An Efficient FPGA-based Architecture for Contractive Autoencoders
2020 IEEE 28th Annual International Symposium on Field-Programmable Custom Computing Machines (FCCM)(2020)
摘要
Deep learning neural networks have gained much attention in recent research. Excellent results in various domains have proved the usefulness of such algorithms. However, training a deep learning network requires substantial computational effort; therefore, resource-constrained systems like edge devices in the IoT domain still lack full implementations, and training of the network is offloaded to the cloud. Online or unsupervised training of the network, on the other hand, is often a must if the system has to adjust to possible drift of the environment parameters or there is not enough data available initially. This paper proposes the first Xilinx Zynq FPGA (Field Programmable Gate Array) based implementation of the contractive autoencoder (CAE), including training of the network.
更多查看译文
关键词
Xilinx Zynq FPGA,contractive autoencoder,FPGA-based architecture,deep learning network,resource-constrained systems,edge devices,IoT domain,unsupervised training,online training
AI 理解论文
溯源树
样例
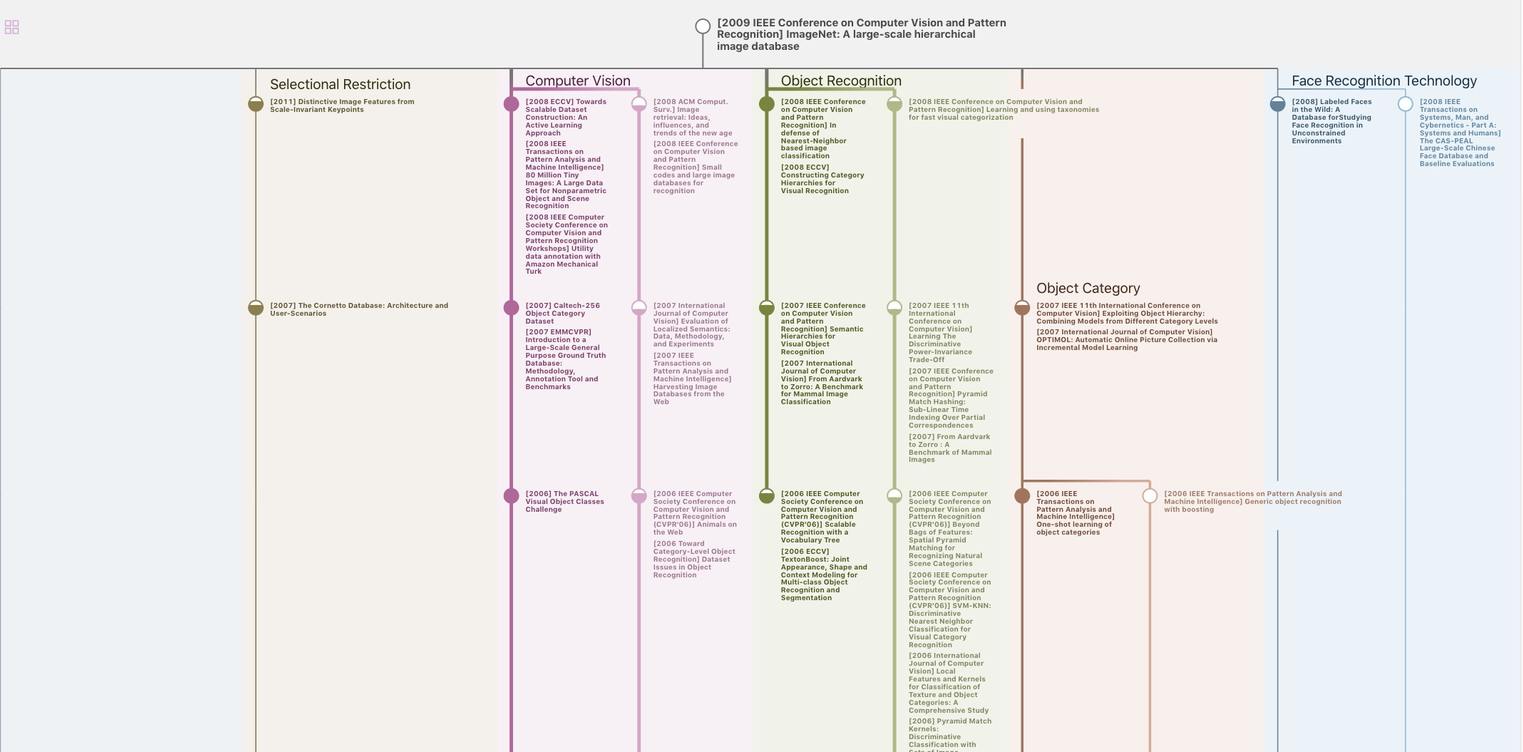
生成溯源树,研究论文发展脉络
Chat Paper
正在生成论文摘要