Towards Differentially Private Text Representations
SIGIR '20: The 43rd International ACM SIGIR conference on research and development in Information Retrieval Virtual Event China July, 2020(2020)
摘要
Most deep learning frameworks require users to pool their local data or model updates to a trusted server to train or maintain a global model. The assumption of a trusted server who has access to user information is ill-suited in many applications. To tackle this problem, we develop a new deep learning framework under an untrusted server setting, which includes three modules: (1) embedding module, (2) randomization module, and (3) classifier module. For the randomization module, we propose a novel local differentially private (LDP) protocol to reduce the impact of privacy parameter ε on accuracy, and provide enhanced flexibility in choosing randomization probabilities for LDP. Analysis and experiments show that our framework delivers comparable or even better performance than the non-private framework and existing LDP protocols, demonstrating the advantages of our LDP protocol.
更多查看译文
关键词
representations,text
AI 理解论文
溯源树
样例
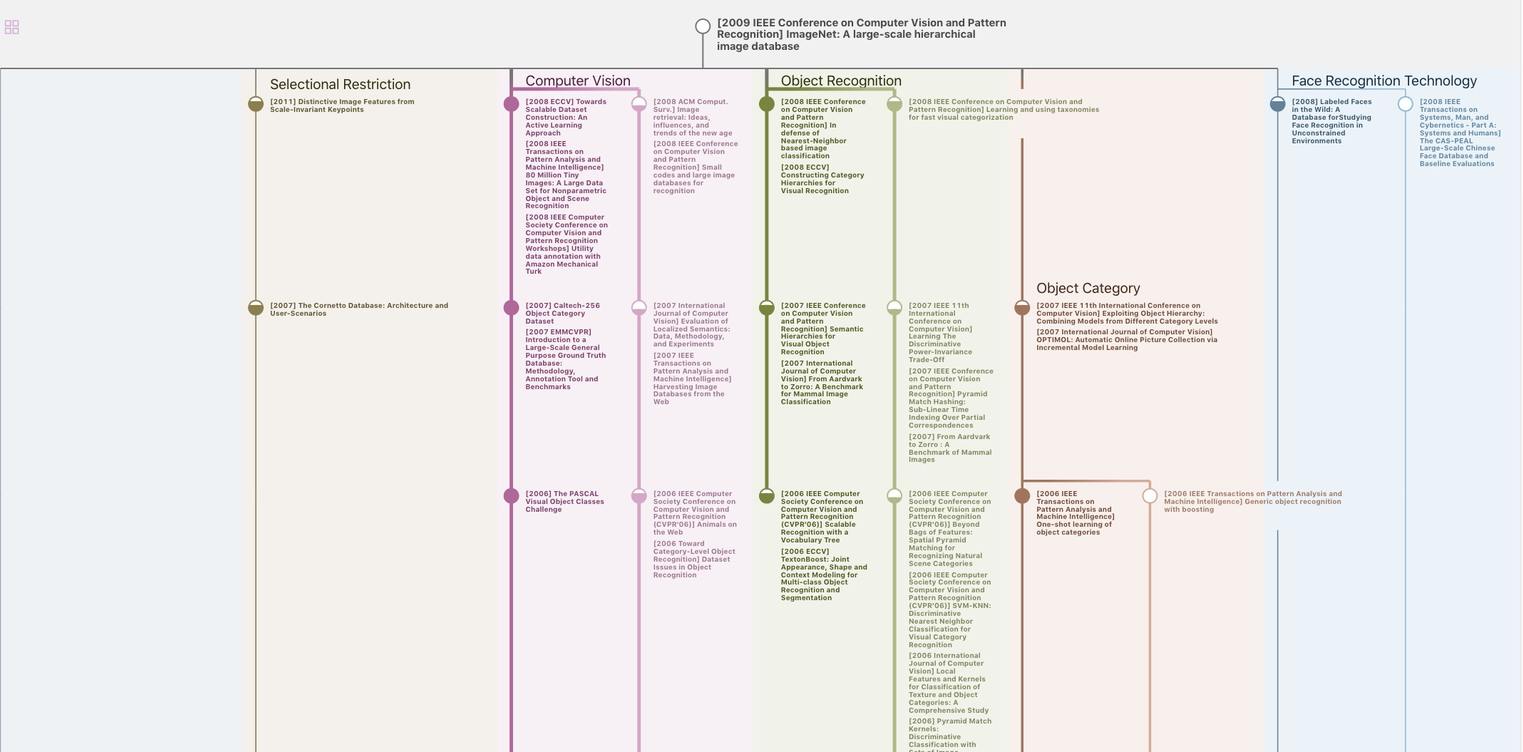
生成溯源树,研究论文发展脉络
Chat Paper
正在生成论文摘要