Random Forests applied to High Precision Photometry Analysis with Spitzer IRAC
arxiv(2020)
摘要
We present a new method employing machine learning techniques for measuring astrophysical features by correcting systematics in IRAC high precision photometry using Random Forests. The main systematic in IRAC light curve data is position changes due to unavoidable telescope motions coupled with an intrapixel response function. We aim to use the large amount of publicly available calibration data for the single pixel used for this type of work (the sweet spot pixel) to make a fast, easy to use, accurate correction to science data. This correction on calibration data has the advantage of using an independent dataset instead of using the science data on itself, which has the disadvantage of including astrophysical variations. After focusing on feature engineering and hyperparameter optimization, we show that a boosted random forest model can reduce the data such that we measure the median of ten archival eclipse observations of XO-3b to be 1459 +- 200 parts per million. This is a comparable depth to the average of those in the literature done by seven different methods, however the spread in measurements is 30-100 than those literature values, depending on the reduction method. We also caution others attempting similar methods to check their results with the fiducial dataset of XO-3b as we were also able to find models providing initially great scores on their internal test datasets but whose results significantly underestimated the eclipse depth of that planet.
更多查看译文
关键词
Exoplanets,Infrared astronomy,Random Forests,Astronomy software,Space telescopes,Infrared telescopes,Astronomy data reduction
AI 理解论文
溯源树
样例
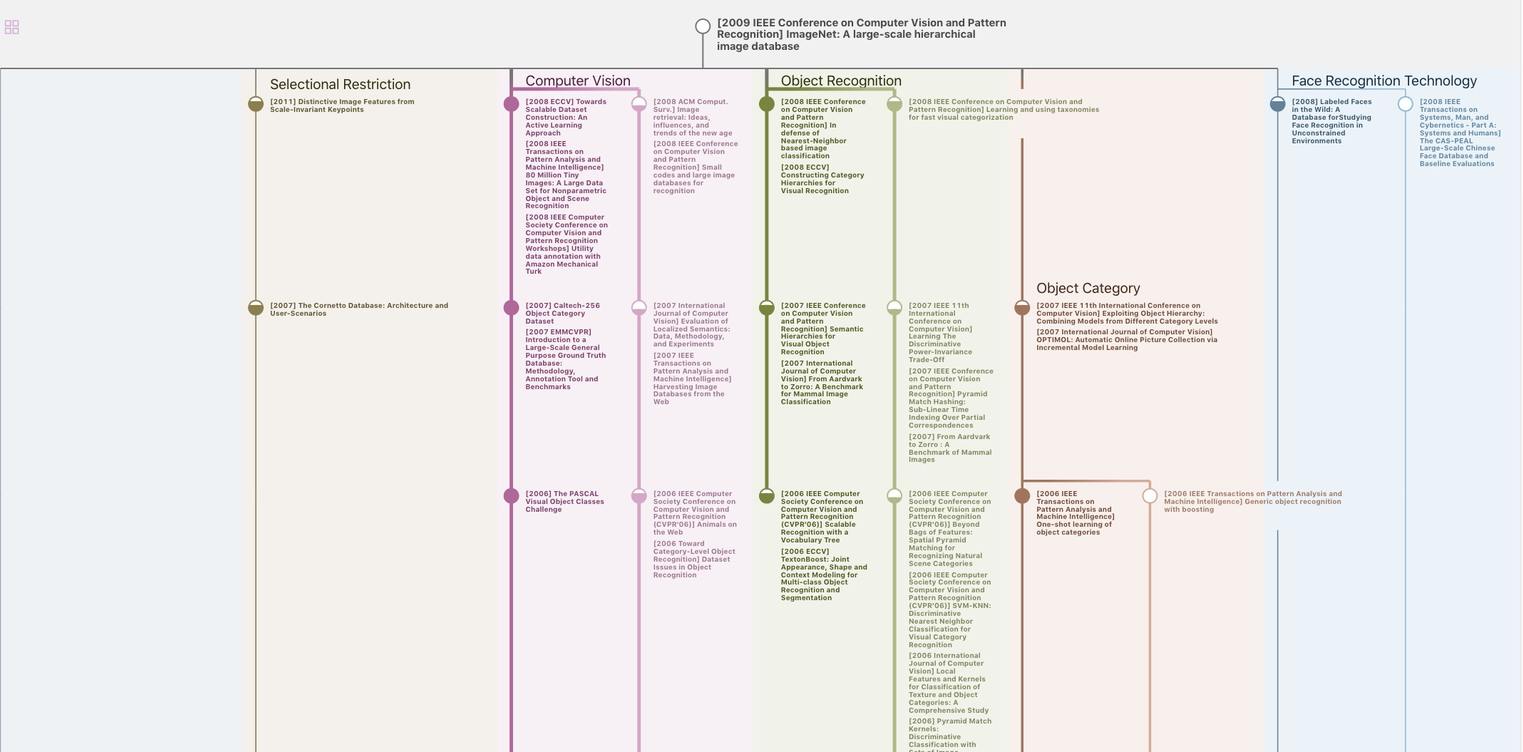
生成溯源树,研究论文发展脉络
Chat Paper
正在生成论文摘要