Time-varying networks of ERPs in P300-speller paradigms based on spatially and semantically congruent audiovisual bimodality.
JOURNAL OF NEURAL ENGINEERING(2020)
摘要
Objective In the P300-speller paradigm using spatially and semantically congruent audiovisual (AV) stimuli, AV stimuli elicited significantly higher event-related potential (ERP) amplitudes than those for a single visual (V) stimulus. Nevertheless, it remains unclear whether ERPs in AV and V spelling paradigms are generated with identical brain network architecture. Approach In this study, we constructed time-varying networks for ERPs in AV and V spelling paradigms based on adaptive directed transfer function to investigate the dynamic processes underpinning the processing of stimuli in the two spelling paradigms. Main results Our analysis revealed that early AV integration, AV spatial and semantic information, and late AV integration enhanced activation in attention-related areas between 40-160 ms, areas associated with attention to target stimuli between 200-280 ms, and areas associated with decision-making between 320-520 ms, respectively. Left temporal areas were associated with AV spatial-semantic information-processing and late AV integration, the activation of which impacted the activation of brain areas associated with attention (P3a) and decision-making (P3b). In addition, the ability and efficiency of information transmission from brain networks in the AV spelling paradigm were significantly stronger than those in the V spelling paradigm between 40 and 160 ms, 200 and 280 ms, and 320 and 520 ms. Significance This work provides a theoretical basis for deepening our understanding of the neural mechanisms underscoring ERPs production in the AV P300-speller paradigm as well as a theoretical reference for further optimization of the induced paradigm based on AV stimuli and improves the performance of BCI system.
更多查看译文
关键词
audiovisual,event-related potentials,P300-speller paradigm,spatial and semantical information,time-varying network
AI 理解论文
溯源树
样例
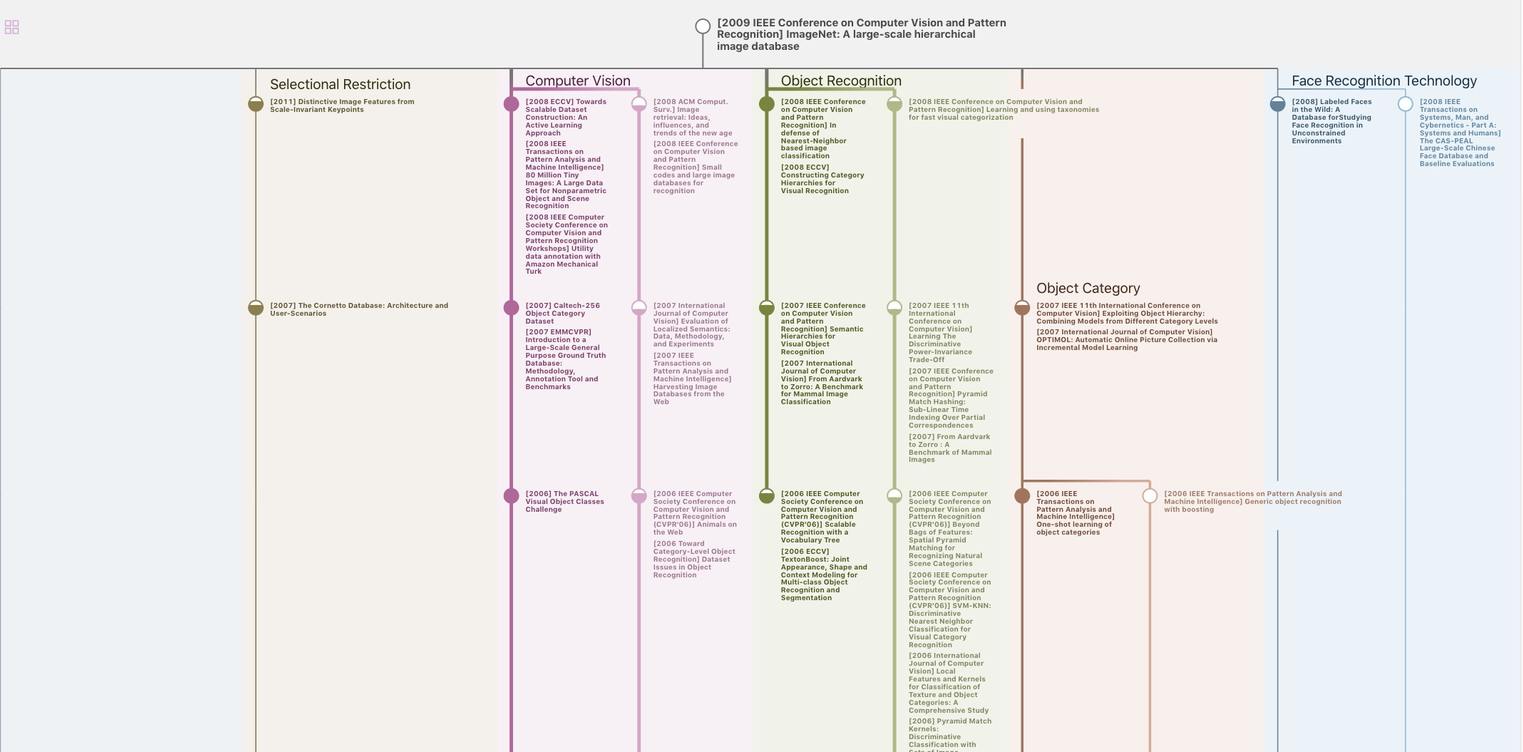
生成溯源树,研究论文发展脉络
Chat Paper
正在生成论文摘要