GRAMARCH: A GPU-ReRAM based Heterogeneous Architecture for Neural Image Segmentation
2020 Design, Automation & Test in Europe Conference & Exhibition (DATE)(2020)
摘要
Deep Neural Networks (DNNs) employed for image segmentation are computationally more expensive and complex compared to the ones used for classification. However, manycore architectures to accelerate the training of these DNNs are relatively unexplored. Resistive random-access memory (ReRAM)-based architectures offer a promising alternative to commonly used GPU-based platforms for training DNNs. However, due to their low-precision storage capability, these architectures cannot support all DNN layers and suffer from accuracy loss of the learned models. To address these challenges, we propose GRAMARCH, a heterogeneous architecture that combines the benefits of ReRAM and GPUs simultaneously by using a high-throughput 3D Network-on-Chip. Experimental results indicate that by suitably mapping DNN layers to processing elements, it is possible to achieve up to 53X better performance compared to conventional GPUs for image segmentation.
更多查看译文
关键词
Heterogenous,3D,ReRAM,NoC,DNNs
AI 理解论文
溯源树
样例
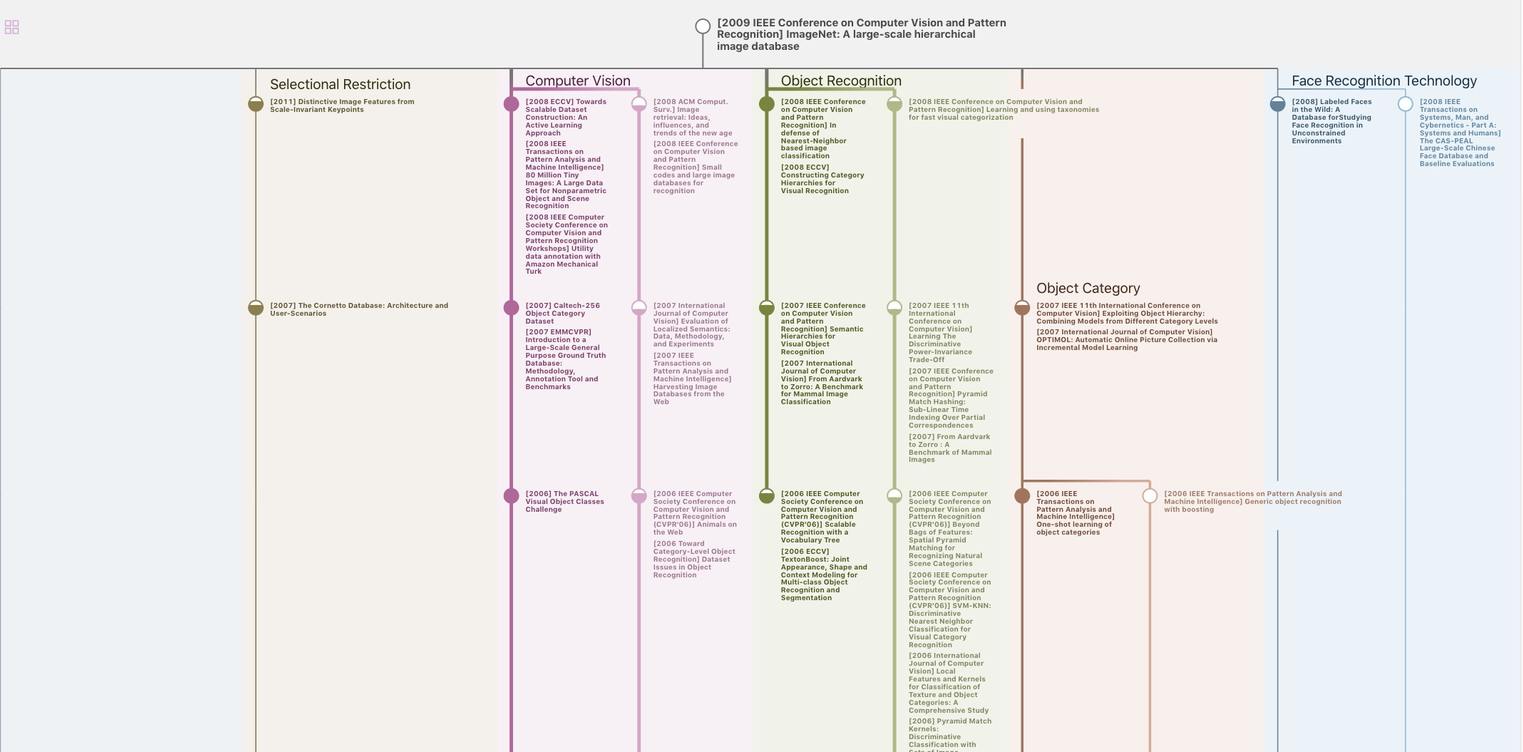
生成溯源树,研究论文发展脉络
Chat Paper
正在生成论文摘要