Automatic Estimation Of Self-Reported Pain By Interpretable Representations Of Motion Dynamics
2020 25TH INTERNATIONAL CONFERENCE ON PATTERN RECOGNITION (ICPR)(2020)
摘要
We propose an automatic method for pain intensity measurement from video. For each video, pain intensity was measured using the dynamics of facial movement using 66 facial points. Gram matrices formulation was used for facial points trajectory representations on the Riemannian manifold of symmetric positive semi-definite matrices of fixed rank. Curve fitting and temporal alignment were then used to smooth the extracted trajectories. A Support Vector Regression model was then trained to encode the extracted trajectories into ten pain intensity levels consistent with the Visual Analogue Scale for pain intensity measurement. The proposed approach was evaluated using the UNBC McMaster Shoulder Pain Archive and was compared to the state-of-the-art on the same data. Using both 5-fold cross-validation and leave-one-subject-out cross-validation, our results are competitive with respect to state-of-the-art methods.
更多查看译文
关键词
automatic estimation,self-reported pain,interpretable representations,motion dynamics,automatic method,pain intensity measurement,facial movement,66 facial points,gram matrices formulation,facial points trajectory representations,symmetric positive semidefinite matrices,temporal alignment,extracted trajectories,Support Vector Regression model,pain intensity levels,UNBC McMaster Shoulder Pain Archive
AI 理解论文
溯源树
样例
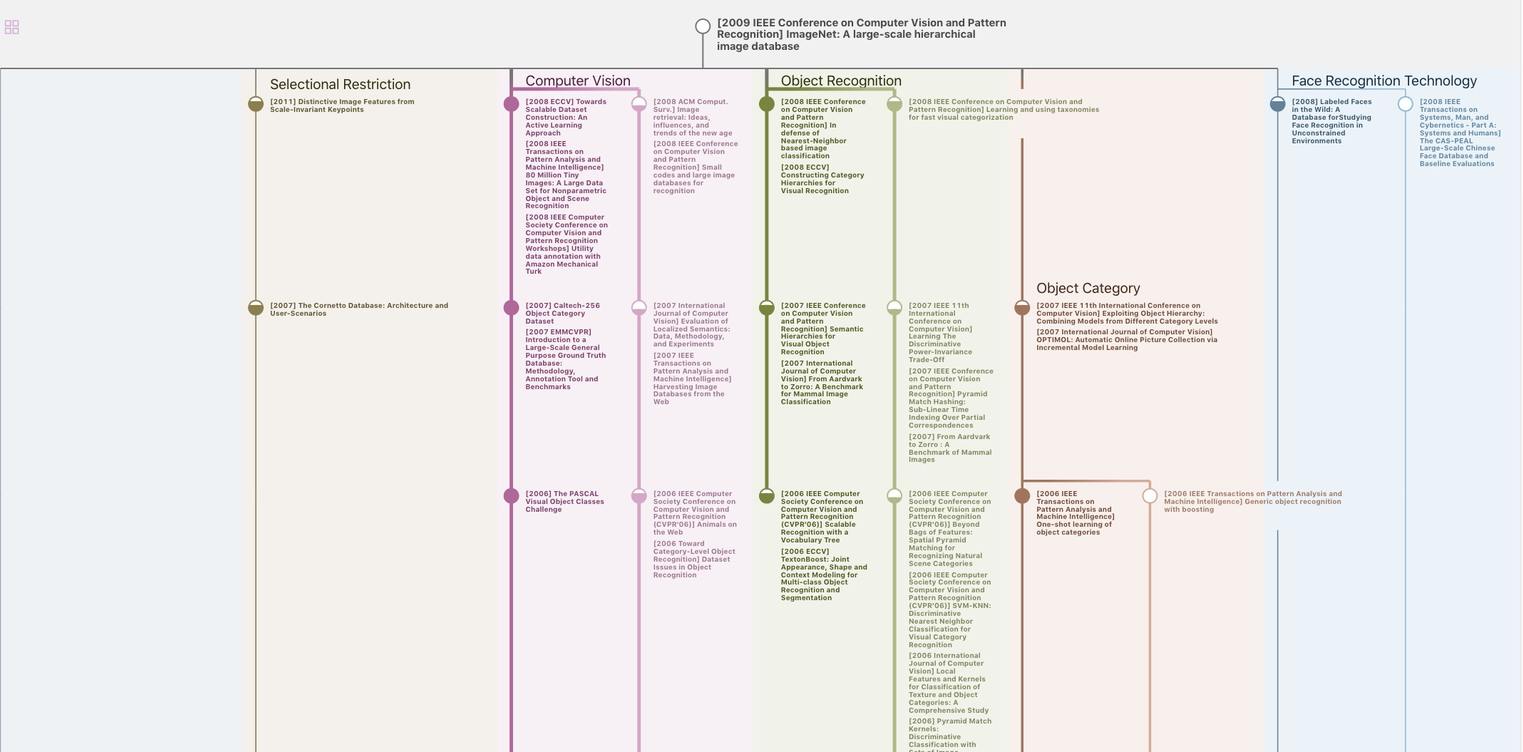
生成溯源树,研究论文发展脉络
Chat Paper
正在生成论文摘要