Path integral based convolution and pooling for graph neural networks*
JOURNAL OF STATISTICAL MECHANICS-THEORY AND EXPERIMENT(2020)
摘要
Graph neural networks (GNNs) extend the functionality of traditional neural networks to graph-structured data. Similar to CNNs, an optimized design of graph convolution and pooling is key to success. Borrowing ideas from physics, we propose path integral-based GNNs (PAN) for classification and regression tasks on graphs. Specifically, we consider a convolution operation that involves every path linking the message sender and receiver with learnable weights depending on the path length, which corresponds to the maximal entropy random walk. It generalizes the graph Laplacian to a new transition matrix that we call the maximal entropy transition (MET) matrix derived from a path integral formalism. Importantly, the diagonal entries of the MET matrix are directly related to the subgraph centrality, thus leading to a natural and adaptive pooling mechanism. PAN provides a versatile framework that can be tailored for different graph data with varying sizes and structures. We can view most existing GNN architectures as special cases of PAN. Experimental results show that PAN achieves state-of-the-art performance on various graph classification/regression tasks, including a new benchmark dataset from statistical mechanics that we propose to boost applications of GNN in physical sciences.
更多查看译文
关键词
deep learning, inference of graphical models, message-passing algorithms, random, ordered microstructures
AI 理解论文
溯源树
样例
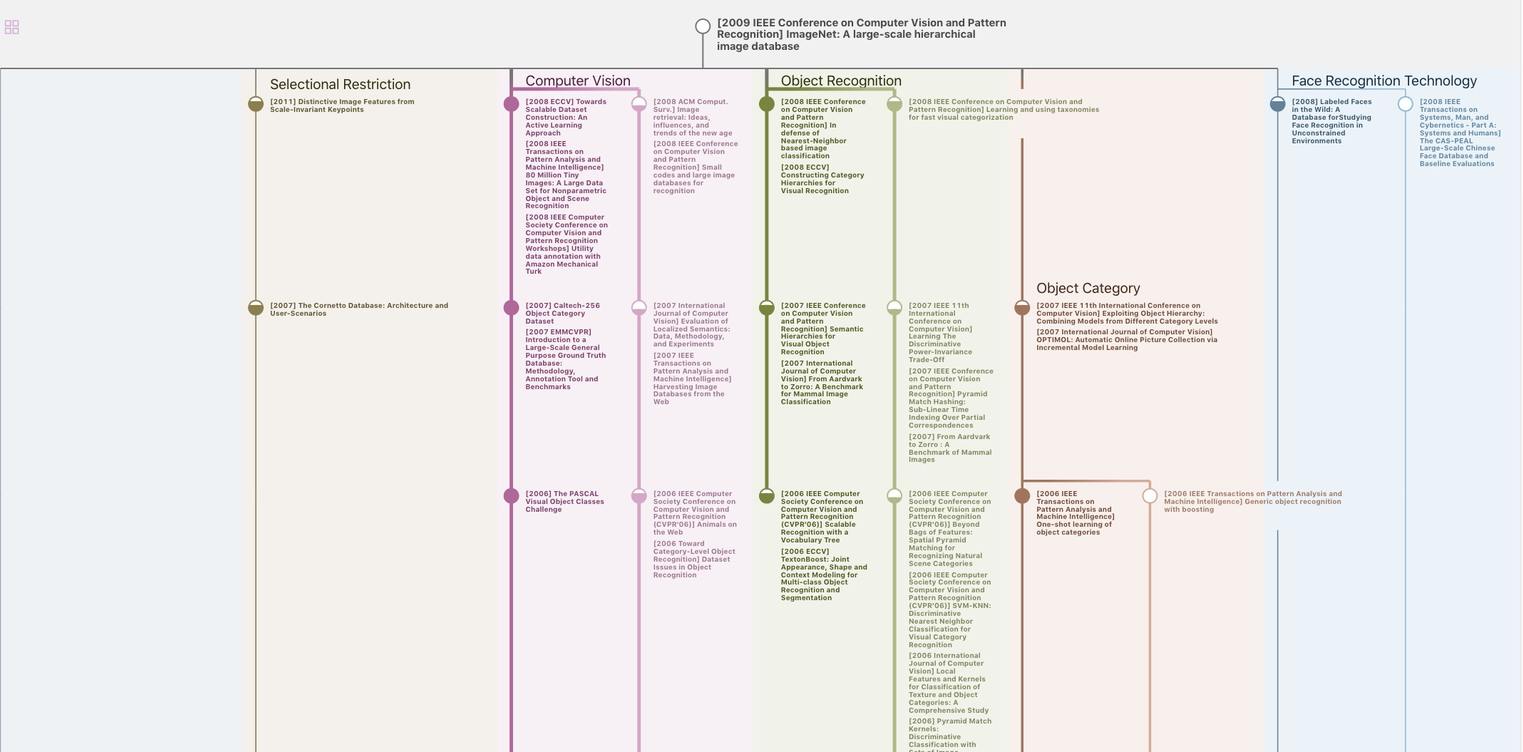
生成溯源树,研究论文发展脉络
Chat Paper
正在生成论文摘要