Federated Mutual Learning
arxiv(2020)
摘要
Federated learning (FL) enables collaboratively training deep learning models on decentralized data. However, there are three types of heterogeneities in FL setting bringing about distinctive challenges to the canonical federated learning algorithm (FedAvg). First, due to the Non-IIDness of data, the global shared model may perform worse than local models that solely trained on their private data; Second, the objective of center server and clients may be different, where center server seeks for a generalized model whereas client pursue a personalized model, and clients may run different tasks; Third, clients may need to design their customized model for various scenes and tasks; In this work, we present a novel federated learning paradigm, named Federated Mutual Leaning (FML), dealing with the three heterogeneities. FML allows clients training a generalized model collaboratively and a personalized model independently, and designing their private customized models. Thus, the Non-IIDness of data is no longer a bug but a feature that clients can be personally served better. The experiments show that FML can achieve better performance than alternatives in typical FL setting, and clients can be benefited from FML with different models and tasks.
更多查看译文
关键词
learning
AI 理解论文
溯源树
样例
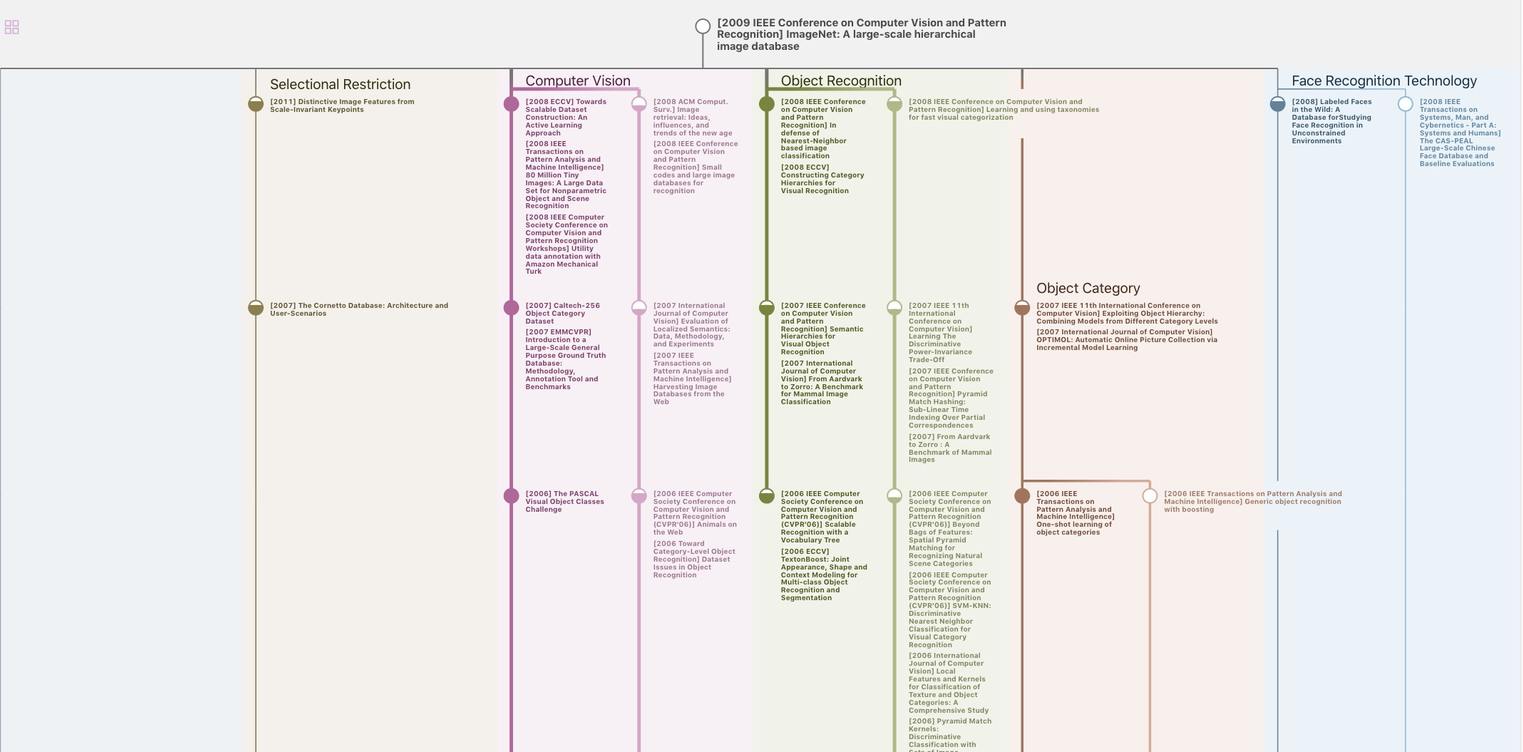
生成溯源树,研究论文发展脉络
Chat Paper
正在生成论文摘要