Machine learning the real discriminant locus
Journal of Symbolic Computation(2023)
摘要
Parameterized systems of polynomial equations arise in many applications in science and engineering with the real solutions describing, for example, equilibria of a dynamical system, linkages satisfying design constraints, and scene reconstruction in computer vision. Since different parameter values can have a different number of real solutions, the parameter space is decomposed into regions whose boundary forms the real discriminant locus. This article views locating the real discriminant locus as a supervised classification problem in machine learning where the goal is to determine classification boundaries over the parameter space, with the classes being the number of real solutions. This article presents a novel sampling method which carefully samples a multidimensional parameter space. At each sample point, homotopy continuation is used to obtain the number of real solutions to the corresponding polynomial system. Machine learning techniques including nearest neighbors, support vector classifiers, and neural networks are used to efficiently approximate the real discriminant locus. One application of having learned the real discriminant locus is to develop a real homotopy method that only tracks real solution paths unlike traditional methods which track all complex solution paths. Examples show that the proposed approach can efficiently approximate complicated solution boundaries such as those arising from the equilibria of the N=4 Kuramoto model which was previously intractable using traditional methods.
更多查看译文
关键词
Discriminant locus,Machine learning,Deep learning,Numerical algebraic geometry
AI 理解论文
溯源树
样例
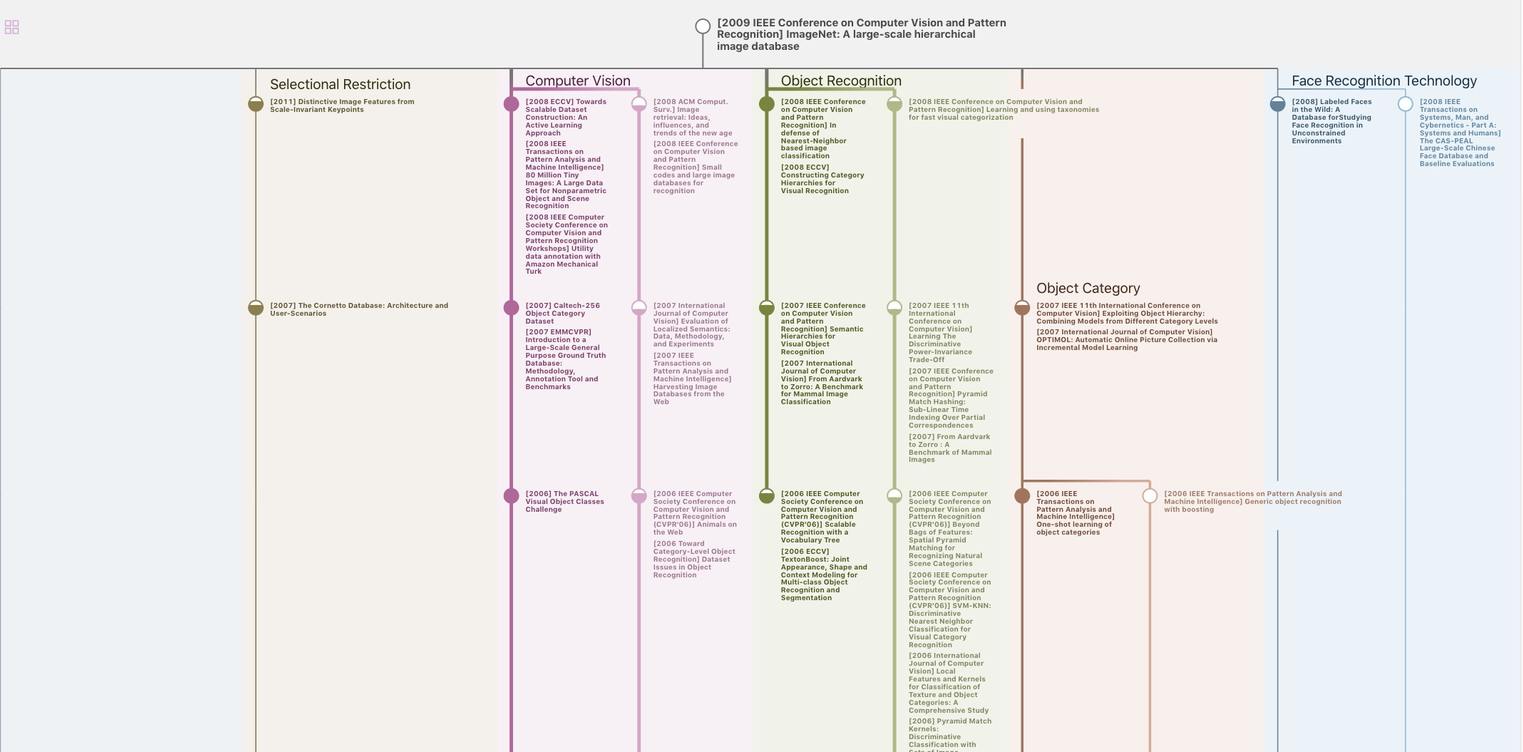
生成溯源树,研究论文发展脉络
Chat Paper
正在生成论文摘要