Investigating Weak Supervision in Deep Ranking.
Data Inf. Manag.(2019)
摘要
A number of deep neural networks have been proposed to improve the performance of document ranking in information retrieval studies. However, the training processes of these models usually need a large scale of labeled data, leading to data shortage becoming a major hindrance to the improvement of neural ranking models’ performances. Recently, several weakly supervised methods have been proposed to address this challenge with the help of heuristics or users’ interaction in the Search Engine Result Pages (SERPs) to generate weak relevance labels. In this work, we adopt two kinds of weakly supervised relevance, BM25-based relevance and click model-based relevance, and make a deep investigation into their differences in the training of neural ranking models. Experimental results show that BM25-based relevance helps models capture more exact matching signals, while click model-based relevance enhances the rankings of documents that may be preferred by users. We further proposed a cascade ranking framework to combine the two weakly supervised relevance, which significantly promotes the ranking performance of neural ranking models and outperforms the best result in the last NTCIR-13 We Want Web (WWW) task. This work reveals the potential of constructing better document retrieval systems based on multiple kinds of weak relevance signals.
更多查看译文
关键词
document ranking,ad hoc retrieval,neural ranking model,weak supervision
AI 理解论文
溯源树
样例
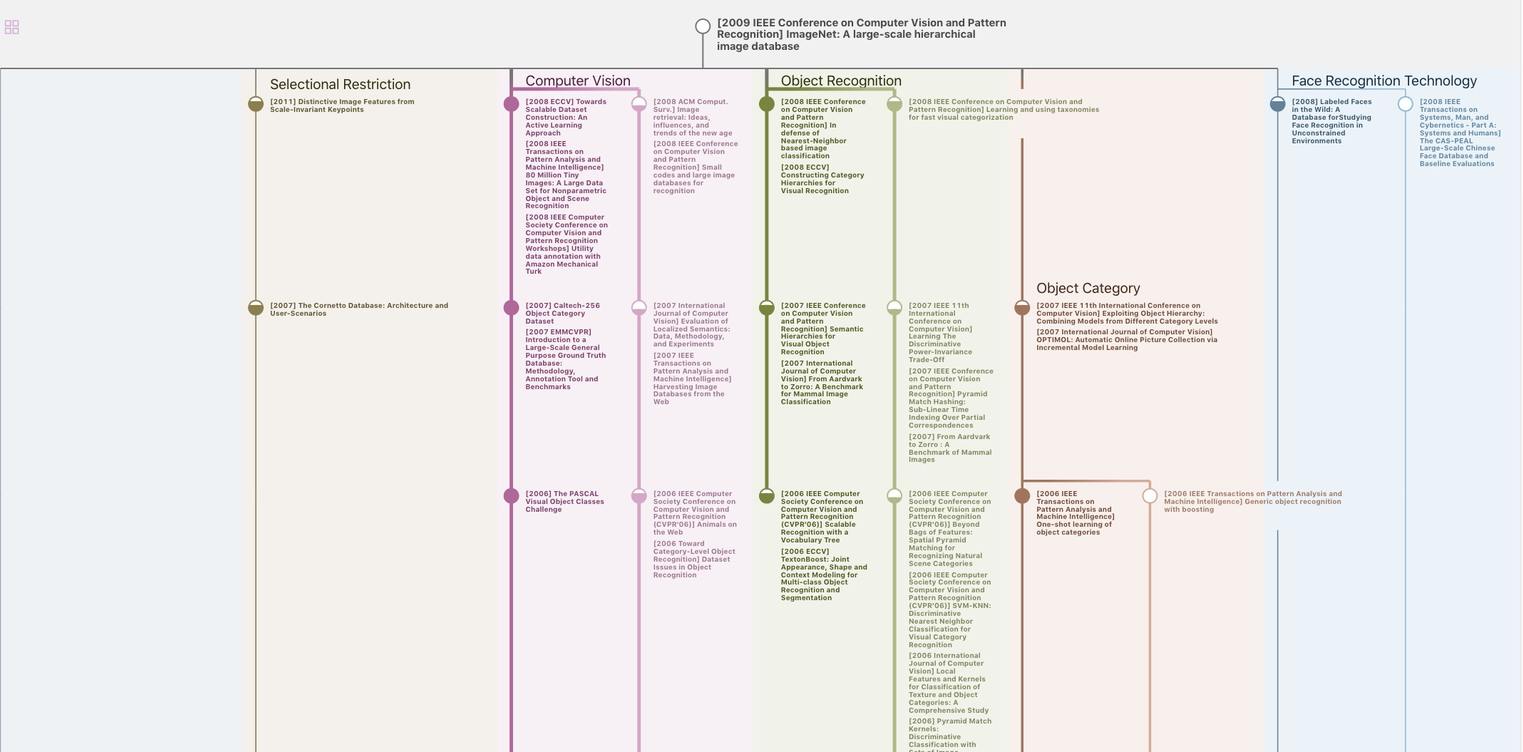
生成溯源树,研究论文发展脉络
Chat Paper
正在生成论文摘要