rich posteriors. We test our model in a data recovery task under the common setting of missing values and noise, demonstrating superior performance to existing variational methods for imputation and de-noising with different real data sets. We further show higher classification accuracy after imputation, proving the advantage of propagating uncertainty to downstream tasks with our model.
更多查看译文
关键词
unsupervised bayesian recovery,data,auto-encoder
AI 理解论文
溯源树
样例
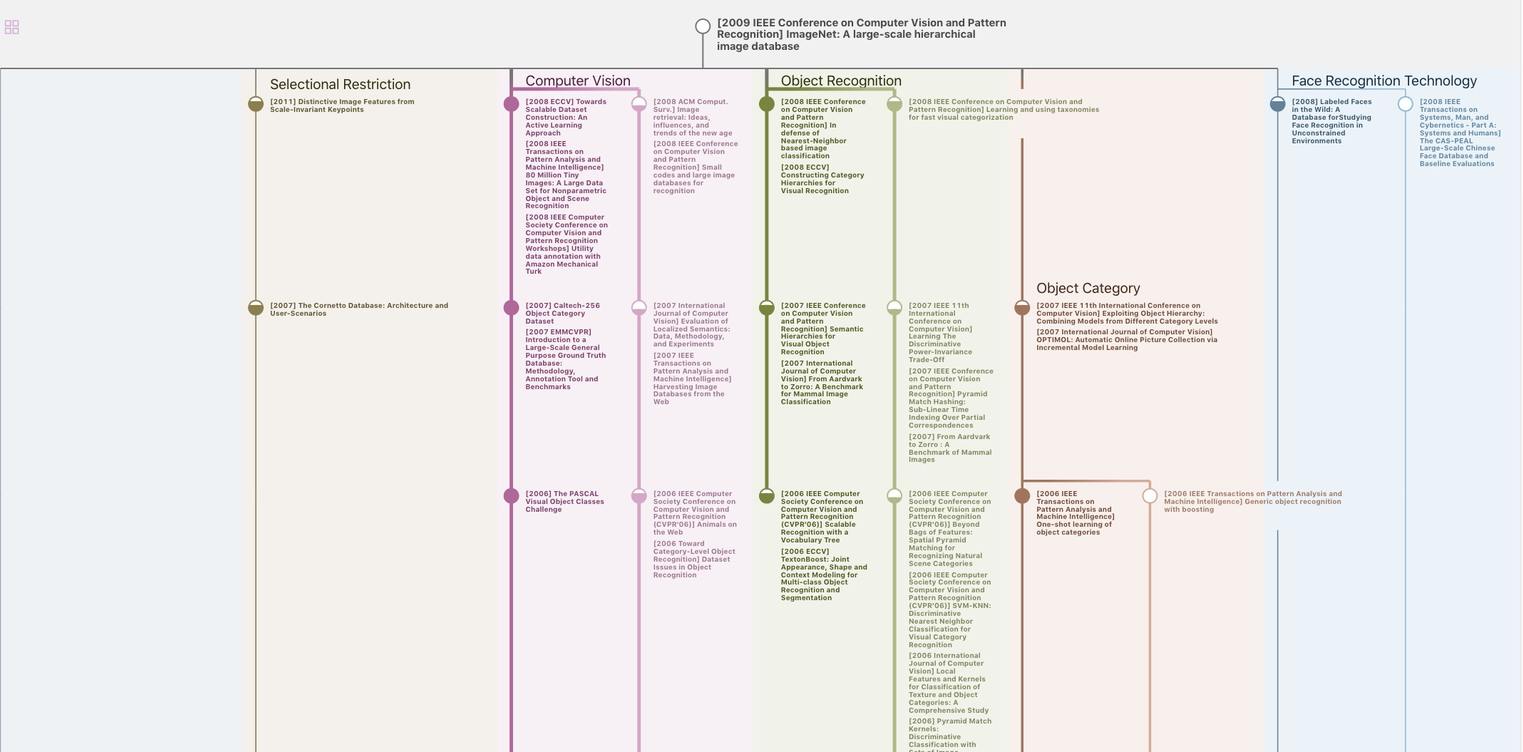
生成溯源树,研究论文发展脉络
Chat Paper
正在生成论文摘要