Automatic task recognition in a flexible endoscopy benchtop trainer with semi-supervised learning
INTERNATIONAL JOURNAL OF COMPUTER ASSISTED RADIOLOGY AND SURGERY(2020)
摘要
Purpose Inexpensive benchtop training systems offer significant advantages to meet the increasing demand of training surgeons and gastroenterologists in flexible endoscopy. Established scoring systems exist, based on task duration and mistake evaluation. However, they require trained human raters, which limits broad and low-cost adoption. There is an unmet and important need to automate rating with machine learning. Method We present a general and robust approach for recognizing training tasks from endoscopic training video, which consequently automates task duration computation. Our main technical novelty is to show the performance of state-of-the-art CNN-based approaches can be improved significantly with a novel semi-supervised learning approach, using both labelled and unlabelled videos. In the latter case, we assume only the task execution order is known a priori. Results Two video datasets are presented: the first has 19 videos recorded in examination conditions, where the participants complete their tasks in predetermined order. The second has 17 h of videos recorded in self-assessment conditions, where participants complete one or more tasks in any order. For the first dataset, we obtain a mean task duration estimation error of 3.65 s, with a mean task duration of 159 s ( 2.3% relative error). For the second dataset, we obtain a mean task duration estimation error of 3.67 s. We reduce an average of 5.63
更多查看译文
关键词
Flexible endoscopy, Education, Skill, Benchtop simulator, Phase recognition, Semi-supervised learning
AI 理解论文
溯源树
样例
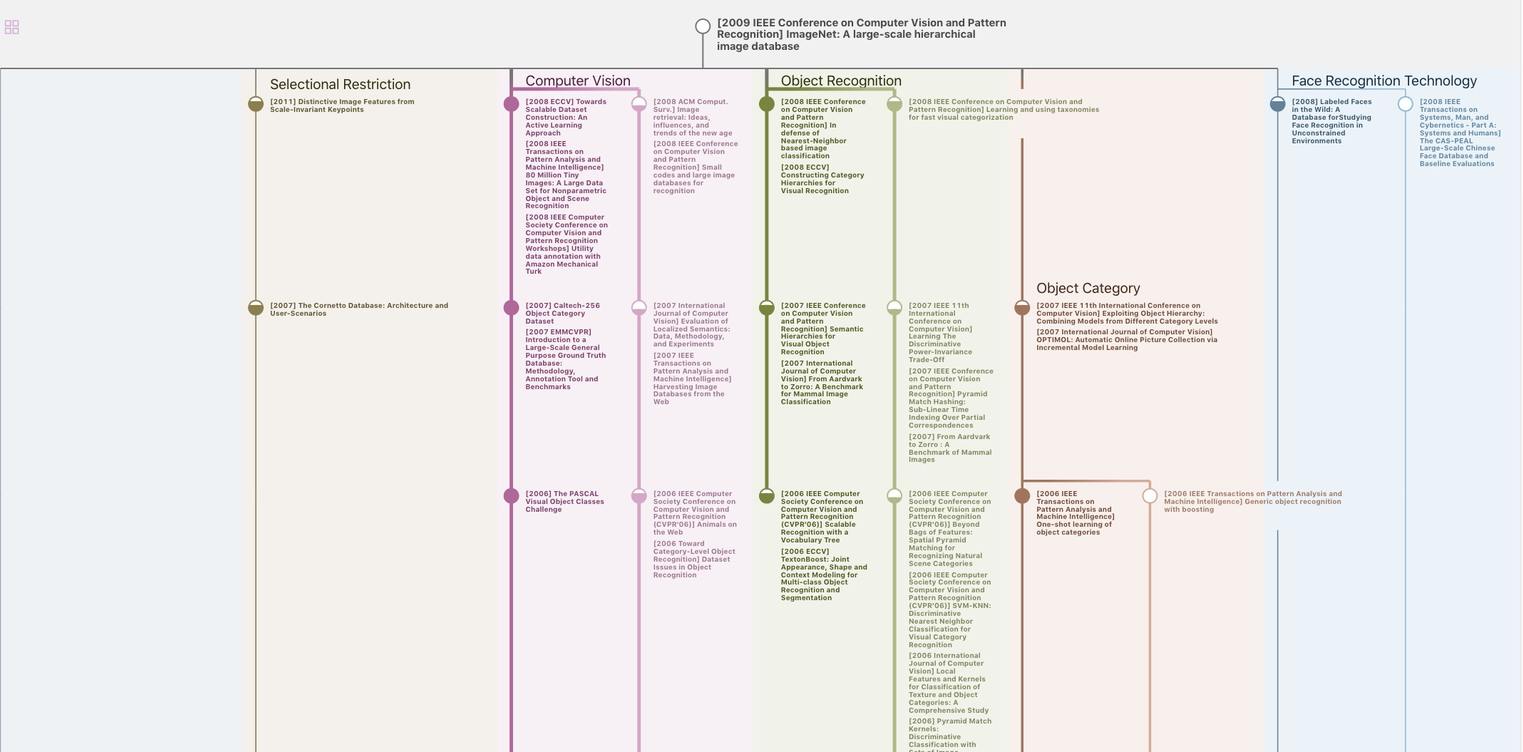
生成溯源树,研究论文发展脉络
Chat Paper
正在生成论文摘要