On Regret with Multiple Best Arms
NIPS 2020(2020)
摘要
We study a regret minimization problem with the existence of multiple best/near-optimal arms in the multi-armed bandit setting. We consider the case when the number of arms/actions is comparable or much larger than the time horizon, and make no assumptions about the structure of the bandit instance. Our goal is to design algorithms that can automatically adapt to the unknown hardness of the problem, i.e., the number of best arms. Our setting captures many modern applications of bandit algorithms where the action space is enormous and the information about the underlying instance/structure is unavailable. We first propose an adaptive algorithm that is agnostic to the hardness level and theoretically derive its regret bound. We then prove a lower bound for our problem setting, which indicates: (1) no algorithm can be minimax optimal simultaneously over all hardness levels; and (2) our algorithm achieves a rate function that is Pareto optimal. With additional knowledge of the expected reward of the best arm, we propose another adaptive algorithm that is minimax optimal, up to polylog factors, over all hardness levels. Experimental results confirm our theoretical guarantees and show advantages of our algorithms over the previous state-of-the-art.
更多查看译文
关键词
multiple best arms,regret
AI 理解论文
溯源树
样例
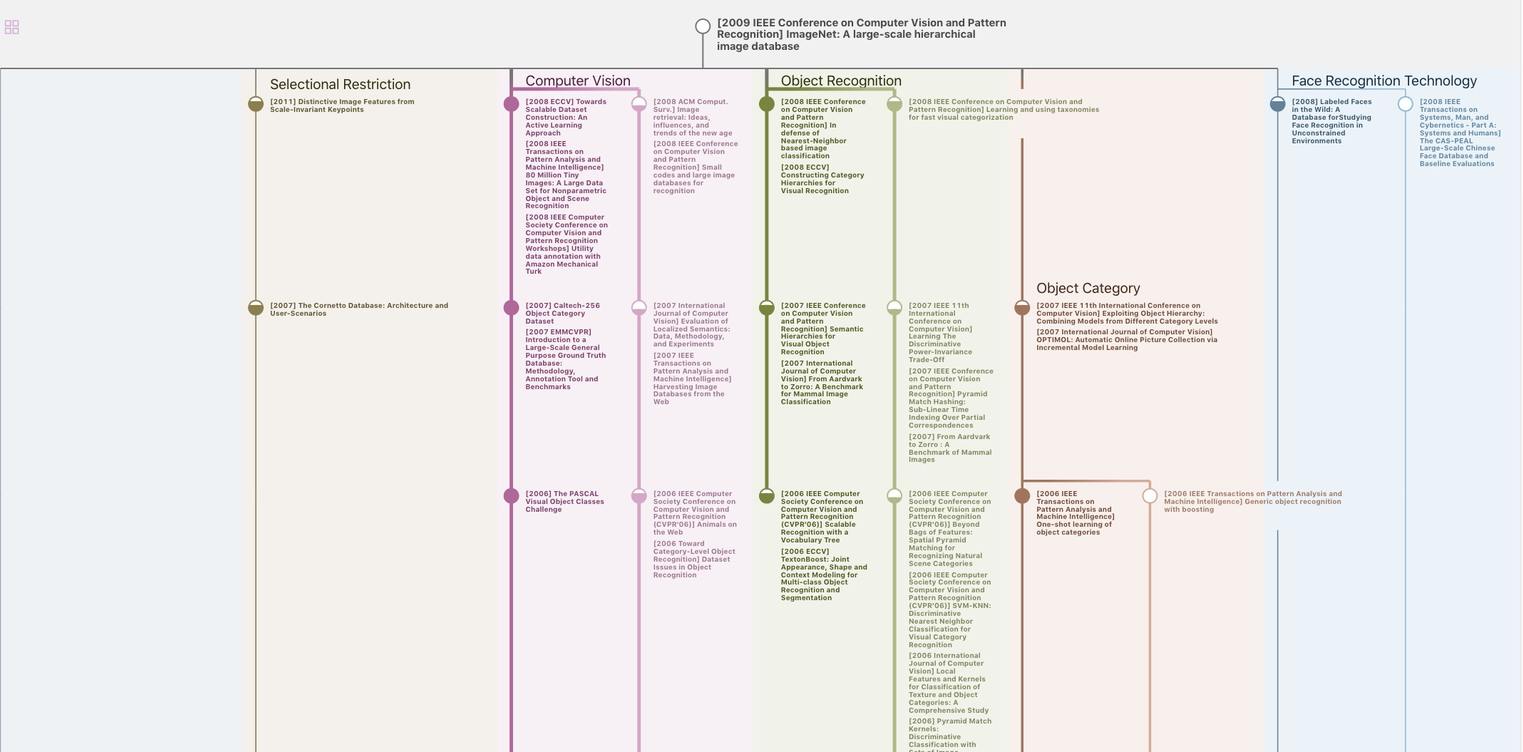
生成溯源树,研究论文发展脉络
Chat Paper
正在生成论文摘要