A Spatial Missing Value Imputation Method for Multi-view Urban Statistical Data
IJCAI 2020(2020)
摘要
Large volumes of urban statistical data with multiple views imply rich knowledge about the development degree of cities. These data present crucial statistics which play an irreplaceable role in the regional analysis and urban computing. In reality, however, the statistical data divided into fine-grained regions usually suffer from missing data problems. Those missing values hide the useful information that may result in a distorted data analysis. Thus, in this paper, we propose a spatial missing data imputation method for multi-view urban statistical data. To address this problem, we exploit an improved spatial multi-kernel clustering method to guide the imputation process cooperating with an adaptive-weight non-negative matrix factorization strategy. Intensive experiments are conducted with other state-of-the-art approaches on six real-world urban statistical datasets. The results not only show the superiority of our method against other comparative methods on different datasets, but also represent a strong generalizability of our model.
更多查看译文
AI 理解论文
溯源树
样例
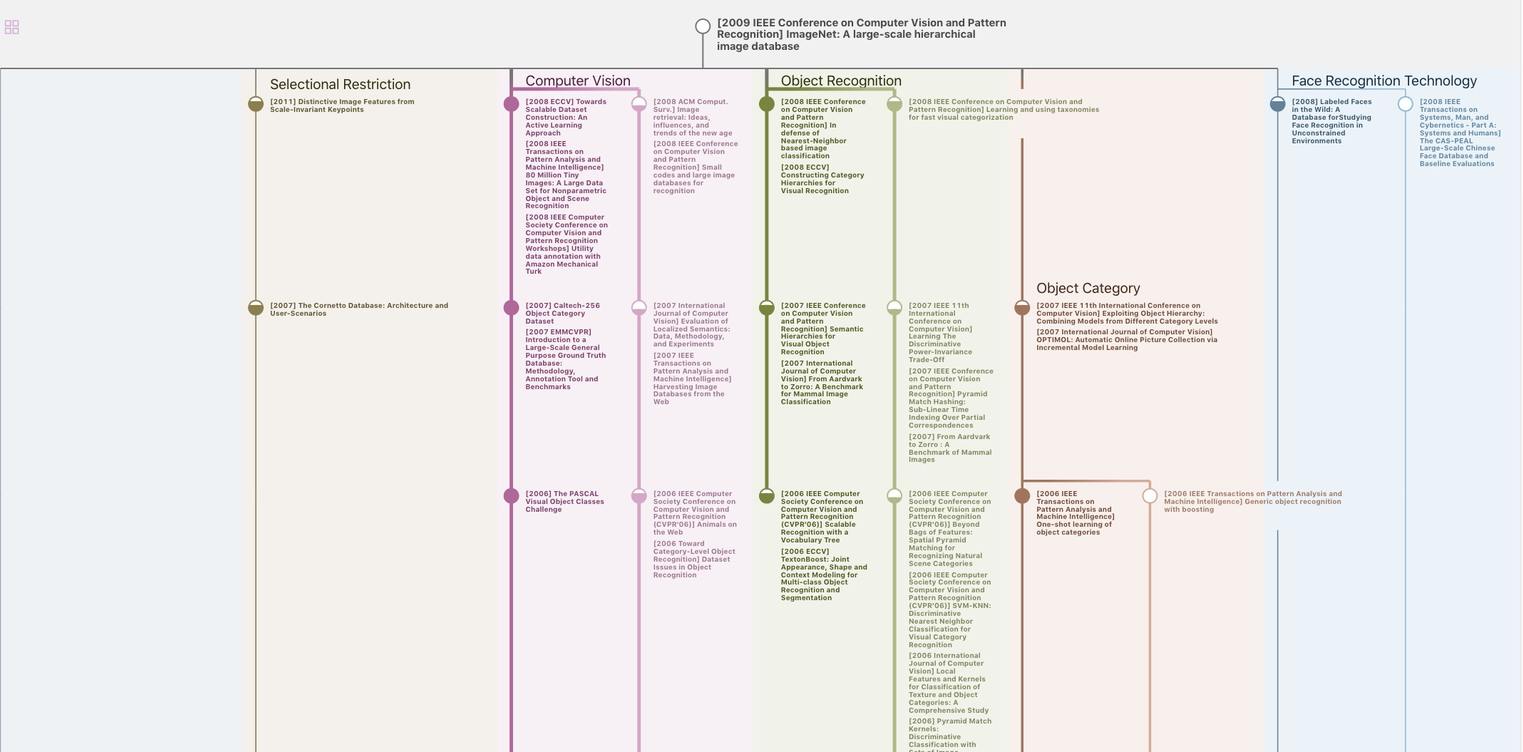
生成溯源树,研究论文发展脉络
Chat Paper
正在生成论文摘要