BAgger: A Bayesian algorithm for safe and query-efficient imitation learning
Machine Learning in Robot Motion Planning–IROS 2018 Workshop(2018)
摘要
Safety and query efficiency may present a challenge when learning a robot control policy with Dataset Aggregation (DAgger). We propose BAgger, an Imitation Learning algorithm that, using a Bayesian approach, aims to mitigate those challenges by predicting state novelty and policy error. In BAgger, the expert is queried only when there is a significant risk of not being able to imitate the expert, eg in novel parts of the state space. We present empirical results indicating that BAgger is, both, safer than DAgger and SafeDAgger on a robot control task, while still being query-efficient.
更多查看译文
AI 理解论文
溯源树
样例
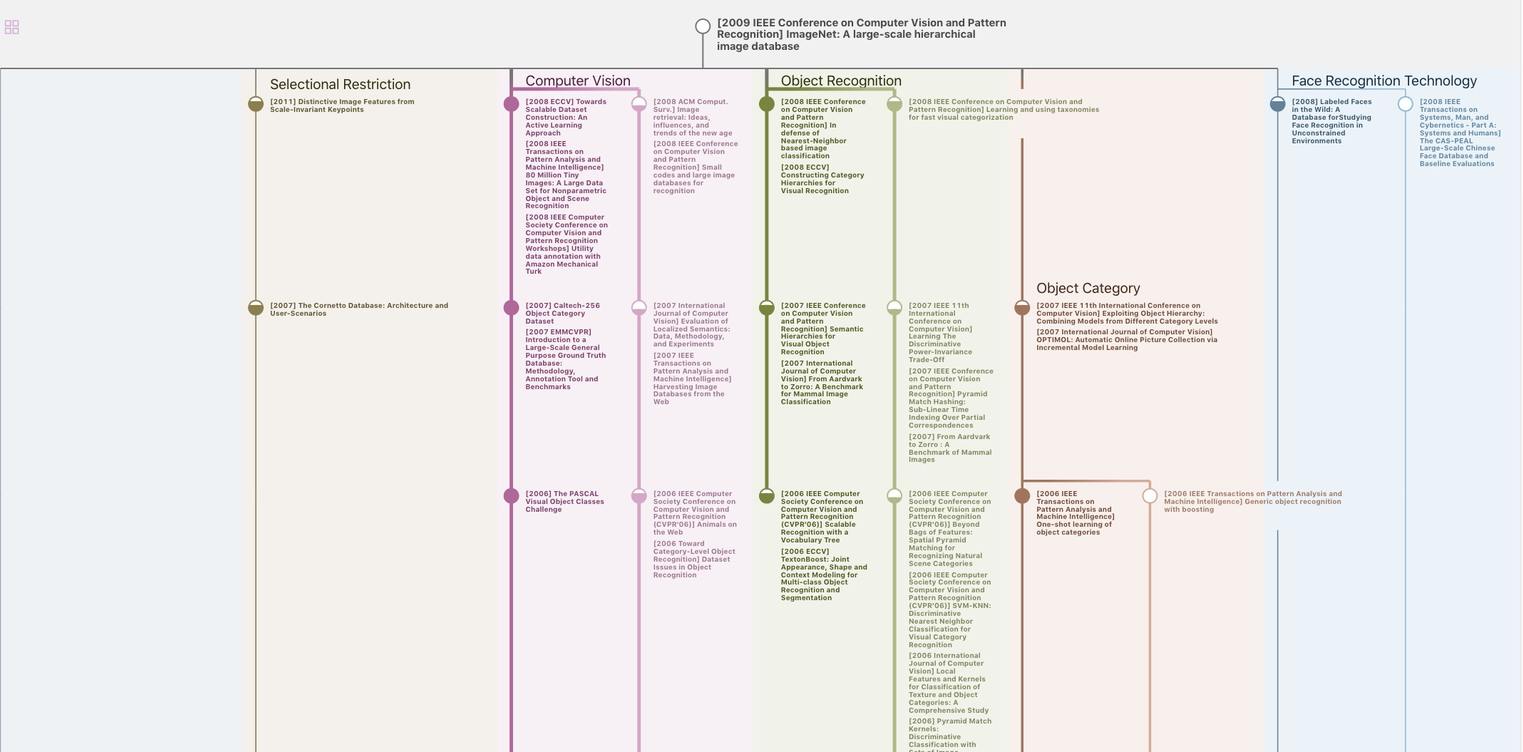
生成溯源树,研究论文发展脉络
Chat Paper
正在生成论文摘要