OIFlow: Occlusion-Inpainting Optical Flow Estimation by Unsupervised Learning.
CoRR(2021)
摘要
Occlusion is an inevitable and critical problem in unsupervised optical flow learning. Existing methods either treat occlusions equally as non-occluded regions or simply remove them to avoid incorrectness. However, the occlusion regions can provide effective information for optical flow learning. In this paper, we present OIFlow, an occlusion-inpainting framework to make full use of occlusion regions. Specifically, a new appearance-flow network is proposed to inpaint occluded flows based on the image content. Moreover, a boundary dilated warp is proposed to deal with occlusions caused by displacement beyond the image border. We conduct experiments on multiple leading flow benchmark datasets such as Flying Chairs, KITTI and MPI-Sintel, which demonstrate that the performance is significantly improved by our proposed occlusion handling framework.
更多查看译文
关键词
Optical imaging, Feature extraction, Optical variables control, Estimation, Optical fiber networks, Adaptive optics, Optical losses, Optical flow, inpainting, appearance flow, occlusion, unsupervised learning
AI 理解论文
溯源树
样例
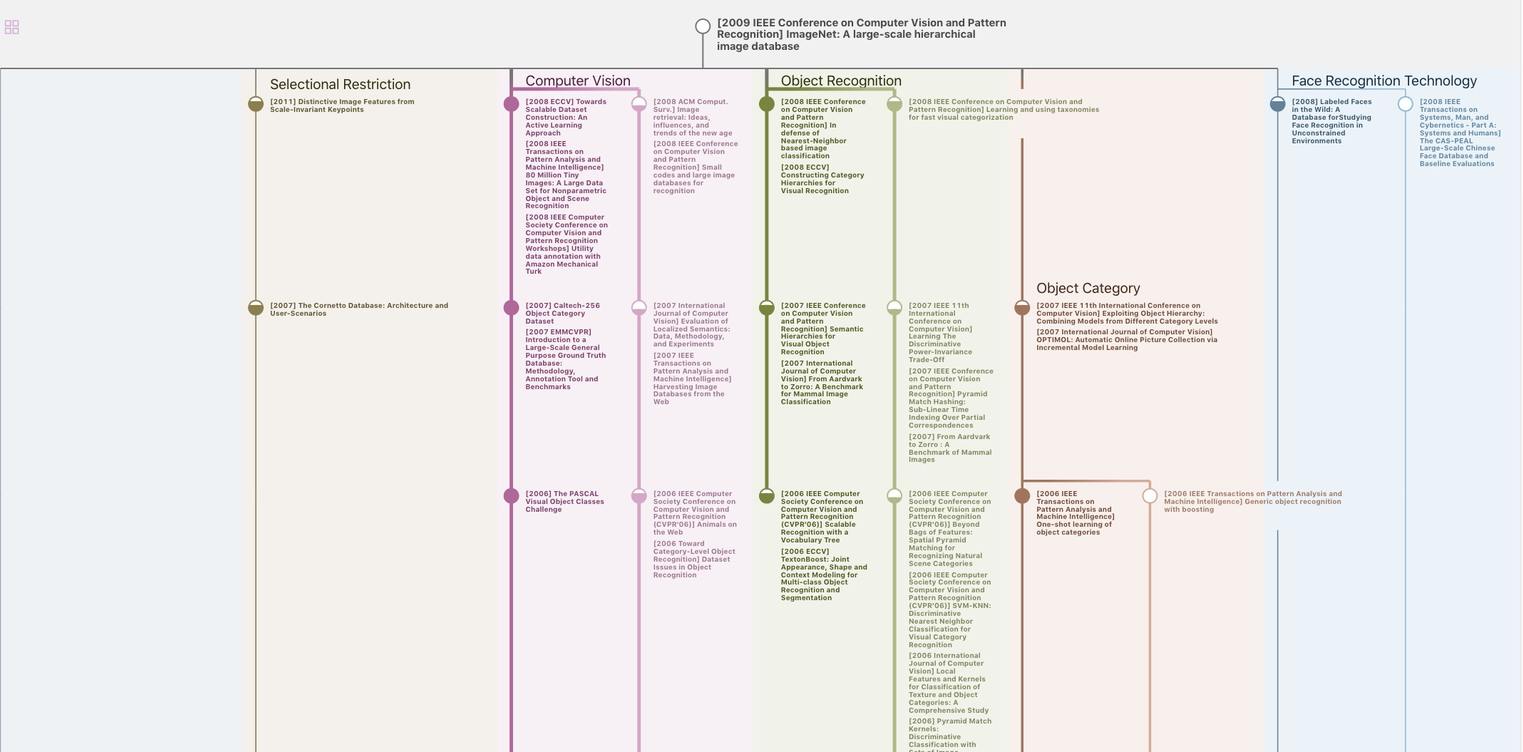
生成溯源树,研究论文发展脉络
Chat Paper
正在生成论文摘要