Machine Learning Etudes in Conformal Field Theories
arXiv (Cornell University)(2020)
摘要
We demonstrate that various aspects of Conformal Field Theory are amenable to machine learning. Relatively modest feed-forward neural networks are able to distinguish between scale and conformal invariance of a three-point function and identify a crossing-symmetric four-point function to nearly a hundred percent accuracy. Furthermore, neural networks are also able to identify conformal blocks appearing in a putative CFT four-point function and predict the values of the corresponding OPE coefficients. Neural networks also successfully classify primary operators by their quantum numbers under discrete symmetries in the CFT from examining OPE data. We also demonstrate that neural networks are able to learn the available OPE data for scalar correlation function in the 3d Ising model and predict the twists of higher-spin operators that appear in scalar OPE channels by regression.
更多查看译文
AI 理解论文
溯源树
样例
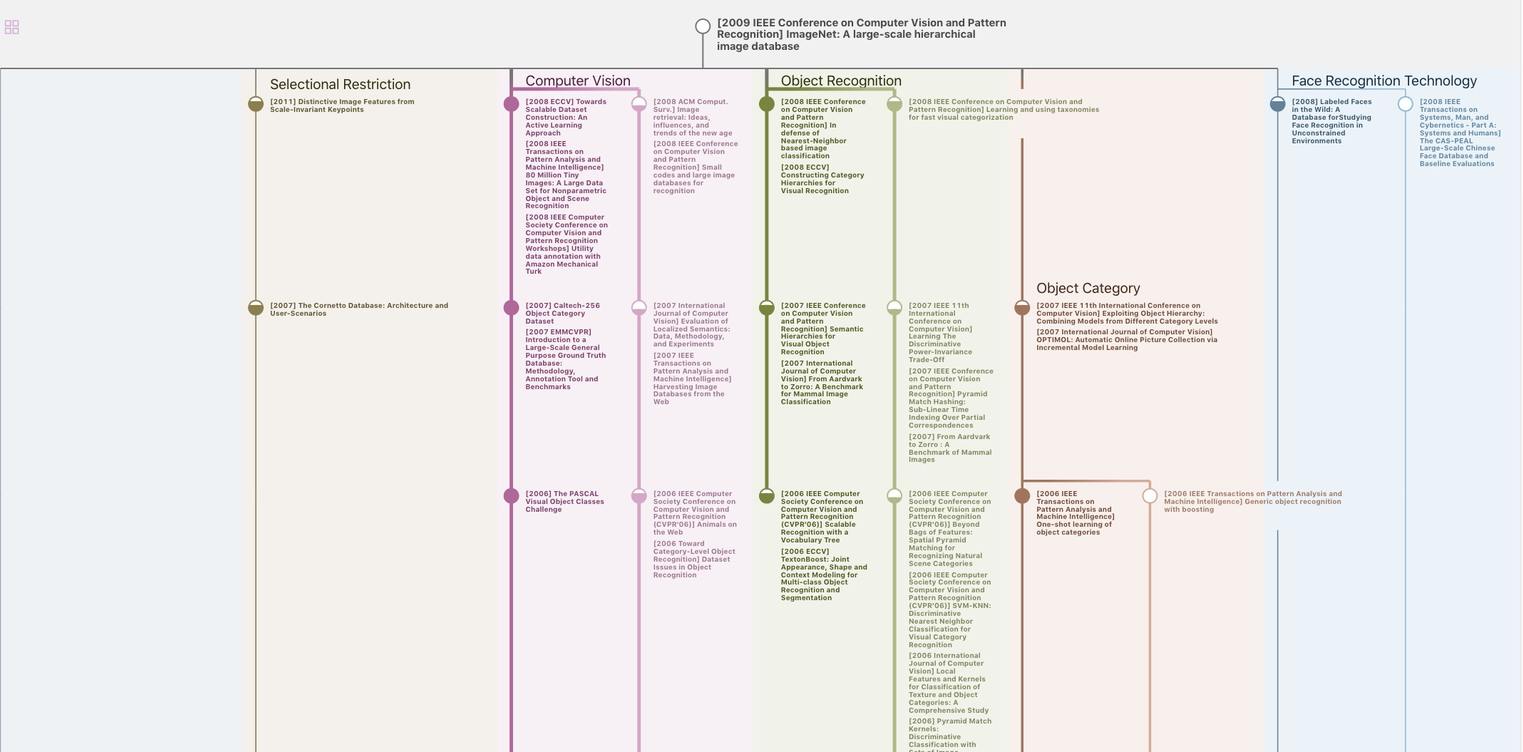
生成溯源树,研究论文发展脉络
Chat Paper
正在生成论文摘要