Deep Latent Low-Rank Fusion Network for Progressive Subspace Discovery
IJCAI 2020(2020)
摘要
Low-rank representation is powerful for recovering and clustering the subspace structures, but it cannot obtain deep hierarchical information due to the single-layer mode. In this paper, we present a new and effective strategy to extend the single-layer latent low-rank models into multiple-layers, and propose a new and progressive Deep Latent Low-Rank Fusion Network (DLRF-Net) to uncover deep features and structures embedded in input data. The basic idea of DLRF-Net is to refine features progressively from the previous layers by fusing the subspaces in each layer, which can potentially obtain accurate features and subspaces for representation. To learn deep information, DLRF-Net inputs shallow features of the last layers into subsequent layers. Then, it recovers the deeper features and hierarchical information by congregating the projective subspaces and clustering subspaces respectively in each layer. Thus, one can learn hierarchical subspaces, remove noise and discover the underlying clean subspaces. Note that most existing latent low-rank coding models can be extended to multilayers using DLRF-Net. Extensive results show that our network can deliver enhanced performance over other related frameworks.
更多查看译文
AI 理解论文
溯源树
样例
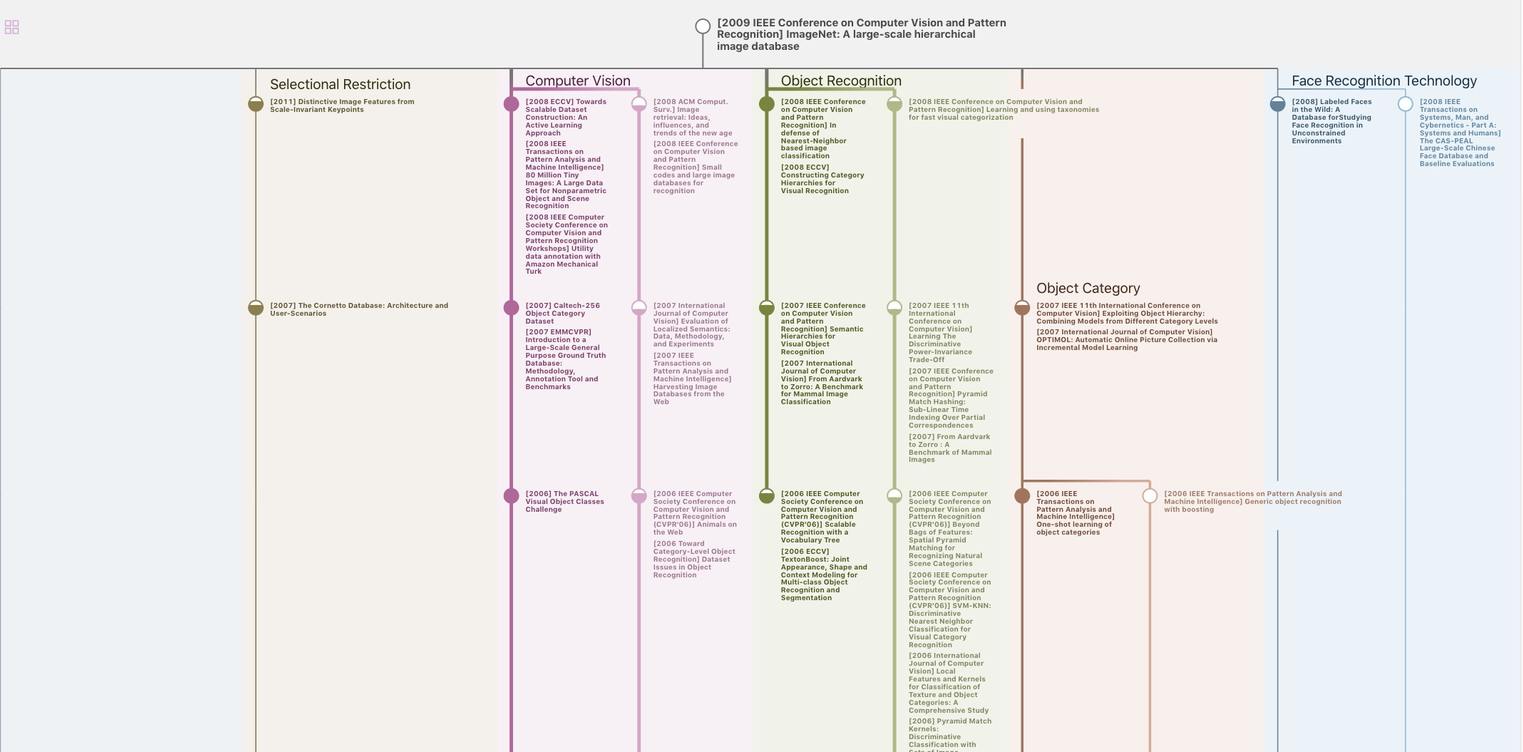
生成溯源树,研究论文发展脉络
Chat Paper
正在生成论文摘要